
BOOKS - Distributional Reinforcement Learning (Adaptive Computation and Machine Learn...

Distributional Reinforcement Learning (Adaptive Computation and Machine Learning)
Author: Marc G. Bellemare
Year: May 30, 2023
Format: PDF
File size: PDF 12 MB
Language: English
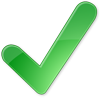
Year: May 30, 2023
Format: PDF
File size: PDF 12 MB
Language: English
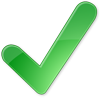
Distributional Reinforcement Learning Adaptive Computation and Machine Learning Distributional Reinforcement Learning Adaptive Computation and Machine Learning is a groundbreaking book that offers a fresh perspective on how intelligent agents make decisions in uncertain environments. The book provides a comprehensive guide to distributional reinforcement learning, a new mathematical formalism that focuses on the total reward or return obtained as a consequence of an agent's choices, specifically how this return behaves from a probabilistic perspective. The authors, who spearheaded the development of the field, present the key concepts and demonstrate its power to account for many complex phenomena that arise from interactions with one's environment. The book begins by providing a classical overview of reinforcement learning to contextualize distributional topics, followed by mathematical proofs pertaining to major results discussed in the text. The authors then guide the reader through a series of algorithmic and mathematical developments that characterize compute estimates and decision-making based on random returns. This comprehensive guide covers a wide range of applications, including finance, risk management, computational neuroscience, psychiatry, psychology, macroeconomics, and robotics, showcasing the expanding applications of distributional reinforcement learning in these fields. At its core, distributional reinforcement learning represents a new paradigm for understanding how intelligent agents make predictions and decisions in uncertain environments.
Распределенное обучение с подкреплением Адаптивные вычисления и машинное обучение Распределенное обучение с подкреплением Адаптивные вычисления и машинное обучение - это новаторская книга, которая предлагает новый взгляд на то, как интеллектуальные агенты принимают решения в неопределенной среде. Книга предоставляет всеобъемлющее руководство по обучению распределительному подкреплению, новый математический формализм, который фокусируется на общем вознаграждении или отдаче, полученной как следствие выбора агента, в частности, как эта отдача ведет себя с вероятностной точки зрения. Авторы, которые возглавили разработку месторождения, представляют ключевые концепции и демонстрируют его способность учитывать многие сложные явления, возникающие в результате взаимодействия с окружающей средой. Книга начинается с предоставления классического обзора обучения подкреплению для контекстуализации тем распределения, за которым следуют математические доказательства, относящиеся к основным результатам, обсуждаемым в тексте. Затем авторы направляют читателя через серию алгоритмических и математических разработок, которые характеризуют вычислительные оценки и принятие решений на основе случайных результатов. Это всеобъемлющее руководство охватывает широкий спектр приложений, включая финансы, управление рисками, вычислительную нейробиологию, психиатрию, психологию, макроэкономику и робототехнику, демонстрируя расширяющиеся приложения распределенного обучения в этих областях. По своей сути обучение распределенному подкреплению представляет собой новую парадигму для понимания того, как интеллектуальные агенты делают прогнозы и принимают решения в неопределенной среде.
Apprentissage distribué avec renfort Calcul adaptatif et apprentissage automatique Apprentissage distribué avec renforcement calcul adaptatif et l'apprentissage automatique sont des livres novateurs qui offrent un nouveau regard sur la façon dont les agents intellectuels prennent des décisions dans un environnement incertain. livre fournit un guide complet pour l'apprentissage des renforts de distribution, un nouveau formalisme mathématique qui se concentre sur la rémunération globale ou le rendement résultant du choix de l'agent, en particulier comment ce rendement se comporte d'un point de vue probabiliste. s auteurs qui ont dirigé le développement du gisement présentent des concepts clés et démontrent sa capacité à prendre en compte de nombreux phénomènes complexes résultant de l'interaction avec l'environnement. livre commence par fournir un aperçu classique de l'apprentissage du renforcement pour contextualiser les thèmes de la distribution, suivi de preuves mathématiques relatives aux principaux résultats discutés dans le texte. s auteurs dirigent ensuite le lecteur à travers une série de développements algorithmiques et mathématiques qui caractérisent les estimations de calcul et la prise de décision sur la base de résultats aléatoires. Ce guide complet couvre un large éventail d'applications, y compris la finance, la gestion des risques, les neurosciences informatiques, la psychiatrie, la psychologie, la macroéconomie et la robotique, démontrant l'expansion des applications de formation distribuée dans ces domaines. Par essence, l'apprentissage des renforts distribués constitue un nouveau paradigme pour comprendre comment les agents intellectuels font des prédictions et prennent des décisions dans un environnement incertain.
Aprendizaje distribuido con refuerzos Computación adaptativa y aprendizaje automático Aprendizaje distribuido con refuerzos Computación adaptativa y aprendizaje automático es un libro pionero que ofrece una nueva visión de cómo los agentes inteligentes toman decisiones en un entorno incierto. libro proporciona una guía integral para enseñar refuerzos distributivos, un nuevo formalismo matemático que se centra en la recompensa total o rendimientos obtenidos como consecuencia de la elección del agente, en particular, cómo este retorno se comporta desde un punto de vista probabilístico. autores que han liderado el desarrollo del yacimiento presentan conceptos clave y demuestran su capacidad para tener en cuenta muchos fenómenos complejos derivados de la interacción con el medio ambiente. libro comienza ofreciendo una revisión clásica del aprendizaje de refuerzos para contextualizar temas de distribución, seguida de evidencia matemática relacionada con los principales resultados discutidos en el texto. autores luego guían al lector a través de una serie de desarrollos algorítmicos y matemáticos que caracterizan las estimaciones computacionales y la toma de decisiones basadas en resultados aleatorios. Esta guía integral cubre una amplia gama de aplicaciones, incluyendo finanzas, gestión de riesgos, neurociencia computacional, psiquiatría, psicología, macroeconomía y robótica, mostrando aplicaciones de aprendizaje distribuido en expansión en estas áreas. Inherentemente, el entrenamiento en refuerzos distribuidos representa un nuevo paradigma para entender cómo los agentes intelectuales hacen predicciones y toman decisiones en un entorno incierto.
Apprendimento distribuito con supporto Elaborazione adattiva e apprendimento automatico Apprendimento distribuito con rinforzi Elaborazione adattiva e apprendimento automatico è un libro innovativo che offre una nuova visione del modo in cui gli agenti intelligenti decidono in un ambiente incerto. Il libro fornisce una guida completa per l'apprendimento dei rinforzi distributivi, un nuovo formalismo matematico che si concentra sulla ricompensa complessiva o sul rendimento ottenuto come conseguenza della scelta dell'agente, in particolare come questo rendimento si comporta da un punto di vista probabile. Gli autori che hanno guidato lo sviluppo del giacimento rappresentano i concetti chiave e dimostrano la sua capacità di tenere conto di molti fenomeni complessi derivanti dall'interazione con l'ambiente. Il libro inizia fornendo una panoramica classica dell'apprendimento dei rinforzi per contestualizzare i temi di distribuzione, seguita da prove matematiche relative ai principali risultati del testo. Gli autori guidano il lettore attraverso una serie di sviluppi algoritmici e matematici che caratterizzano le valutazioni computazionali e le decisioni basate su risultati casuali. Questa guida completa comprende una vasta gamma di applicazioni, tra cui finanza, gestione dei rischi, neuroscienze informatiche, psichiatria, psicologia, macroeconomia e robotica, dimostrando l'espansione delle applicazioni di apprendimento distribuito in questi settori. In pratica, l'apprendimento dei rinforzi distribuiti rappresenta un nuovo paradigma per capire come gli agenti intelligenti fanno previsioni e decidono in un ambiente incerto.
Verteiltes rnen mit Verstärkung Adaptives Rechnen und maschinelles rnen Verteiltes rnen mit Verstärkung Adaptives Rechnen und maschinelles rnen ist ein bahnbrechendes Buch, das einen neuen Blick darauf wirft, wie intelligente Agenten in einem unsicheren Umfeld Entscheidungen treffen. Das Buch bietet eine umfassende Anleitung zum rnen von Verteilungsverstärkung, einem neuen mathematischen Formalismus, der sich auf die Gesamtvergütung oder die Rendite konzentriert, die als Folge der Wahl des Agenten erzielt wird, insbesondere wie sich diese Rendite aus probabilistischer cht verhält. Die Autoren, die die Entwicklung des Feldes geleitet haben, präsentieren Schlüsselkonzepte und demonstrieren seine Fähigkeit, viele der komplexen Phänomene zu berücksichtigen, die sich aus der Interaktion mit der Umwelt ergeben. Das Buch beginnt mit einem klassischen Überblick über das Verstärkungstraining, um Verteilungsthemen zu kontextualisieren, gefolgt von mathematischen Beweisen, die sich auf die im Text diskutierten Hauptergebnisse beziehen. Die Autoren führen den ser dann durch eine Reihe von algorithmischen und mathematischen Entwicklungen, die rechnerische Auswertungen und Entscheidungen auf der Grundlage zufälliger Ergebnisse charakterisieren. Dieser umfassende itfaden deckt eine breite Palette von Anwendungen ab, darunter Finanzen, Risikomanagement, Computational Neuroscience, Psychiatrie, Psychologie, Makroökonomie und Robotik, und zeigt die wachsenden Anwendungen des verteilten rnens in diesen Bereichen. Im Kern stellt das verteilte Verstärkungstraining ein neues Paradigma dar, um zu verstehen, wie intelligente Agenten Vorhersagen treffen und Entscheidungen in einem unsicheren Umfeld treffen.
''
Dağıtılmış Takviye Öğrenme Uyarlamalı Hesaplama ve Makine Öğrenimi Dağıtılmış Takviye Öğrenme Uyarlamalı Hesaplama ve Makine Öğrenimi, akıllı ajanların belirsiz ortamlarda nasıl karar verdiğine dair yeni bir bakış açısı sunan çığır açan bir kitaptır. Kitap, dağıtımsal pekiştirmeyi öğretmek için kapsamlı bir rehber, ajan seçiminin bir sonucu olarak alınan toplam ödül veya geri dönüşe odaklanan yeni bir matematiksel biçimcilik, özellikle de bu dönüşün olasılıksal bir perspektiften nasıl davrandığını göstermektedir. Alanın gelişimine öncülük eden yazarlar, temel kavramları sunar ve çevre ile etkileşimlerden kaynaklanan birçok karmaşık fenomeni hesaba katma yeteneğini gösterir. Kitap, dağıtımın temalarını bağlamsallaştırmak için takviye eğitimine klasik bir genel bakış sunarak başlar, ardından metinde tartışılan ana sonuçlarla ilgili matematiksel kanıtlar gelir. Yazarlar daha sonra okuyucuyu, rastgele sonuçlara dayanan hesaplama değerlendirmelerini ve karar vermeyi karakterize eden bir dizi algoritmik ve matematiksel gelişme boyunca yönlendirir. Bu kapsamlı kılavuz, finans, risk yönetimi, hesaplamalı sinirbilim, psikiyatri, psikoloji, makroekonomi ve robotik gibi çok çeşitli uygulamaları kapsamakta ve bu alanlarda dağıtılmış öğrenmenin genişleyen uygulamalarını göstermektedir. Özünde, dağıtılmış takviye eğitimi, akıllı ajanların nasıl tahminler yaptığını ve belirsiz ortamlarda karar verdiğini anlamak için yeni bir paradigmayı temsil eder.
التعلم المعزز الموزع الحوسبة التكيفية والتعلم الآلي المعزز الموزع الحوسبة التكيفية والتعلم الآلي هو كتاب رائد يقدم منظورًا جديدًا حول كيفية اتخاذ الوكلاء الأذكياء للقرارات في بيئات غير مؤكدة. يقدم الكتاب دليلًا شاملاً لتعليم تعزيز التوزيع، وهو شكل رياضي جديد يركز على المكافأة الإجمالية أو العائد الذي يتم تلقيه نتيجة لاختيار الوكيل، وتحديداً كيف تتصرف هذه العودة من منظور احتمالي. يقدم المؤلفون الذين قادوا تطوير المجال مفاهيم رئيسية ويظهرون قدرته على حساب العديد من الظواهر المعقدة الناشئة عن التفاعلات مع البيئة. يبدأ الكتاب بتقديم لمحة عامة كلاسيكية عن تدريب التعزيز لوضع مواضيع التوزيع في سياقها، تليها البراهين الرياضية المتعلقة بالنتائج الرئيسية التي تمت مناقشتها في النص. ثم يوجه المؤلفون القارئ من خلال سلسلة من التطورات الخوارزمية والرياضية التي تميز التقييمات الحسابية وصنع القرار بناءً على النتائج العشوائية. يغطي هذا الدليل الشامل مجموعة واسعة من التطبيقات بما في ذلك التمويل وإدارة المخاطر وعلم الأعصاب الحسابي والطب النفسي وعلم النفس والاقتصاد الكلي والروبوتات، مما يوضح التطبيقات الموسعة للتعلم الموزع في هذه المجالات. يمثل تدريب التعزيز الموزع في جوهره نموذجًا جديدًا لفهم كيفية قيام الوكلاء الأذكياء بالتنبؤات واتخاذ القرارات في بيئات غير مؤكدة.
