
BOOKS - Deep Reinforcement Learning with Python, 2E

Deep Reinforcement Learning with Python, 2E
Author: Nimish Sanghi
Format: PDF
File size: PDF 18 MB
Language: English
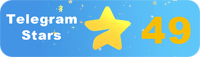
Format: PDF
File size: PDF 18 MB
Language: English
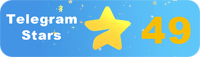
This new edition focuses on the latest advances in deep RL, covering everything from games and robotics to finance, and providing readers with a theoretical understanding of this rapidly evolving field. With a learn-by-coding approach, readers will be able to assimilate and replicate the latest research in deep RL, and explore its many applications.
The book begins with an introduction to reinforcement learning and its importance in modern technology, highlighting the need to study and understand the process of technological evolution as the basis for human survival and unity in a warring world. It emphasizes the importance of developing a personal paradigm for perceiving the technological process of developing modern knowledge, and how this can lead to the survival of humanity and the unification of people.
The first chapter covers the fundamentals of reinforcement learning, including the Markov decision process (MDP) and dynamic programming, providing a solid foundation for the rest of the book. The following chapters delve into more advanced topics such as multi-agent reinforcement learning, where multiple agents compete, and proximal policy optimization (PPO), one of the most widely used deep RL algorithms.
The book also explores the use of reinforcement learning with human feedback (RLHF) in chatbots built using large language models like ChatGPT, demonstrating how conversational capabilities can be improved through the integration of RL and NLP.
The book begins with an introduction to reinforcement learning and its importance in modern technology, highlighting the need to study and understand the process of technological evolution as the basis for human survival and unity in a warring world. It emphasizes the importance of developing a personal paradigm for perceiving the technological process of developing modern knowledge, and how this can lead to the survival of humanity and the unification of people.
The first chapter covers the fundamentals of reinforcement learning, including the Markov decision process (MDP) and dynamic programming, providing a solid foundation for the rest of the book. The following chapters delve into more advanced topics such as multi-agent reinforcement learning, where multiple agents compete, and proximal policy optimization (PPO), one of the most widely used deep RL algorithms.
The book also explores the use of reinforcement learning with human feedback (RLHF) in chatbots built using large language models like ChatGPT, demonstrating how conversational capabilities can be improved through the integration of RL and NLP.
Это новое издание посвящено последним достижениям в глубинной RL, охватывая все, от игр и робототехники до финансов, и предоставляя читателям теоретическое понимание этой быстро развивающейся области. Благодаря подходу «обучение по коду» читатели смогут ассимилировать и воспроизвести последние исследования в области глубокого RL и исследовать его многочисленные применения.
Книга начинается с введения в обучение с подкреплением и его важность в современных технологиях, подчеркивая необходимость изучения и понимания процесса технологической эволюции как основы выживания и единства человека в воюющем мире. В нем подчеркивается важность выработки личностной парадигмы восприятия технологического процесса развития современных знаний, и того, как это может привести к выживанию человечества и объединению людей.
B первой главе рассматриваются основы обучения с подкреплением, включая марковский процесс принятия решений (MDP) и динамическое программирование, что обеспечивает прочную основу для остальной части книги. Следующие главы углубляются в более продвинутые темы, такие как многоагентное обучение с подкреплением, где конкурируют несколько агентов, и проксимальная оптимизация политики (PPO), один из наиболее широко используемых алгоритмов глубокого RL.
В книге также рассматривается использование обучения с подкреплением с обратной связью от человека (RLHF) в чат-ботах, построенных с использованием больших языковых моделей, таких как ChatGPT, демонстрируя, как можно улучшить разговорные возможности за счет интеграции RL и NLP.
Книга начинается с введения в обучение с подкреплением и его важность в современных технологиях, подчеркивая необходимость изучения и понимания процесса технологической эволюции как основы выживания и единства человека в воюющем мире. В нем подчеркивается важность выработки личностной парадигмы восприятия технологического процесса развития современных знаний, и того, как это может привести к выживанию человечества и объединению людей.
B первой главе рассматриваются основы обучения с подкреплением, включая марковский процесс принятия решений (MDP) и динамическое программирование, что обеспечивает прочную основу для остальной части книги. Следующие главы углубляются в более продвинутые темы, такие как многоагентное обучение с подкреплением, где конкурируют несколько агентов, и проксимальная оптимизация политики (PPO), один из наиболее широко используемых алгоритмов глубокого RL.
В книге также рассматривается использование обучения с подкреплением с обратной связью от человека (RLHF) в чат-ботах, построенных с использованием больших языковых моделей, таких как ChatGPT, демонстрируя, как можно улучшить разговорные возможности за счет интеграции RL и NLP.
Cette nouvelle édition est consacrée aux dernières avancées de la RL profonde, couvrant tout, des jeux et de la robotique à la finance, et fournissant aux lecteurs une compréhension théorique de ce domaine en évolution rapide. Grâce à l'approche « code learning », les lecteurs seront en mesure d'assimiler et de reproduire les dernières recherches dans le domaine de la RL profonde et d'explorer ses nombreuses applications.
livre commence par une introduction à l'apprentissage avec des renforts et son importance dans les technologies modernes, soulignant la nécessité d'étudier et de comprendre le processus d'évolution technologique comme base de la survie et de l'unité de l'homme dans un monde en guerre. Il souligne l'importance d'élaborer un paradigme personnel pour la perception du processus technologique du développement des connaissances modernes, et comment cela peut conduire à la survie de l'humanité et à l'unification des hommes.
premier chapitre traite des bases de l'apprentissage avec des renforts, y compris le processus décisionnel de Markov (MDP) et la programmation dynamique, ce qui fournit une base solide pour le reste du livre. s chapitres suivants examinent des sujets plus avancés, tels que l'apprentissage multi-agents avec des renforts, où plusieurs agents sont en concurrence, et l'optimisation proximale des politiques (PPO), l'un des algorithmes de RL profonde les plus utilisés.
livre examine également l'utilisation de l'apprentissage par rétroaction humaine (RLHF) dans les chatbots construits à l'aide de grands modèles linguistiques tels que ChatGPT, montrant comment améliorer les capacités de conversation en intégrant RL et NLP.
livre commence par une introduction à l'apprentissage avec des renforts et son importance dans les technologies modernes, soulignant la nécessité d'étudier et de comprendre le processus d'évolution technologique comme base de la survie et de l'unité de l'homme dans un monde en guerre. Il souligne l'importance d'élaborer un paradigme personnel pour la perception du processus technologique du développement des connaissances modernes, et comment cela peut conduire à la survie de l'humanité et à l'unification des hommes.
premier chapitre traite des bases de l'apprentissage avec des renforts, y compris le processus décisionnel de Markov (MDP) et la programmation dynamique, ce qui fournit une base solide pour le reste du livre. s chapitres suivants examinent des sujets plus avancés, tels que l'apprentissage multi-agents avec des renforts, où plusieurs agents sont en concurrence, et l'optimisation proximale des politiques (PPO), l'un des algorithmes de RL profonde les plus utilisés.
livre examine également l'utilisation de l'apprentissage par rétroaction humaine (RLHF) dans les chatbots construits à l'aide de grands modèles linguistiques tels que ChatGPT, montrant comment améliorer les capacités de conversation en intégrant RL et NLP.
Esta nueva edición trata sobre los últimos avances en el RL profundo, abarcando todo, desde juegos y robótica hasta finanzas, y proporcionando a los lectores una comprensión teórica de este campo en rápida evolución. Gracias al enfoque de «aprendizaje en código», los lectores podrán asimilar y reproducir las últimas investigaciones en el campo de la RL profunda e investigar sus múltiples aplicaciones.
libro comienza con una introducción al aprendizaje con refuerzos y su importancia en la tecnología moderna, destacando la necesidad de estudiar y comprender el proceso de evolución tecnológica como base de la supervivencia y unidad del hombre en un mundo en guerra. Destaca la importancia de generar un paradigma personal para percibir el proceso tecnológico del desarrollo del conocimiento moderno, y cómo esto puede conducir a la supervivencia de la humanidad y a la unión de los seres humanos.
primer capítulo aborda los fundamentos del aprendizaje con refuerzos, incluyendo el proceso de toma de decisiones de Markov (MDP) y la programación dinámica, lo que proporciona una base sólida para el resto del libro. siguientes capítulos profundizan en temas más avanzados, como el aprendizaje multiagente con refuerzos, donde compiten varios agentes, y la optimización de políticas proximales (PPO), uno de los algoritmos de RL profundo más utilizados.
libro también aborda el uso del aprendizaje con refuerzo de retroalimentación humana (RLHF) en los chatbots construidos con grandes modelos de lenguaje como ChatGPT, demostrando cómo se pueden mejorar las capacidades de conversación mediante la integración de RL y NLP.
libro comienza con una introducción al aprendizaje con refuerzos y su importancia en la tecnología moderna, destacando la necesidad de estudiar y comprender el proceso de evolución tecnológica como base de la supervivencia y unidad del hombre en un mundo en guerra. Destaca la importancia de generar un paradigma personal para percibir el proceso tecnológico del desarrollo del conocimiento moderno, y cómo esto puede conducir a la supervivencia de la humanidad y a la unión de los seres humanos.
primer capítulo aborda los fundamentos del aprendizaje con refuerzos, incluyendo el proceso de toma de decisiones de Markov (MDP) y la programación dinámica, lo que proporciona una base sólida para el resto del libro. siguientes capítulos profundizan en temas más avanzados, como el aprendizaje multiagente con refuerzos, donde compiten varios agentes, y la optimización de políticas proximales (PPO), uno de los algoritmos de RL profundo más utilizados.
libro también aborda el uso del aprendizaje con refuerzo de retroalimentación humana (RLHF) en los chatbots construidos con grandes modelos de lenguaje como ChatGPT, demostrando cómo se pueden mejorar las capacidades de conversación mediante la integración de RL y NLP.
Esta nova edição trata dos avanços mais recentes na RL profunda, abrangendo tudo, desde jogos e robótica até finanças, e fornecendo aos leitores uma compreensão teórica desta área em rápida evolução. Com a abordagem «treinamento de código», os leitores poderão assimilar e reproduzir as últimas pesquisas sobre RL profundo e explorar suas múltiplas aplicações.
O livro começa com a introdução ao aprendizado com reforços e sua importância nas tecnologias modernas, enfatizando a necessidade de explorar e compreender o processo de evolução tecnológica como base para a sobrevivência e unidade do homem no mundo em guerra. Ele enfatiza a importância de criar um paradigma pessoal para a percepção do processo tecnológico de desenvolvimento do conhecimento moderno, e como isso pode levar à sobrevivência da humanidade e à união das pessoas.
O primeiro capítulo aborda os fundamentos do treinamento com reforços, incluindo o processo decisório de Marcova (MDP) e a programação dinâmica, o que oferece uma base sólida para o resto do livro. Os capítulos seguintes se aprofundam em temas mais avançados, como treinamento multifacetado com reforços, onde vários agentes competem, e otimização proximal de políticas (PPO), um dos algoritmos mais utilizados do RL profundo.
O livro também aborda o uso de treinamento com reforços com feedback humano (RLHF) em bate-bots construídos com modelos linguísticos maiores, como ChatGPT, mostrando como é possível melhorar a capacidade de comunicação através da integração de RL e NLP.
O livro começa com a introdução ao aprendizado com reforços e sua importância nas tecnologias modernas, enfatizando a necessidade de explorar e compreender o processo de evolução tecnológica como base para a sobrevivência e unidade do homem no mundo em guerra. Ele enfatiza a importância de criar um paradigma pessoal para a percepção do processo tecnológico de desenvolvimento do conhecimento moderno, e como isso pode levar à sobrevivência da humanidade e à união das pessoas.
O primeiro capítulo aborda os fundamentos do treinamento com reforços, incluindo o processo decisório de Marcova (MDP) e a programação dinâmica, o que oferece uma base sólida para o resto do livro. Os capítulos seguintes se aprofundam em temas mais avançados, como treinamento multifacetado com reforços, onde vários agentes competem, e otimização proximal de políticas (PPO), um dos algoritmos mais utilizados do RL profundo.
O livro também aborda o uso de treinamento com reforços com feedback humano (RLHF) em bate-bots construídos com modelos linguísticos maiores, como ChatGPT, mostrando como é possível melhorar a capacidade de comunicação através da integração de RL e NLP.
Questa nuova edizione è dedicata agli ultimi progressi nella RL profonda, coprendo tutto, dai giochi alla robotica alla finanza, e fornendo ai lettori una comprensione teorica di questo campo in rapida evoluzione. Grazie all'approccio «apprendimento del codice», i lettori possono assimilare e riprodurre le ricerche più recenti in materia di RL profonda e esplorare le sue numerose applicazioni.
Il libro inizia con l'introduzione all'apprendimento con rinforzi e la sua importanza nelle tecnologie moderne, sottolineando la necessità di studiare e comprendere l'evoluzione tecnologica come base per la sopravvivenza e l'unità umana in un mondo in guerra. Sottolinea l'importanza di sviluppare un paradigma personale per la percezione del processo tecnologico dello sviluppo delle conoscenze moderne, e come ciò possa portare alla sopravvivenza dell'umanità e all'unione delle persone.
B il primo capitolo affronta le basi dell'apprendimento con rinforzi, inclusi il processo decisionale di Markov (MDP) e la programmazione dinamica, fornendo una base solida per il resto del libro. I seguenti capitoli vengono approfonditi su temi più avanzati, come l'apprendimento multiagente con rinforzi in cui concorrono più agenti e l'ottimizzazione proximica delle politiche (PPO), uno degli algoritmi più utilizzati dalla RL profonda.
Il libro descrive anche l'utilizzo di un apprendimento con feedback umano (RLHF) in chat-bot costruiti utilizzando modelli linguistici di grandi dimensioni, come ad esempio il ChatGPT, dimostrando come è possibile migliorare le capacità di conversazione attraverso l'integrazione di RL e NLP.
Il libro inizia con l'introduzione all'apprendimento con rinforzi e la sua importanza nelle tecnologie moderne, sottolineando la necessità di studiare e comprendere l'evoluzione tecnologica come base per la sopravvivenza e l'unità umana in un mondo in guerra. Sottolinea l'importanza di sviluppare un paradigma personale per la percezione del processo tecnologico dello sviluppo delle conoscenze moderne, e come ciò possa portare alla sopravvivenza dell'umanità e all'unione delle persone.
B il primo capitolo affronta le basi dell'apprendimento con rinforzi, inclusi il processo decisionale di Markov (MDP) e la programmazione dinamica, fornendo una base solida per il resto del libro. I seguenti capitoli vengono approfonditi su temi più avanzati, come l'apprendimento multiagente con rinforzi in cui concorrono più agenti e l'ottimizzazione proximica delle politiche (PPO), uno degli algoritmi più utilizzati dalla RL profonda.
Il libro descrive anche l'utilizzo di un apprendimento con feedback umano (RLHF) in chat-bot costruiti utilizzando modelli linguistici di grandi dimensioni, come ad esempio il ChatGPT, dimostrando come è possibile migliorare le capacità di conversazione attraverso l'integrazione di RL e NLP.
Diese neue Ausgabe konzentriert sich auf die neuesten Fortschritte in der Deep RL und deckt alles von Spielen und Robotik bis hin zu Finanzen ab und bietet den sern einen theoretischen Einblick in dieses sich schnell entwickelnde Feld. Durch den „Code arning“ -Ansatz werden die ser in der Lage sein, die neuesten Forschungsergebnisse auf dem Gebiet der tiefen RL zu assimilieren und zu reproduzieren und ihre zahlreichen Anwendungen zu erforschen. Das Buch beginnt mit einer Einführung in das rnen mit Verstärkung und seiner Bedeutung in der modernen Technologie und betont die Notwendigkeit, den Prozess der technologischen Evolution als Grundlage für das Überleben und die Einheit des Menschen in einer kriegerischen Welt zu studieren und zu verstehen. Es betont, wie wichtig es ist, ein persönliches Paradigma für die Wahrnehmung des technologischen Prozesses der Entwicklung des modernen Wissens zu entwickeln und wie dies zum Überleben der Menschheit und zur Vereinigung der Menschen führen kann.
Im ersten Kapitel werden die Grundlagen des verstärkenden rnens behandelt, einschließlich des Markov-Entscheidungsprozesses (MDP) und der dynamischen Programmierung, die eine solide Grundlage für den Rest des Buches bilden. Die folgenden Kapitel vertiefen sich in fortgeschrittenere Themen wie Multi-Agent-Training mit Verstärkung, bei dem mehrere Agenten konkurrieren, und proximale Policy Optimization (PPO), einer der am häufigsten verwendeten Deep-RL-Algorithmen. Das Buch befasst sich auch mit der Verwendung von Human Feedback Enhancement Training (RLHF) in Chatbots, die mit großen Sprachmodellen wie ChatGPT erstellt wurden, und zeigt, wie die Konversationsfähigkeit durch die Integration von RL und NLP verbessert werden kann.
Im ersten Kapitel werden die Grundlagen des verstärkenden rnens behandelt, einschließlich des Markov-Entscheidungsprozesses (MDP) und der dynamischen Programmierung, die eine solide Grundlage für den Rest des Buches bilden. Die folgenden Kapitel vertiefen sich in fortgeschrittenere Themen wie Multi-Agent-Training mit Verstärkung, bei dem mehrere Agenten konkurrieren, und proximale Policy Optimization (PPO), einer der am häufigsten verwendeten Deep-RL-Algorithmen. Das Buch befasst sich auch mit der Verwendung von Human Feedback Enhancement Training (RLHF) in Chatbots, die mit großen Sprachmodellen wie ChatGPT erstellt wurden, und zeigt, wie die Konversationsfähigkeit durch die Integration von RL und NLP verbessert werden kann.
Ta nowa edycja koncentruje się na najnowszych osiągnięciach w głębokim RL, obejmujących wszystko, od gier i robotyki do finansowania, oraz zapewniając czytelnikom teoretyczne zrozumienie tej szybko rozwijającej się dziedziny. Dzięki podejściu do „uczenia się kodu” czytelnicy będą mogli asymilować i replikować najnowsze badania w głębokim RL i badać jego wiele zastosowań.
Książka rozpoczyna się wprowadzeniem do uczenia się wzmacniającego i jej znaczenia w nowoczesnej technologii, podkreślając potrzebę studiowania i zrozumienia procesu ewolucji technologicznej jako podstawy ludzkiego przetrwania i jedności w wojującym świecie. Podkreśla znaczenie rozwijania osobistego paradygmatu postrzegania technologicznego procesu rozwoju nowoczesnej wiedzy i tego, jak może to prowadzić do przetrwania ludzkości i zjednoczenia ludzi.
Pierwszy rozdział obejmuje podstawy uczenia się wzmacniającego, w tym Proces decyzyjny Markowa (MDP) i programowanie dynamiczne, które stanowi solidny fundament dla reszty książki. Poniższe rozdziały skupiają się na bardziej zaawansowanych tematach, takich jak wieloagentowe uczenie się wzmacniania, w którym konkuruje wielu agentów, oraz proksymalna optymalizacja polityki (PPO), jeden z najczęściej stosowanych algorytmów głębokiego RL.
Książka bada również wykorzystanie ludzkiego wzmacniania zwrotnego (RLHF) uczenia się w chatbotach zbudowanych przy użyciu dużych modeli językowych, takich jak ChatGPT, pokazując, w jaki sposób można poprawić zdolności konwersacyjne poprzez integrację RL i NLP.
Książka rozpoczyna się wprowadzeniem do uczenia się wzmacniającego i jej znaczenia w nowoczesnej technologii, podkreślając potrzebę studiowania i zrozumienia procesu ewolucji technologicznej jako podstawy ludzkiego przetrwania i jedności w wojującym świecie. Podkreśla znaczenie rozwijania osobistego paradygmatu postrzegania technologicznego procesu rozwoju nowoczesnej wiedzy i tego, jak może to prowadzić do przetrwania ludzkości i zjednoczenia ludzi.
Pierwszy rozdział obejmuje podstawy uczenia się wzmacniającego, w tym Proces decyzyjny Markowa (MDP) i programowanie dynamiczne, które stanowi solidny fundament dla reszty książki. Poniższe rozdziały skupiają się na bardziej zaawansowanych tematach, takich jak wieloagentowe uczenie się wzmacniania, w którym konkuruje wielu agentów, oraz proksymalna optymalizacja polityki (PPO), jeden z najczęściej stosowanych algorytmów głębokiego RL.
Książka bada również wykorzystanie ludzkiego wzmacniania zwrotnego (RLHF) uczenia się w chatbotach zbudowanych przy użyciu dużych modeli językowych, takich jak ChatGPT, pokazując, w jaki sposób można poprawić zdolności konwersacyjne poprzez integrację RL i NLP.
מהדורה חדשה זו מתמקדת בהתקדמות האחרונה ב-RL העמוק, סיקור הכל החל ממשחקים ורובוטיקה וכלה במימון, בעזרת גישת ”לימוד קוד”, הקוראים יוכלו להטמיע ולשכפל את המחקר העדכני ביותר ב-RL העמוק ולחקור את יישומיו הרבים.
הספר מתחיל במבוא לחיזוק הלמידה וחשיבותה בטכנולוגיה המודרנית, ומדגיש את הצורך ללמוד ולהבין את תהליך האבולוציה הטכנולוגית כבסיס להישרדות ולאחדות האנושית בעולם לוחם. הוא מדגיש את החשיבות של פיתוח פרדיגמה אישית לתפיסה של התהליך הטכנולוגי של התפתחות הידע המודרני, וכיצד זה יכול להוביל להישרדות האנושות ולאיחוד בני האדם.
הפרק הראשון מכסה את היסודות של למידת חיזוק, כולל תהליך החלטה מרקוב (MDP) ותכנות דינמי, אשר מספק בסיס מוצק לשאר הספר. הפרקים הבאים מתעמקים בנושאים מתקדמים יותר כמו למידת חיזוק רב-סוכנים, בהם סוכנים מרובים מתחרים, ואופטימיזציה של מדיניות פרוקסימלית (PPO), אחד האלגוריתמים העמוקים ביותר בשימוש.
הספר בוחן גם את השימוש בחיזוק משוב אנושי (RLHF) למידה בצ 'אט-בוטים הבנויים באמצעות מודלים גדולים של שפות כגון ChatGPT, המדגים כיצד יכולות שיחה יכולות להשתפר על ידי שילוב RL ו-NLP.''
הספר מתחיל במבוא לחיזוק הלמידה וחשיבותה בטכנולוגיה המודרנית, ומדגיש את הצורך ללמוד ולהבין את תהליך האבולוציה הטכנולוגית כבסיס להישרדות ולאחדות האנושית בעולם לוחם. הוא מדגיש את החשיבות של פיתוח פרדיגמה אישית לתפיסה של התהליך הטכנולוגי של התפתחות הידע המודרני, וכיצד זה יכול להוביל להישרדות האנושות ולאיחוד בני האדם.
הפרק הראשון מכסה את היסודות של למידת חיזוק, כולל תהליך החלטה מרקוב (MDP) ותכנות דינמי, אשר מספק בסיס מוצק לשאר הספר. הפרקים הבאים מתעמקים בנושאים מתקדמים יותר כמו למידת חיזוק רב-סוכנים, בהם סוכנים מרובים מתחרים, ואופטימיזציה של מדיניות פרוקסימלית (PPO), אחד האלגוריתמים העמוקים ביותר בשימוש.
הספר בוחן גם את השימוש בחיזוק משוב אנושי (RLHF) למידה בצ 'אט-בוטים הבנויים באמצעות מודלים גדולים של שפות כגון ChatGPT, המדגים כיצד יכולות שיחה יכולות להשתפר על ידי שילוב RL ו-NLP.''
Bu yeni baskı, oyun ve robotikten finansa kadar her şeyi kapsayan ve okuyuculara bu hızla gelişen alan hakkında teorik bir anlayış sağlayan derin RL'deki en son gelişmelere odaklanmaktadır. "Kod öğrenme" yaklaşımıyla, okuyucular derin RL'deki en son araştırmaları özümseyebilecek ve çoğaltabilecek ve birçok uygulamasını keşfedebileceklerdir. Kitap, takviye öğrenmeye ve modern teknolojideki önemine bir giriş ile başlar ve teknolojik evrim sürecini, savaşan bir dünyada insanın hayatta kalması ve birliği için temel olarak inceleme ve anlama ihtiyacını vurgular. Modern bilginin gelişiminin teknolojik sürecinin algılanması için kişisel bir paradigma geliştirmenin önemini ve bunun insanlığın hayatta kalmasına ve insanların birleşmesine nasıl yol açabileceğini vurgular. İlk bölüm, Markov Karar Süreci (MDP) ve kitabın geri kalanı için sağlam bir temel sağlayan dinamik programlama dahil olmak üzere pekiştirmeli öğrenmenin temellerini kapsar. Aşağıdaki bölümlerde, çoklu ajanların rekabet ettiği çok aracılı pekiştirmeli öğrenme ve en yaygın kullanılan derin RL algoritmalarından biri olan proksimal politika optimizasyonu (PPO) gibi daha ileri konulara değinilmektedir. Kitap ayrıca, ChatGPT gibi büyük dil modelleri kullanılarak oluşturulan chatbotlarda insan geri bildirim takviyesi (RLHF) öğreniminin kullanımını inceleyerek, RL ve NLP'yi entegre ederek konuşma yeteneklerinin nasıl geliştirilebileceğini göstermektedir.
يركز هذا الإصدار الجديد على أحدث التطورات في RL العميقة، حيث يغطي كل شيء من الألعاب والروبوتات إلى التمويل، ويزود القراء بفهم نظري لهذا المجال سريع التطور. من خلال نهج «تعلم الكود»، سيتمكن القراء من استيعاب وتكرار أحدث الأبحاث في RL العميق واستكشاف تطبيقاته العديدة. يبدأ الكتاب بمقدمة عن التعلم المعزز وأهميته في التكنولوجيا الحديثة، مع التأكيد على الحاجة إلى دراسة وفهم عملية التطور التكنولوجي كأساس لبقاء الإنسان ووحدته في عالم متحارب. ويؤكد على أهمية وضع نموذج شخصي لتصور العملية التكنولوجية لتطور المعرفة الحديثة، وكيف يمكن أن يؤدي ذلك إلى بقاء البشرية وتوحيد الشعوب. يغطي الفصل الأول أساسيات التعلم المعزز، بما في ذلك عملية قرار ماركوف (MDP) والبرمجة الديناميكية، والتي توفر أساسًا متينًا لبقية الكتاب. تتعمق الفصول التالية في موضوعات أكثر تقدمًا مثل التعلم المعزز متعدد الوكلاء، حيث يتنافس العديد من الوكلاء، وتحسين السياسة القريبة (PPO)، وهي واحدة من خوارزميات RL العميقة الأكثر استخدامًا. يبحث الكتاب أيضًا في استخدام تعزيز التعليقات البشرية (RLHF) في روبوتات الدردشة المبنية باستخدام نماذج لغوية كبيرة مثل ChatGPT، مما يوضح كيف يمكن تحسين قدرات المحادثة من خلال دمج RL و NLP.
이 새 버전은 게임 및 로봇 공학에서 금융에 이르기까지 모든 것을 다루고 독자들에게 빠르게 진화하는 분야에 대한 이론적 이해를 제공하는 깊은 RL의 최신 발전에 중점을 둡니다. "코드 학습" 접근 방식을 통해 독자는 심층 RL의 최신 연구를 동화하고 복제하고 많은 응용 프로그램을 탐색 할 수 있습니다.
이 책은 강화 학습에 대한 소개와 현대 기술에서의 중요성으로 시작하여 전쟁 세계에서 인간 생존과 연합의 기초로서 기술 진화 과정을 연구하고 이해할 필요성을 강조합니다. 현대 지식 개발의 기술 과정에 대한 인식을위한 개인 패러다임 개발의 중요성과 이것이 어떻게 인류의 생존과 사람들의 통일로 이어질 수 있는지를 강조합니다.
첫 번째 장은 Markov Decision Process (MDP) 및 동적 프로그래밍을 포함하여 강화 학습의 기본 사항을 다루며 나머지 책에 대한 확실한 토대를 제공합니다. 다음 장은 다중 에이전트가 경쟁하는 다중 에이전트 강화 학습 및 가장 널리 사용되는 딥 RL 알고리즘 중 하나 인 근위 정책 최적화 (PPO) 와 같은 고급 주제를 설명합니다.
이 책은 또한 ChatGPT와 같은 대형 언어 모델을 사용하여 구축 된 핫봇에서 인간 피드백 강화 (RLHF) 학습의 사용을 검토하여 RL과 NLP를 통합하여 대화 기능을 개선 할 수있는 방법을 보여줍니다.
이 책은 강화 학습에 대한 소개와 현대 기술에서의 중요성으로 시작하여 전쟁 세계에서 인간 생존과 연합의 기초로서 기술 진화 과정을 연구하고 이해할 필요성을 강조합니다. 현대 지식 개발의 기술 과정에 대한 인식을위한 개인 패러다임 개발의 중요성과 이것이 어떻게 인류의 생존과 사람들의 통일로 이어질 수 있는지를 강조합니다.
첫 번째 장은 Markov Decision Process (MDP) 및 동적 프로그래밍을 포함하여 강화 학습의 기본 사항을 다루며 나머지 책에 대한 확실한 토대를 제공합니다. 다음 장은 다중 에이전트가 경쟁하는 다중 에이전트 강화 학습 및 가장 널리 사용되는 딥 RL 알고리즘 중 하나 인 근위 정책 최적화 (PPO) 와 같은 고급 주제를 설명합니다.
이 책은 또한 ChatGPT와 같은 대형 언어 모델을 사용하여 구축 된 핫봇에서 인간 피드백 강화 (RLHF) 학습의 사용을 검토하여 RL과 NLP를 통합하여 대화 기능을 개선 할 수있는 방법을 보여줍니다.
この新版は、深層RLの最新の進歩に焦点を当て、ゲームやロボティクスから金融までを網羅し、この急速に進化する分野について理論的な理解を読者に提供します。「コード学習」アプローチを使用すると、読者は深いRLの最新の研究を同化して複製し、その多くのアプリケーションを探索することができます。
本書は、現代の技術における強化学習とその重要性の紹介から始まり、戦争世界における人間の生存と統一の基礎としての技術進化の過程を研究し理解する必要性を強調した。それは、現代の知識の発展の技術的プロセスの認識のための個人的なパラダイムを開発することの重要性を強調し、これが人類の生存と人々の統一につながる方法。
第1章では、マルコフ意思決定プロセス(MDP)や動的プログラミングなどの強化学習の基礎について説明します。次の章では、複数のエージェントが競合するマルチエージェント強化学習や、最も広く使用されている深層RLアルゴリズムの1つである近接政策最適化(PPO)など、より高度なトピックについて詳しく説明します。
また、ChatGPTなどの大きな言語モデルを用いて構築されたチャットボットでの人間のフィードバック強化(RLHF)学習の使用を検討し、RLとNLPを統合することで会話能力をどのように向上させることができるかを実証している。
本書は、現代の技術における強化学習とその重要性の紹介から始まり、戦争世界における人間の生存と統一の基礎としての技術進化の過程を研究し理解する必要性を強調した。それは、現代の知識の発展の技術的プロセスの認識のための個人的なパラダイムを開発することの重要性を強調し、これが人類の生存と人々の統一につながる方法。
第1章では、マルコフ意思決定プロセス(MDP)や動的プログラミングなどの強化学習の基礎について説明します。次の章では、複数のエージェントが競合するマルチエージェント強化学習や、最も広く使用されている深層RLアルゴリズムの1つである近接政策最適化(PPO)など、より高度なトピックについて詳しく説明します。
また、ChatGPTなどの大きな言語モデルを用いて構築されたチャットボットでの人間のフィードバック強化(RLHF)学習の使用を検討し、RLとNLPを統合することで会話能力をどのように向上させることができるかを実証している。
此新版本致力於深度RL的最新進展,涵蓋了從遊戲和機器人技術到金融的所有內容,並為讀者提供了對該快速發展的領域的理論見解。借助「代碼學習」方法,讀者將能夠吸收和復制深度RL領域的最新研究,並研究其眾多應用。本書首先介紹強化學習及其在現代技術中的重要性,強調需要研究和理解技術進化的過程,作為人類在交戰世界中生存和團結的基礎。它強調了建立個人範式以感知現代知識發展的過程過程的重要性,以及這如何導致人類的生存和人類團結。第一章B論述了強化學習的基礎,包括馬爾可夫決策過程(MDP)和動態編程,為本書的其余部分提供了堅實的基礎。以下章節將深入研究更高級的主題,例如多代理強化學習(多代理競爭)和近端策略優化(PPO)(最廣泛使用的深度RL算法之一)。
該書還探討了使用大型語言模型(例如ChatGPT)構建的聊天機器人中使用人類反饋強化學習(RLHF)的方法,展示了如何通過集成RL和NLP來改善對話機會。
該書還探討了使用大型語言模型(例如ChatGPT)構建的聊天機器人中使用人類反饋強化學習(RLHF)的方法,展示了如何通過集成RL和NLP來改善對話機會。
