
BOOKS - Probabilistic Machine Learning: An Introduction (Adaptive Computation and Mac...

Probabilistic Machine Learning: An Introduction (Adaptive Computation and Machine Learning series)
Author: Kevin P. Murphy
Year: March 1, 2022
Format: PDF
File size: PDF 48 MB
Language: English
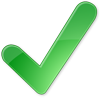
Year: March 1, 2022
Format: PDF
File size: PDF 48 MB
Language: English
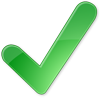
Book: Probabilistic Machine Learning An Introduction Adaptive Computation and Machine Learning series As technology continues to evolve at an unprecedented rate, it is crucial for us to understand the process of technological development and its impact on modern knowledge. Probabilistic Machine Learning: An Introduction, part of the Adaptive Computation and Machine Learning series, offers a comprehensive and up-to-date introduction to machine learning through the lens of probabilistic modeling and Bayesian decision theory. This book provides a detailed overview of mathematical background, including linear algebra and optimization, basic supervised learning, and advanced topics like transfer learning and unsupervised learning. The author, who previously wrote Machine Learning: A Probabilistic Perspective, has thoroughly updated the content to reflect the significant advancements in the field since 2012, particularly deep learning. The new book includes online Python code using libraries such as scikit-learn, JAX, PyTorch, and TensorFlow, allowing students to apply their knowledge and reproduce figures. This practical component complements the theoretical topics discussed in the book, making it an essential resource for anyone looking to gain a deeper understanding of machine learning. The book begins by introducing the fundamental concepts of probability theory and Bayesian decision theory, providing a solid foundation for the subsequent chapters. It covers linear regression, logistic regression, and deep neural networks, with each chapter building upon the previous one to create a cohesive and comprehensive introduction to machine learning.
Book: Probabilistic Machine arning An Introduction Adaptive Computation and Machine arning series Поскольку технологии продолжают развиваться беспрецедентными темпами, для нас крайне важно понимать процесс технологического развития и его влияние на современные знания. Probabilistic Machine arning: An Introduction, часть серии Adaptive Computation and Machine arning, предлагает всестороннее и актуальное введение в машинное обучение через призму вероятностного моделирования и байесовской теории принятия решений. В этой книге представлен подробный обзор математического фона, включая линейную алгебру и оптимизацию, базовое обучение с учителем и расширенные темы, такие как обучение с переносом и обучение без учителя. Автор, ранее написавший книгу «Машинное обучение: вероятностная перспектива», тщательно обновил содержание, чтобы отразить значительные достижения в этой области с 2012 года, особенно глубокое обучение. Новая книга включает онлайн-код на Python с использованием таких библиотек, как scikit-learn, JAX, PyTorch и TensorFlow, что позволяет студентам применять свои знания и воспроизводить фигуры. Этот практический компонент дополняет теоретические темы, обсуждаемые в книге, что делает его важным ресурсом для всех, кто хочет получить более глубокое понимание машинного обучения. Книга начинается с введения фундаментальных концепций теории вероятностей и байесовской теории принятия решений, обеспечивая прочную основу для последующих глав. Он охватывает линейную регрессию, логистическую регрессию и глубокие нейронные сети, причем каждая глава опирается на предыдущую, чтобы создать целостное и всестороннее введение в машинное обучение.
Livre : La technologie continue d'évoluer à un rythme sans précédent, il est essentiel pour nous de comprendre le processus de développement technologique et son impact sur les connaissances modernes. Probabilistic Machine Arning : An Introduction, une partie de la série Adaptive Computation and Machine Arning, offre une introduction complète et pertinente à l'apprentissage automatique à travers le prisme de la modélisation probabiliste et de la théorie de la prise de décision bayésienne. Ce livre présente un aperçu détaillé de l'arrière-plan mathématique, y compris l'algèbre linéaire et l'optimisation, l'apprentissage de base avec le professeur et des sujets avancés tels que l'apprentissage avec transfert et l'apprentissage sans professeur. L'auteur, qui avait déjà écrit le livre « Machine arning : A probability perspective », a soigneusement mis à jour le contenu pour refléter les progrès importants réalisés dans ce domaine depuis 2012, en particulier l'apprentissage profond. nouveau livre comprend du code en ligne sur Python en utilisant des bibliothèques telles que scikit-learn, JAX, PyTorch et TensorFlow, ce qui permet aux étudiants d'appliquer leurs connaissances et de reproduire des formes. Cette composante pratique complète les thèmes théoriques abordés dans le livre, ce qui en fait une ressource importante pour tous ceux qui souhaitent acquérir une meilleure compréhension de l'apprentissage automatique. livre commence par l'introduction des concepts fondamentaux de la théorie des probabilités et de la théorie de la prise de décision bayésienne, fournissant une base solide pour les chapitres suivants. Il couvre la régression linéaire, la régression logistique et les réseaux neuronaux profonds, chaque chapitre s'appuyant sur le précédent pour créer une introduction holistique et complète à l'apprentissage automatique.
Book: Probabilistic Machine arning An Introduction Adaptive Computation and Machine arning series A medida que la tecnología continúa evolucionando a un ritmo sin precedentes, es fundamental que comprendamos el proceso de desarrollo tecnológico y su impacto en el conocimiento actual. Mecanizado probabilístico: Una introducción, parte de la serie Adaptive Computation and Machine Arning, ofrece una introducción integral y actualizada al aprendizaje automático a través del prisma de la simulación probabilística y la teoría bayesiana de la toma de decisiones. Este libro ofrece una visión general detallada del fondo matemático, incluyendo álgebra lineal y optimización, aprendizaje básico con el profesor y temas avanzados como aprendizaje con transferencia y aprendizaje sin profesor. autor, que anteriormente había escrito el libro «Machine arning: probability Prospective», actualizó cuidadosamente los contenidos para reflejar los avances significativos en este campo desde 2012, especialmente el aprendizaje profundo. nuevo libro incluye código en línea en Python utilizando bibliotecas como scikit-learn, JAX, PyTorch y TensorFlow, lo que permite a los estudiantes aplicar sus conocimientos y reproducir figuras. Este componente práctico complementa los temas teóricos discutidos en el libro, convirtiéndolo en un recurso importante para cualquier persona que desee obtener una comprensión más profunda del aprendizaje automático. libro comienza con la introducción de los conceptos fundamentales de la teoría de la probabilidad y la teoría bayesiana de la toma de decisiones, proporcionando una base sólida para los capítulos posteriores. Abarca la regresión lineal, la regresión logística y las redes neuronales profundas, con cada capítulo basado en el anterior para crear una introducción holística y completa al aprendizaje automático.
Buch: Probabilistisches maschinelles rnen Eine Einführung Adaptive Datenverarbeitung und maschinelles rnen Da sich die Technologie in einem beispiellosen Tempo weiterentwickelt, ist es für uns von entscheidender Bedeutung, den technologischen Entwicklungsprozess und seine Auswirkungen auf das heutige Wissen zu verstehen. Probabilistic Machine arning: Eine Einführung, Teil der Adaptive Computation and Machine arning-Serie, bietet eine umfassende und relevante Einführung in das maschinelle rnen durch das Prisma der probabilistischen Modellierung und Bayes'schen Entscheidungstheorie. Dieses Buch bietet einen detaillierten Überblick über den mathematischen Hintergrund, einschließlich linearer Algebra und Optimierung, grundlegendes rnen mit einem hrer und erweiterte Themen wie Transferlernen und Nicht-hrerlernen. Der Autor, der zuvor das Buch Machine arning: A Probabilistic Perspective geschrieben hat, hat den Inhalt sorgfältig aktualisiert, um die bedeutenden Fortschritte in diesem Bereich seit 2012 widerzuspiegeln, insbesondere Deep arning. Das neue Buch enthält Online-Python-Code mit Bibliotheken wie scikit-learn, JAX, PyTorch und TensorFlow, die es den Schülern ermöglichen, ihr Wissen anzuwenden und Figuren zu reproduzieren. Diese praktische Komponente ergänzt die theoretischen Themen, die in dem Buch diskutiert werden, und macht es zu einer wichtigen Ressource für alle, die ein tieferes Verständnis des maschinellen rnens erlangen möchten. Das Buch beginnt mit einer Einführung in die grundlegenden Konzepte der Wahrscheinlichkeitstheorie und der Bayes'schen Entscheidungstheorie und bietet eine solide Grundlage für die nachfolgenden Kapitel. Es umfasst lineare Regression, logistische Regression und tiefe neuronale Netzwerke, wobei jedes Kapitel auf dem vorherigen aufbaut, um eine ganzheitliche und umfassende Einführung in das maschinelle rnen zu schaffen.
''
Book: Probabilistic Machine arning An Introduction Adaptive Computation and Machine arning series Teknoloji benzeri görülmemiş bir hızla gelişmeye devam ederken, teknolojik gelişme sürecini ve modern bilgi üzerindeki etkisini anlamamız çok önemlidir. Olasılıksal Makine Öğrenimi: Adaptif Hesaplama ve Makine Öğrenimi serisinin bir parçası olan bir Giriş, olasılıksal modelleme ve Bayes karar teorisi merceği aracılığıyla makine öğrenimine kapsamlı ve ilgili bir giriş sunar. Bu kitap, doğrusal cebir ve optimizasyon, temel denetimli öğrenme ve transpoze öğrenme ve denetimsiz öğrenme gibi ileri konular dahil olmak üzere matematiksel arka plana ayrıntılı bir genel bakış sunmaktadır. Daha önce Machine arning: A Probabilistic Perspective adlı kitabı yazan yazar, içeriği 2012'den bu yana alandaki önemli gelişmeleri, özellikle de derin öğrenmeyi yansıtacak şekilde titizlikle güncelledi. Yeni kitap, öğrencilerin bilgilerini uygulamalarına ve rakamları çoğaltmalarına olanak tanıyan scikit-learn, JAX, PyTorch ve TensorFlow gibi kütüphaneleri kullanan çevrimiçi Python kodunu içeriyor. Bu pratik bileşen, kitapta tartışılan teorik konuları tamamlayarak, makine öğrenimi hakkında daha derin bir anlayış kazanmak isteyen herkes için önemli bir kaynak haline getirmektedir. Kitap, olasılık teorisi ve Bayes karar teorisinin temel kavramlarını tanıtarak başlar ve sonraki bölümler için sağlam bir temel sağlar. Lineer regresyon, lojistik regresyon ve derin sinir ağlarını kapsar ve her bölüm makine öğrenimine bütünsel ve kapsamlı bir giriş oluşturmak için bir öncekini oluşturur.
كتاب: التعلم الآلي الاحتمالي مقدمة سلسلة الحوسبة التكيفية والتعلم الآلي مع استمرار تطور التكنولوجيا بوتيرة غير مسبوقة، من الضروري بالنسبة لنا فهم عملية التطور التكنولوجي وتأثيرها على المعرفة الحديثة. التعلم الآلي الاحتمالي: مقدمة، وهي جزء من سلسلة الحوسبة التكيفية والتعلم الآلي، تقدم مقدمة شاملة وذات صلة بالتعلم الآلي من خلال عدسة النمذجة الاحتمالية ونظرية القرار البايزية. يقدم هذا الكتاب لمحة عامة مفصلة عن الخلفية الرياضية، بما في ذلك الجبر الخطي والتحسين، والتعلم الأساسي تحت الإشراف، والمواضيع المتقدمة مثل التعلم المنقول والتعلم غير الخاضع للإشراف. قام المؤلف، الذي كتب سابقًا كتاب التعلم الآلي: منظور احتمالي، بتحديث المحتوى بدقة ليعكس التطورات الكبيرة في هذا المجال منذ عام 2012، وخاصة التعلم العميق. يتضمن الكتاب الجديد رمز Python عبر الإنترنت باستخدام مكتبات مثل scikit-learn و JAX و PyTorch و TensorFlow، مما يسمح للطلاب بتطبيق معرفتهم وإعادة إنتاج الأرقام. يكمل هذا المكون العملي الموضوعات النظرية التي تمت مناقشتها في الكتاب، مما يجعله مصدرًا مهمًا لأي شخص يريد اكتساب فهم أعمق للتعلم الآلي. يبدأ الكتاب بتقديم مفاهيم أساسية لنظرية الاحتمالات ونظرية القرار البايزية، مما يوفر أساسًا صلبًا للفصول اللاحقة. يغطي الانحدار الخطي والانحدار اللوجستي والشبكات العصبية العميقة، حيث يعتمد كل فصل على الفصل السابق لإنشاء مقدمة شاملة وشاملة للتعلم الآلي.
