
BOOKS - PROGRAMMING - Multi-Agent Machine Learning A Reinforcement Approach

Multi-Agent Machine Learning A Reinforcement Approach
Author: H.M. Schwartz
Year: 2014
Pages: 256
Format: EPUB | PDF CONV
File size: 21.7 MB
Language: ENG
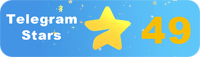
Year: 2014
Pages: 256
Format: EPUB | PDF CONV
File size: 21.7 MB
Language: ENG
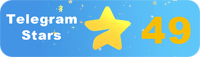
''
