
BOOKS - PROGRAMMING - Explainable Artificial Intelligence for Biomedical Applications

Explainable Artificial Intelligence for Biomedical Applications
Author: Utku Kose, Deepak Gupta, Xi Chen
Year: 2023
Pages: 421
Format: PDF
File size: 30.8 MB
Language: ENG
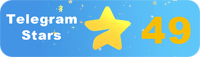
Year: 2023
Pages: 421
Format: PDF
File size: 30.8 MB
Language: ENG
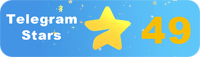
''
