
BOOKS - PROGRAMMING - Deep Learning Techniques and Optimization Strategies in Big Dat...

Deep Learning Techniques and Optimization Strategies in Big Data Analytics
Author: J Joshua Thomas, Pinar Karagoz, B Bazeer Ahamed
Year: 2020
Pages: 381
Format: EPUB | PDF
File size: 23,9 MB, 12,3 MB
Language: ENG
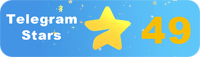
Year: 2020
Pages: 381
Format: EPUB | PDF
File size: 23,9 MB, 12,3 MB
Language: ENG
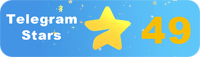
. Book Deep Learning Techniques and Optimization Strategies in Big Data Analytics Introduction: In today's fast-paced technological landscape, big data analytics has become an integral part of various industries such as healthcare, finance, marketing, and social media. The sheer volume of data generated every day requires advanced techniques to process, analyze, and make sense of it all. This is where deep learning techniques come into play. Deep Learning Techniques and Optimization Strategies in Big Data Analytics offers a comprehensive overview of the latest developments in deep learning methodologies, their applications, and optimization strategies to help readers grasp the full potential of these cutting-edge techniques. Chapter 1: Introduction to Deep Learning The book begins by introducing the fundamental concepts of deep learning and its significance in the field of big data analytics. It covers the history of deep learning, its evolution, and the current state of the technology. Readers will gain an understanding of how deep learning has revolutionized the way we approach big data analysis and what it can offer in terms of accuracy, speed, and scalability. Chapter 2: Data Integration and Computational Modeling This chapter delves into the intricacies of data integration and computational modeling, highlighting the challenges associated with integrating large datasets and the importance of computational modeling in deep learning. Readers will learn about the different types of deep learning models, their strengths, and weaknesses, as well as the various optimization techniques used to improve their performance.
.Book Методы глубокого обучения и стратегии оптимизации в аналитике больших данных Введение: В современном быстро развивающемся технологическом ландшафте аналитика больших данных стала неотъемлемой частью различных отраслей, таких как здравоохранение, финансы, маркетинг и социальные сети. Огромный объем данных, генерируемых каждый день, требует передовых методов для обработки, анализа и осмысления всего этого. Здесь в игру вступают техники глубокого обучения. Методы глубокого обучения и стратегии оптимизации в аналитике больших данных предлагают полный обзор последних разработок в методологиях глубокого обучения, их приложениях и стратегиях оптимизации, чтобы помочь читателям понять весь потенциал этих передовых методов. Глава 1: Введение в глубокое обучение Книга начинается с введения фундаментальных концепций глубокого обучения и его значения в области аналитики больших данных. Он охватывает историю глубокого обучения, его эволюцию и текущее состояние технологии. Читатели получат представление о том, как глубокое обучение произвело революцию в подходе к анализу больших данных и что оно может предложить с точки зрения точности, скорости и масштабируемости. Глава 2: Интеграция данных и вычислительное моделирование В этой главе рассматриваются тонкости интеграции данных и вычислительного моделирования, освещаются проблемы, связанные с интеграцией больших наборов данных, и важность вычислительного моделирования в глубоком обучении. Читатели узнают о различных типах моделей глубокого обучения, их сильных и слабых сторонах, а также о различных методах оптимизации, используемых для повышения их производительности.
.Book Title : Méthodes d'apprentissage profond et stratégies d'optimisation en analyse de Big Data Introduction : Dans le paysage technologique en évolution rapide d'aujourd'hui, l'analyse de Big Data est devenue une partie intégrante de divers secteurs tels que la santé, la finance, le marketing et les réseaux sociaux. La grande quantité de données générées chaque jour nécessite des techniques de pointe pour traiter, analyser et comprendre tout cela. C'est là que les techniques d'apprentissage profond entrent en jeu. s méthodes de deep learning et les stratégies d'optimisation en analyse Big Data offrent un aperçu complet des derniers développements dans les méthodologies de deep learning, leurs applications et les stratégies d'optimisation pour aider les lecteurs à comprendre tout le potentiel de ces meilleures pratiques. Chapitre 1 : Introduction à l'apprentissage profond livre commence par l'introduction des concepts fondamentaux de l'apprentissage profond et de son importance dans le domaine de l'analyse des grandes données. Il couvre l'histoire de l'apprentissage profond, son évolution et l'état actuel de la technologie. s lecteurs auront une idée de la façon dont l'apprentissage profond a révolutionné l'approche de l'analyse des données volumineuses et de ce qu'il peut offrir en termes de précision, de vitesse et d'évolutivité. Chapitre 2 : Intégration des données et modélisation de l'informatique Ce chapitre traite des subtilités de l'intégration des données et de la modélisation de l'informatique, met en lumière les défis liés à l'intégration de grands ensembles de données et l'importance de la modélisation de l'informatique dans l'apprentissage en profondeur. s lecteurs apprennent les différents types de modèles d'apprentissage profond, leurs forces et leurs faiblesses, ainsi que les différentes méthodes d'optimisation utilisées pour améliorer leurs performances.
.Book Técnicas de aprendizaje profundo y estrategias de optimización en análisis de big data Introducción: En el panorama tecnológico en rápida evolución, la analítica de big data se ha convertido en una parte integral de diversas industrias como la salud, las finanzas, el marketing y las redes sociales. La enorme cantidad de datos que se generan cada día requiere de técnicas avanzadas para procesar, analizar y comprender todo esto. Aquí entran en juego las técnicas del aprendizaje profundo. técnicas de aprendizaje profundo y las estrategias de optimización en análisis de big data ofrecen una visión completa de los últimos desarrollos en metodologías de aprendizaje profundo, sus aplicaciones y estrategias de optimización para ayudar a los lectores a comprender todo el potencial de estas técnicas avanzadas. Capítulo 1: Introducción al aprendizaje profundo libro comienza con la introducción de los conceptos fundamentales del aprendizaje profundo y su significado en el campo de la analítica de big data. Abarca la historia del aprendizaje profundo, su evolución y el estado actual de la tecnología. lectores tendrán una idea de cómo el aprendizaje profundo ha revolucionado el enfoque del análisis de big data y qué puede ofrecer en términos de precisión, velocidad y escalabilidad. Capítulo 2: Integración de datos y simulación computacional Este capítulo aborda las sutilezas de la integración de datos y la simulación computacional, destaca los desafíos relacionados con la integración de grandes conjuntos de datos y la importancia de la simulación computacional en el aprendizaje profundo. lectores aprenden sobre los diferentes tipos de modelos de aprendizaje profundo, sus fortalezas y debilidades, así como los diferentes métodos de optimización utilizados para mejorar su rendimiento.
.Book Métodos de aprendizagem profunda e estratégias de otimização em análise de big data Introdução: No panorama tecnológico em desenvolvimento moderno, o analista de big data tornou-se parte integrante de vários setores, como saúde, finanças, marketing e redes sociais. A grande quantidade de dados gerados todos os dias requer técnicas avançadas de processamento, análise e compreensão. É aqui que entram em jogo as técnicas de aprendizagem profunda. Técnicas de aprendizagem profunda e estratégias de otimização em análise de big data oferecem uma visão completa dos últimos desenvolvimentos em metodologias de aprendizagem profunda, suas aplicações e estratégias de otimização para ajudar os leitores a compreender todo o potencial dessas técnicas avançadas. Capítulo 1: Introdução ao aprendizado profundo Livro começa introduzindo conceitos fundamentais de aprendizado profundo e sua importância no campo dos analistas de big data. Ele abrange o histórico de aprendizado profundo, sua evolução e o estado atual da tecnologia. Os leitores terão uma ideia de como a aprendizagem profunda revolucionou a abordagem da análise de grandes dados e o que ela pode oferecer em termos de precisão, velocidade e escalabilidade. Capítulo 2: Integração de Dados e mulação Computacional Este capítulo aborda as sutilezas da integração de dados e da simulação computacional, os desafios da integração de grandes conjuntos de dados e a importância da simulação computacional no aprendizado profundo. Os leitores aprendem sobre os diferentes tipos de modelos de aprendizado profundo, seus pontos fortes e fracos, e sobre os diferentes métodos de otimização usados para melhorar sua produtividade.
.Book Metodi di apprendimento approfondito e strategie di ottimizzazione nell'analisi dei big data Introduzione: in un panorama tecnologico in continua evoluzione, l'analista dei big data è diventato parte integrante di diversi settori come salute, finanza, marketing e social media. La grande quantità di dati generati ogni giorno richiede procedure ottimali per elaborare, analizzare e comprendere tutto questo. Qui entrano in gioco i tecnici dell'apprendimento profondo. tecniche di formazione approfondita e le strategie di ottimizzazione dell'analisi dei big data offrono una panoramica completa degli ultimi sviluppi delle metodologie di apprendimento approfondito, delle applicazioni e delle strategie di ottimizzazione per aiutare i lettori a comprendere il potenziale di queste best practice. Capitolo 1: Introduzione all'apprendimento profondo Il libro inizia introducendo concetti fondamentali di apprendimento profondo e il suo significato nel campo degli analisti di big data. Copre la storia dell'apprendimento profondo, la sua evoluzione e lo stato attuale della tecnologia. I lettori avranno un'idea di come l'apprendimento profondo abbia rivoluzionato l'approccio all'analisi dei grandi dati e di cosa possa offrire in termini di precisione, velocità e scalabilità. Capitolo 2: Integrazione dei dati e simulazione computazionale In questo capitolo vengono descritte le finezze dell'integrazione dei dati e della simulazione computazionale, vengono illustrate le problematiche legate all'integrazione di grandi set di dati e l'importanza della simulazione computazionale nell'apprendimento approfondito. I lettori conosceranno i diversi tipi di modelli di apprendimento approfondito, i loro punti di forza e debolezza e i vari metodi di ottimizzazione utilizzati per migliorare le loro prestazioni.
.Buch Titel: Deep-arning-Methoden und Optimierungsstrategien in der Big-Data-Analyse Einleitung: In der heutigen schnelllebigen Technologielandschaft ist die Big-Data-Analyse zu einem integralen Bestandteil verschiedener Branchen wie Gesundheitswesen, Finanzen, Marketing und Social Media geworden. Die enorme Menge an Daten, die jeden Tag generiert wird, erfordert fortschrittliche Techniken, um all dies zu verarbeiten, zu analysieren und zu verstehen. Hier kommen Deep-arning-Techniken ins Spiel. Deep-arning-Techniken und Optimierungsstrategien in der Big-Data-Analyse bieten einen vollständigen Überblick über die neuesten Entwicklungen in Deep-arning-Methoden, deren Anwendungen und Optimierungsstrategien, um den sern zu helfen, das volle Potenzial dieser fortschrittlichen Techniken zu verstehen. Kapitel 1: Einführung in Deep arning Das Buch beginnt mit der Einführung grundlegender Konzepte von Deep arning und seiner Bedeutung im Bereich der Big Data Analytics. Es umfasst die Geschichte des Deep arning, seine Entwicklung und den aktuellen Stand der Technik. Die ser erhalten einen Einblick, wie Deep arning den Ansatz der Big Data-Analyse revolutioniert hat und was es in Bezug auf Genauigkeit, Geschwindigkeit und Skalierbarkeit zu bieten hat. Kapitel 2: Datenintegration und Computermodellierung Dieses Kapitel befasst sich mit den Feinheiten der Datenintegration und Computermodellierung, beleuchtet die Herausforderungen bei der Integration großer Datensätze und die Bedeutung der Computermodellierung im Deep arning. Die ser lernen die verschiedenen Arten von Deep-arning-Modellen, ihre Stärken und Schwächen sowie die verschiedenen Optimierungsmethoden kennen, die zur Verbesserung ihrer istung verwendet werden.
.Book Tytuł: Techniki głębokiego uczenia się i strategie optymalizacji w Big Data Analytics Wprowadzenie: W dzisiejszym szybko rozwijającym się krajobrazie technologicznym, analiza dużych danych stała się integralną częścią różnych branż, takich jak opieka zdrowotna, finanse, marketing i media społecznościowe. Sama objętość danych generowanych każdego dnia wymaga zaawansowanych technik przetwarzania, analizy i sensu tego wszystkiego. Tutaj wchodzą w grę techniki głębokiego uczenia się. Techniki głębokiego uczenia się i strategie optymalizacji w analizie dużych danych oferują pełny przegląd najnowszych osiągnięć w metodologii głębokiego uczenia się, ich zastosowań i strategii optymalizacji, aby pomóc czytelnikom zrozumieć pełny potencjał tych najlepszych praktyk. Rozdział 1: Wprowadzenie do głębokiego uczenia się Książka rozpoczyna się od wprowadzenia podstawowych koncepcji głębokiego uczenia się i jego konsekwencji w dziedzinie analizy dużych danych. Obejmuje historię głębokiego uczenia się, jego ewolucję i obecny stan technologii. Czytelnicy zyskają wgląd w to, jak głębokie uczenie się zrewolucjonizowało podejście do analizy dużych danych i co ma do zaoferowania w zakresie dokładności, szybkości i skalowalności. Rozdział 2: Integracja danych i modelowanie obliczeniowe Niniejszy rozdział omawia zawiłości integracji danych i modelowania obliczeniowego, podkreśla wyzwania związane z integracją dużych zbiorów danych oraz znaczenie modelowania obliczeniowego w głębokim uczeniu się. Czytelnicy poznają różne rodzaje modeli głębokiego uczenia się, ich mocne i słabe strony oraz różne techniki optymalizacji stosowane do poprawy ich wydajności.
. Book Gather: Deep arning Technologies and Optimization Strategies in Big Data Analytics Introduction: בנוף הטכנולוגי המתפתח במהירות, ביג אנליטיקס הפך לחלק בלתי נפרד מתעשיות שונות כגון בריאות, פיננסים, שיווינדים. הנפח העצום של נתונים הנוצרים בכל יום דורש טכניקות מתקדמות כדי לעבד, לנתח ולהבין את כל זה. כאן משתלבות טכניקות למידה מעמיקה. שיטות למידה עמוקה ואסטרטגיות אופטימיזציה באנליטיקת מידע גדולה מציעות סקירה מלאה של התפתחויות האחרונות במתודולוגיות למידה עמוקה, ביישומים שלהם ובאסטרטגיות אופטימיזציה פרק 1: מבוא ללמידה עמוקה הספר מתחיל בהצגת מושגי היסוד של למידה עמוקה והשלכותיה בתחום של ניתוח נתונים גדולים. הוא מכסה את ההיסטוריה של למידה עמוקה, האבולוציה שלה ואת המצב הנוכחי של הטכנולוגיה. הקוראים ישיגו תובנה על אופן הלמידה העמוקה שחוללה מהפכה בגישה לניתוח נתונים גדולים ומה יש לה להציע במונחים של דיוק, מהירות וסקפטיות. פרק 2: אינטגרציית נתונים ומידול חישובי פרק זה דן במורכבות של אינטגרציית נתונים ומידול חישובי, מדגיש את האתגרים הקשורים באינטגרציה בין מערכות מידע גדולות, ואת החשיבות של מודול חישובי בלמידה עמוקה. הקוראים ילמדו על סוגים שונים של מודלים ללמידה עמוקה, על החוזקות והחולשות שלהם, ועל שיטות האופטימיזציה השונות המשמשות לשיפור ביצועיהם.''
.Book Büyük Veri Analitiğinde Derin Öğrenme Teknikleri ve Optimizasyon Stratejileri Giriş: Günümüzün hızla gelişen teknoloji ortamında, büyük veri analitiği sağlık, finans, pazarlama ve sosyal medya gibi çeşitli endüstrilerin ayrılmaz bir parçası haline gelmiştir. Her gün üretilen verilerin hacmi, hepsini işlemek, analiz etmek ve anlamlandırmak için gelişmiş teknikler gerektirir. İşte bu noktada derin öğrenme teknikleri devreye giriyor. Büyük veri analitiğindeki derin öğrenme teknikleri ve optimizasyon stratejileri, okuyucuların bu en iyi uygulamaların tam potansiyelini anlamalarına yardımcı olmak için derin öğrenme metodolojilerindeki son gelişmelere, uygulamalarına ve optimizasyon stratejilerine tam bir genel bakış sunar. Bölüm 1: Derin Öğrenmeye Giriş Kitap, derin öğrenmenin temel kavramlarını ve büyük veri analitiği alanındaki etkilerini tanıtarak başlar. Derin öğrenmenin tarihini, evrimini ve teknolojinin mevcut durumunu kapsar. Okuyucular, derin öğrenmenin büyük veri analizine yaklaşımda nasıl bir devrim yarattığı ve doğruluk, hız ve ölçeklenebilirlik açısından neler sunabileceği konusunda fikir edineceklerdir. Bölüm 2: Veri Entegrasyonu ve Hesaplamalı Modelleme Bu bölüm, veri entegrasyonu ve hesaplamalı modellemenin inceliklerini tartışır, büyük veri setlerini entegre etmekle ilgili zorlukları ve derin öğrenmede hesaplamalı modellemenin önemini vurgular. Okuyucular, farklı derin öğrenme modellerini, güçlü ve zayıf yönlerini ve performanslarını iyileştirmek için kullanılan farklı optimizasyon tekniklerini öğreneceklerdir.
عنوان الكتاب: تقنيات التعلم العميق واستراتيجيات التحسين في تحليلات البيانات الضخمة مقدمة: في المشهد التكنولوجي سريع التطور اليوم، أصبحت تحليلات البيانات الضخمة جزءًا لا يتجزأ من مختلف الصناعات مثل الرعاية الصحية والتمويل والتسويق ووسائل التواصل الاجتماعي. يتطلب الحجم الهائل للبيانات التي يتم إنشاؤها كل يوم تقنيات متقدمة لمعالجتها وتحليلها وفهمها. هذا هو المكان الذي تلعب فيه تقنيات التعلم العميق. تقدم تقنيات التعلم العميق واستراتيجيات التحسين في تحليلات البيانات الضخمة نظرة عامة كاملة على التطورات الأخيرة في منهجيات التعلم العميق وتطبيقاتها واستراتيجيات التحسين لمساعدة القراء على فهم الإمكانات الكاملة لهذه الممارسات الفضلى. الفصل 1: مقدمة إلى التعلم العميق يبدأ الكتاب بتقديم المفاهيم الأساسية للتعلم العميق وآثاره في مجال تحليلات البيانات الضخمة. يغطي تاريخ التعلم العميق وتطوره والحالة الحالية للتكنولوجيا. سيكتسب القراء نظرة ثاقبة حول كيفية إحداث التعلم العميق ثورة في نهج تحليل البيانات الضخمة وما يجب أن يقدمه من حيث الدقة والسرعة وقابلية التوسع. الفصل 2: تكامل البيانات والنمذجة الحسابية يناقش هذا الفصل تعقيدات تكامل البيانات والنمذجة الحسابية، ويسلط الضوء على التحديات المرتبطة بدمج مجموعات البيانات الكبيرة، وأهمية النمذجة الحسابية في التعلم العميق. سيتعرف القراء على الأنواع المختلفة لنماذج التعلم العميق ونقاط قوتها وضعفها وتقنيات التحسين المختلفة المستخدمة لتحسين أدائهم.
.Book 제목: 빅 데이터 분석 소개의 딥 러닝 기술 및 최적화 전략: 오늘날의 빠르게 진화하는 기술 환경에서 빅 데이터 분석은 건강 관리, 금융, 마케팅 및 소셜 미디어와 같은 다양한 산업의 필수 부분이되었습니다. 매일 생성되는 많은 양의 데이터는 모든 기술을 처리, 분석 및 이해하기위한 고급 기술이 필요합니다. 딥 러닝 기술이 사용되는 곳입니다. 빅 데이터 분석의 딥 러닝 기술 및 최적화 전략은 딥 러닝 방법론, 응용 프로그램 및 최적화 전략의 최근 개발에 대한 완전한 개요를 제공하여 독자가 이러한 모범 사례의 모든 잠재력을 이해하도록 돕습니다. 1 장: 딥 러닝 소개이 책은 딥 러닝의 기본 개념과 빅 데이터 분석 분야에서의 의미를 소개함으로써 시작됩니다. 딥 러닝의 역사, 진화 및 현재 기술 상태를 다룹니다. 독자는 딥 러닝이 빅 데이터 분석에 대한 접근 방식에 혁명을 일으킨 방법과 정확성, 속도 및 확장 성 측면에서 제공해야 할 사항에 대한 통찰력을 얻습니다. 2 장: 데이터 통합 및 전산 모델링 독자는 다양한 유형의 딥 러닝 모델, 강점 및 약점, 성능 향상에 사용되는 다양한 최적화 기술에 대해 배웁니다.
。Book Title: Deep arning Techniques and Optimization Strategies in Big Data Analyticsはじめに:今日急速に進化しているテクノロジー環境において、ビッグデータ分析は、ヘルスケア、金融、マーケティング、ソーシャルメディアなどの様々な産業に不可欠となっています。毎日生成される大量のデータには、処理、分析、理解するための高度な技術が必要です。そこでディープラーニングのテクニックが生まれます。ビッグデータ分析におけるディープラーニング技術と最適化戦略は、ディープラーニング手法、そのアプリケーション、および最適化戦略の最近の進展の完全な概要を提供し、読者がこれらのベストプラクティスの可能性を完全に理解できるようにします。第1章:ディープラーニングの入門本書は、ディープラーニングの基本的な概念とビッグデータ分析の分野におけるその意味を紹介することから始まります。これは、ディープラーニングの歴史、その進化、技術の現在の状態をカバーしています。読者は、ディープラーニングがビッグデータ分析へのアプローチにどのように革命をもたらしたか、そしてそれが正確さ、スピード、スケーラビリティの観点から何を提供しなければならないかについて洞察を得るでしょう。第2章:データ統合と計算モデリングこの章では、データ統合と計算モデリングの複雑さについて説明し、大規模なデータセットの統合に関連する課題、および深層学習における計算モデリングの重要性を強調します。読者は、さまざまなタイプのディープラーニングモデル、その長所と短所、パフォーマンスを向上させるために使用されるさまざまな最適化技術について学びます。
.图书标题:大数据分析中的深度学习方法和优化策略介绍:在当今快速发展的技术环境中,大数据分析已成为医疗保健,金融,市场营销和社交媒体等不同行业的组成部分。每天生成的大量数据需要先进的技术来处理、分析和理解所有这些。在这里,深度学习技术开始发挥作用。大数据分析中的深度学习方法和优化策略提供了深度学习方法,其应用和优化策略的最新发展的完整概述,以帮助读者了解这些最佳实践的全部潜力。第一章:深度学习入门本书首先介绍了深度学习的基本概念及其在大数据分析领域的意义。它涵盖了深度学习的历史,其演变以及该技术的当前状态。读者将了解深度学习如何彻底改变大数据分析方法,以及它在准确性、速度和可伸缩性方面可以提供什么。第二章:数据集成与计算建模本章探讨数据集成与计算建模的复杂性,突出大数据集集成带来的挑战,以及计算建模在深度学习中的重要性。读者将了解不同类型的深度学习模型,它们的优缺点以及用于提高其性能的不同优化方法。
