
BOOKS - PROGRAMMING - Data Science in Production Building Scalable Model Pipelines wi...

Data Science in Production Building Scalable Model Pipelines with Python
Author: Ben Weber
Year: 2020
Pages: 234
Format: PDF | EPUB
File size: 10 MB
Language: ENG
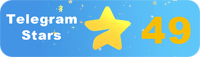
Year: 2020
Pages: 234
Format: PDF | EPUB
File size: 10 MB
Language: ENG
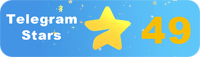
Data Science in Production - Building Scalable Model Pipelines with Python As technology continues to evolve at an unprecedented pace, it's becoming increasingly important for data scientists to understand the process of developing modern knowledge and its impact on humanity. In their latest book, "Data Science in Production - Building Scalable Model Pipelines with Python authors [Author Names] provide a comprehensive guide to scaling up Python code for production-ready model pipelines, highlighting the need for data scientists to adapt and evolve alongside technology. The book begins by emphasizing the importance of understanding the technological process of developing modern knowledge, and how it has the potential to unify people in a warring state. The authors argue that by embracing this paradigm shift, data scientists can not only add value to their organizations but also contribute to the betterment of society as a whole. They stress the need for data scientists to be proactive in developing their personal paradigms for perceiving the technological process, in order to stay ahead of the curve and remain relevant in the ever-changing landscape of data science. The book then delves into the practical aspects of building scalable model pipelines using Python, providing readers with hands-on experience in setting up machine learning models as web endpoints, serverless functions, and streaming pipelines in multiple cloud environments. The authors demonstrate how to scale up prototype models to production-ready code, using libraries such as Pandas and scikit-learn. They emphasize the importance of considering the entire production pipeline, from data ingestion to deployment, when building scalable models.
''
Data Science in Production-Pythonでスケーラブルなモデルパイプラインを構築技術が前例のないペースで進歩し続けるにつれて、データサイエンティストが現代の知識を開発するプロセスとその人類への影響を理解することはますます重要になっています。最新の著書「Data Science in Production-Building Scalable Model Pipelines with Python」では、[Author Names]は、Pythonコードをスケーリングするための包括的なガイドを提供しています。この本は、近代的な知識の発展の技術的プロセスを理解することの重要性と、戦争状態で人々を団結させる可能性をどのように持っているかを強調することから始まります。著者たちは、このパラダイムシフトを受け入れることで、データサイエンティストは組織に付加価値をもたらすだけでなく、社会全体の改善にも貢献できると主張している。彼らは、変化するデータサイエンスのランドスケープにおいて常に関連性を保つために、データサイエンティストが個人的なプロセス認識パラダイムの開発に積極的に取り組む必要性を強調しています。この本は、Pythonを使用してスケーラブルなモデルパイプラインを構築する実用性を掘り下げ、複数のクラウド環境で機械学習モデルをWebエンドポイント、サーバーレス機能、ストリーミングパイプラインとして設定する実践的な経験を読者に提供します。Pandasやscikit-learnなどのライブラリを使用して、プロトタイプモデルを生産準備コードにスケーリングする方法を実証している。彼らは、スケーラブルなモデルを構築する際に、データの受領から展開まで、生産パイプライン全体を考慮することの重要性を強調しています。
