
BOOKS - PROGRAMMING - Variational Bayesian Learning Theory

Variational Bayesian Learning Theory
Author: Shinichi Nakajima, Kazuho Watanabe
Year: 2019
Pages: 561
Format: PDF
File size: 10.1 MB
Language: ENG
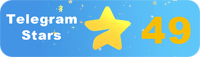
Year: 2019
Pages: 561
Format: PDF
File size: 10.1 MB
Language: ENG
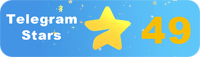
''
