
BOOKS - OS AND DB - Practical Synthetic Data Generation Balancing Privacy and the Bro...

Practical Synthetic Data Generation Balancing Privacy and the Broad Availability of Data
Author: Khaled El Emam, Lucy Mosquera, and Richard Hoptroff
Year: 2020-05-19
Pages: 166
Format: PDF/EPUB
File size: 17.5 MB
Language: ENG
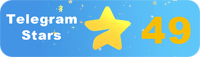
Year: 2020-05-19
Pages: 166
Format: PDF/EPUB
File size: 17.5 MB
Language: ENG
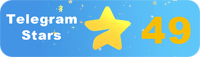
''
