
BOOKS - Probability and Statistics for Machine Learning A Textbook

Probability and Statistics for Machine Learning A Textbook
Author: Charu C. Aggarwal
Year: 2024
Pages: 530
Format: PDF | EPUB
File size: 41.5 MB
Language: ENG
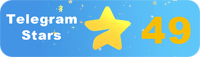
Year: 2024
Pages: 530
Format: PDF | EPUB
File size: 41.5 MB
Language: ENG
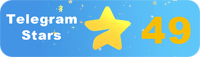
The book "Probability and Statistics for Machine Learning A Textbook" by Dustin Trani, et al. , is a comprehensive guide to understanding the fundamental concepts of probability and statistics in machine learning. The book covers a wide range of topics, from basic concepts such as probability distributions and statistical inference to more advanced topics like Bayesian inference and deep learning. The authors emphasize the importance of understanding these concepts in order to develop a personal paradigm for perceiving the technological process of developing modern knowledge, which they argue is essential for the survival of humanity and the unity of people in a warring state. The book begins by introducing the reader to the basics of probability theory, including the concept of probability spaces and events, and the laws of probability. It then delves into more advanced topics such as conditional probability, Bayes' theorem, and Markov chains. The authors also cover statistical inference, including hypothesis testing and confidence intervals. They provide a detailed explanation of the different types of statistical tests and their applications in machine learning. One of the unique aspects of this book is its focus on the practical application of probability and statistics in machine learning. The authors use real-world examples to illustrate how these concepts are used in industry and research. For example, they discuss the use of Gaussian processes in robotics and the application of Markov chain Monte Carlo (MCMC) methods in bioinformatics. They also provide code examples using Python and R, making it accessible to readers who are new to the field. The book also covers more advanced topics such as deep learning, which is a subset of machine learning that uses neural networks to analyze data. The authors explain how deep learning models can be used for tasks such as image recognition and natural language processing.
Книга «Probability and Statistics for Machine arning A Textbook» Дастина Трани (Dustin Trani) и др. представляет собой всеобъемлющее руководство по пониманию фундаментальных понятий вероятности и статистики в машинном обучении. Книга охватывает широкий спектр тем, от базовых понятий, таких как распределение вероятностей и статистический вывод, до более продвинутых тем, таких как байесовский вывод и глубокое обучение. Авторы подчеркивают важность понимания этих понятий, чтобы выработать личностную парадигму восприятия технологического процесса развития современных знаний, которые, как они утверждают, необходимы для выживания человечества и единства людей в воюющем государстве. Книга начинается с ознакомления читателя с основами теории вероятностей, включая понятие вероятностных пространств и событий, и законами вероятности. Затем он углубляется в более продвинутые темы, такие как условная вероятность, теорема Байеса и цепи Маркова. Авторы также охватывают статистический вывод, включая проверку гипотез и доверительные интервалы. Они дают подробное объяснение различных типов статистических тестов и их применения в машинном обучении. Одним из уникальных аспектов этой книги является её направленность на практическое применение вероятности и статистики в машинном обучении. Авторы используют реальные примеры, чтобы проиллюстрировать, как эти концепции используются в промышленности и исследованиях. Например, они обсуждают использование гауссовых процессов в робототехнике и применение методов марковской цепи Монте-Карло (MCMC) в биоинформатике. Они также предоставляют примеры кода с использованием Python и R, делая его доступным для читателей, которые являются новичками в этой области. Книга также охватывает более продвинутые темы, такие как глубокое обучение, которое представляет собой подмножество машинного обучения, использующее нейронные сети для анализа данных. Авторы объясняют, как можно использовать модели глубокого обучения для таких задач, как распознавание изображений и обработка естественного языка.
''
