
BOOKS - Neural Networks and Deep Learning: A Textbook

Neural Networks and Deep Learning: A Textbook
Author: Charu C. Aggarwal
Year: September 30, 2018
Format: PDF
File size: PDF 51 MB
Language: English
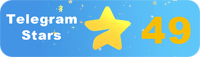
Year: September 30, 2018
Format: PDF
File size: PDF 51 MB
Language: English
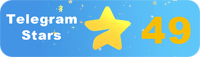
Neural Networks and Deep Learning: A Textbook As we continue to evolve in our technological advancements, it is crucial that we understand the process of developing modern knowledge and its impact on humanity's survival. This textbook provides a comprehensive overview of both classical and modern models in deep learning, focusing on the theory and algorithms of neural networks and their applications in various fields. The Basics of Neural Networks Chapters one and two delve into the fundamentals of neural networks, exploring how traditional machine learning models can be understood as special cases of neural networks. These chapters cover support vector machines, linear logistic regression, singular value decomposition, matrix factorization, and recommender systems, providing a solid foundation for understanding the relationship between traditional machine learning and neural networks. Additionally, recent feature engineering methods like word2vec are discussed. Training and Regularization Chapters three and four dive deeper into training and regularization techniques, offering a detailed discussion of these critical aspects of neural networks. These chapters cover radial basis function (RBF) networks, restricted Boltzmann machines (RBMs), and other advanced topics. Advanced Topics Chapters seven and eight explore more advanced topics such as recurrent neural networks (RNNs), convolutional neural networks (CNNs), and deep reinforcement learning. These chapters also introduce Kohonen self-organizing maps, generative adversarial networks (GANs), and other cutting-edge techniques. Applications of Neural Networks Chapters five and six showcase the practical applications of neural networks in various fields, including image captioning, image classification, reinforcement learning, and text analytics.
Нейронные сети и глубокое обучение: Учебник По мере того, как мы продолжаем развиваться в наших технологических достижениях, крайне важно, чтобы мы понимали процесс развития современных знаний и его влияние на выживание человечества. В этом учебнике представлен всесторонний обзор как классических, так и современных моделей в глубоком обучении с упором на теорию и алгоритмы нейронных сетей и их применения в различных областях. Основы нейронных сетей Главы первая и вторая углубляются в основы нейронных сетей, исследуя, как традиционные модели машинного обучения можно понимать как частные случаи нейронных сетей. Эти главы охватывают вспомогательные векторные машины, линейную логистическую регрессию, разложение сингулярных значений, разложение матриц на множители и рекомендательные системы, обеспечивая прочную основу для понимания взаимосвязи между традиционным машинным обучением и нейронными сетями. Кроме того, обсуждаются последние методы конструирования элементов, такие как word2vec. Обучение и регуляризация Главы третья и четвертая углубляются в методы обучения и регуляризации, предлагая подробное обсуждение этих критических аспектов нейронных сетей. Эти главы охватывают сети радиальных базисных функций (RBF), ограниченные машины Больцмана (RBM) и другие продвинутые темы. Расширенные темы В семи и восьми главах рассматриваются более продвинутые темы, такие как рекуррентные нейронные сети (RNN), сверточные нейронные сети (CNN) и глубокое обучение с подкреплением. В этих главах также представлены самоорганизующиеся карты Кохонена, генеративные состязательные сети (GAN) и другие передовые методы. Приложения нейронных сетей Главы пять и шесть демонстрируют практические применения нейронных сетей в различных областях, включая субтитры изображений, классификацию изображений, обучение подкреплению и текстовую аналитику.
Réseaux neuronaux et Deep arning : Tutoriel Alors que nous continuons à évoluer dans nos progrès technologiques, il est essentiel que nous comprenions le processus de développement des connaissances modernes et son impact sur la survie de l'humanité. Ce tutoriel présente un aperçu complet des modèles classiques et modernes en apprentissage profond, en mettant l'accent sur la théorie et les algorithmes des réseaux neuronaux et leurs applications dans différents domaines. s fondements des réseaux neuronaux s chapitres premier et deuxième s'inscrivent dans les fondements des réseaux neuronaux, explorant comment les modèles traditionnels d'apprentissage automatique peuvent être compris comme des cas particuliers de réseaux neuronaux. Ces chapitres couvrent les machines vectorielles auxiliaires, la régression logistique linéaire, la décomposition des valeurs singulières, la décomposition des matrices en multiplicateurs et les systèmes de recommandation, fournissant une base solide pour comprendre la relation entre l'apprentissage machine traditionnel et les réseaux neuronaux. En outre, les dernières méthodes de conception d'éléments telles que word2vec sont discutées. Formation et régularisation s chapitres 3 et 4 approfondiront les méthodes d'apprentissage et de régularisation en proposant une discussion détaillée de ces aspects critiques des réseaux neuronaux. Ces chapitres couvrent les réseaux de fonctions de base radiales (RBF), les machines limitées de Boltzmann (RBM) et d'autres sujets avancés. Thèmes avancés s sept et huit chapitres traitent de sujets plus avancés, tels que les réseaux neuronaux récurrents (RNN), les réseaux neuronaux convolutifs (CNN) et l'apprentissage profond renforcé. Ces chapitres présentent également les cartes auto-organisées de Kohonen, les réseaux de compétition générative (GAN) et d'autres meilleures pratiques. Applications des réseaux neuronaux s chapitres 5 et 6 présentent les applications pratiques des réseaux neuronaux dans divers domaines, y compris les sous-titres d'images, la classification d'images, la formation en renforcement et l'analyse de texte.
Redes neuronales y aprendizaje profundo: Un libro de texto A medida que continuamos evolucionando en nuestros avances tecnológicos, es esencial que comprendamos el proceso de desarrollo del conocimiento moderno y su impacto en la supervivencia de la humanidad. Este tutorial ofrece una visión general completa de los modelos clásicos y modernos en el aprendizaje profundo, con énfasis en la teoría y algoritmos de las redes neuronales y sus aplicaciones en diferentes campos. Fundamentos de las redes neuronales capítulos primero y segundo profundizan en los fundamentos de las redes neuronales, investigando cómo los modelos tradicionales de aprendizaje automático pueden entenderse como casos privados de redes neuronales. Estos capítulos abarcan máquinas vectoriales auxiliares, regresión logística lineal, descomposición de valores singulares, descomposición de matrices en multiplicadores y sistemas de recomendación, proporcionando una base sólida para entender la relación entre el aprendizaje automático tradicional y las redes neuronales. Además, se discuten los últimos métodos de diseño de elementos, como word2vec. Aprendizaje y regularización capítulos tercero y cuarto profundizan en los métodos de aprendizaje y regularización, ofreciendo una discusión detallada de estos aspectos críticos de las redes neuronales. Estos capítulos cubren redes de funciones básicas radiales (RBF), máquinas limitadas de Boltzmann (RBM) y otros temas avanzados. Temas avanzados En los capítulos siete y ocho se abordan temas más avanzados como redes neuronales recurrativas (RNN), redes neuronales perforadas (CNN) y aprendizaje profundo con refuerzos. Estos capítulos también presentan mapas auto-organizados de Cohonen, Redes de Competencia Generativa (GAN) y otras técnicas avanzadas. Aplicaciones de redes neuronales capítulos cinco y seis muestran las aplicaciones prácticas de las redes neuronales en diferentes áreas, incluyendo subtítulos de imágenes, clasificación de imágenes, entrenamiento de refuerzos y análisis de texto.
Redes neurais e treinamento profundo: Tutorial À medida que continuamos evoluindo em nossos avanços tecnológicos, é fundamental que compreendamos o processo de desenvolvimento do conhecimento moderno e seus efeitos na sobrevivência humana. Este currículo apresenta uma revisão abrangente dos modelos clássicos e modernos no aprendizado profundo, com foco na teoria e algoritmos das redes neurais e suas aplicações em diferentes áreas. Os fundamentos das redes neurais do capítulo um e dois se aprofundam nas redes neurais, explorando como os modelos tradicionais de aprendizagem de máquinas podem ser entendidos como casos privados de redes neurais. Estes capítulos abrangem máquinas vetoriais auxiliares, regressão logística linear, decomposição de valores singulares, decomposição de matrizes em multiplicadores e sistemas de recomendação, fornecendo uma base sólida para compreender a relação entre o aprendizado tradicional das máquinas e as redes neurais. Além disso, os últimos métodos de construção de elementos, como word2vec, estão sendo discutidos. A formação e regularização dos capítulos Terceiro e Quarto se aprofundam nos métodos de aprendizagem e regularização, oferecendo um debate detalhado sobre esses aspectos críticos das redes neurais. Estes capítulos abrangem redes de funções de base radiais (RBF), máquinas limitadas de Bolzmann (RBM) e outros temas avançados. Temas ampliados Em sete e oito capítulos são abordados temas mais avançados, como redes neurais recorrentes (RNN), redes neurais personalizadas (CNN) e treinamento profundo com reforços. Estes capítulos também apresentam mapas auto-organizados de Kohonen, redes genéricas de competição (GAN) e outras técnicas avançadas. Os aplicativos da rede neural Capítulo Cinco e Seis mostram aplicações práticas de redes neurais em várias áreas, incluindo legendas de imagem, classificação de imagens, treinamento de reforços e análise de texto.
Neuronale Netze und Deep arning: hrbuch Während wir uns in unseren technologischen Fortschritten weiterentwickeln, ist es unerlässlich, dass wir den Entwicklungsprozess des modernen Wissens und seine Auswirkungen auf das Überleben der Menschheit verstehen. Dieses Tutorial bietet einen umfassenden Überblick über sowohl klassische als auch moderne Modelle im Deep arning mit Schwerpunkt auf Theorie und Algorithmen neuronaler Netze und deren Anwendungen in verschiedenen Bereichen. Grundlagen neuronaler Netze Kapitel eins und zwei vertiefen sich in die Grundlagen neuronaler Netze und untersuchen, wie traditionelle Modelle des maschinellen rnens als Sonderfälle neuronaler Netze verstanden werden können. Diese Kapitel behandeln Hilfsvektormaschinen, lineare logistische Regression, singuläre Wertzerlegung, Matrixfaktorzerlegung und Empfehlungssysteme und bieten eine solide Grundlage für das Verständnis der Beziehung zwischen traditionellem maschinellem rnen und neuronalen Netzwerken. Darüber hinaus werden die neuesten Konstruktionsmethoden für Elemente wie word2vec diskutiert. Training und Regularisierung Die Kapitel drei und vier vertiefen die rn- und Regularisierungstechniken und bieten eine detaillierte Diskussion über diese kritischen Aspekte neuronaler Netzwerke. Diese Kapitel decken Netzwerke von radialen Basisfunktionen (RBF), Boltzmann Limited Machines (RBM) und andere fortgeschrittene Themen ab. Erweiterte Themen In den Kapiteln sieben und acht werden fortgeschrittenere Themen wie Recurrent Neural Networks (RNNs), Convolutional Neural Networks (CNNs) und Deep arning mit Verstärkung behandelt. Diese Kapitel enthalten auch selbstorganisierende Kohonen-Karten, generative Contracting Networks (GANs) und andere fortgeschrittene Techniken. Die Kapitel fünf und sechs der neuronalen Netzanwendungen zeigen praktische Anwendungen von neuronalen Netzen in einer Vielzahl von Bereichen, einschließlich Bilduntertiteln, Bildklassifizierung, Verstärkungstraining und Textanalyse.
''
nir Ağları ve Derin Öğrenme: Bir Ders Kitabı Teknolojik gelişmelerimizde gelişmeye devam ederken, modern bilginin gelişim sürecini ve bunun insanın hayatta kalması üzerindeki etkisini anlamamız şarttır. Bu ders kitabı, derin öğrenmede hem klasik hem de modern modellere kapsamlı bir genel bakış sunmakta, sinir ağlarının teorisi ve algoritmalarına ve çeşitli alanlardaki uygulamalarına odaklanmaktadır. nir Ağlarının Temelleri Birinci ve ikinci bölümler, geleneksel makine öğrenme modellerinin sinir ağlarının özel durumları olarak nasıl anlaşılabileceğini araştırarak sinir ağlarının temellerini inceler. Bu bölümler, yardımcı vektör makineleri, doğrusal lojistik regresyon, tekil değer ayrıştırma, matris faktörizasyonu ve önerici sistemleri kapsar ve geleneksel makine öğrenimi ile sinir ağları arasındaki ilişkiyi anlamak için sağlam bir temel sağlar. Ayrıca, word2vec gibi son özellik tasarım teknikleri tartışılmaktadır. Öğrenme ve Düzenlenme Üçüncü ve dördüncü bölümler, öğrenme ve düzenlenme yöntemlerini inceler ve sinir ağlarının bu kritik yönleri hakkında ayrıntılı bir tartışma sunar. Bu bölümler radyal temel fonksiyon (RBF) ağlarını, sınırlı Boltzmann makinelerini (RBM'ler) ve diğer ileri konuları kapsar. Genişletilmiş Konular Yedinci ve sekizinci bölümler, tekrarlayan sinir ağları (RNN'ler), evrişimli sinir ağları (CNN'ler) ve derin pekiştirmeli öğrenme gibi daha ileri konuları kapsar. Bu bölümler aynı zamanda Kohonen'in kendi kendini organize eden haritalarını, üretici düşman ağlarını (GAN'lar) ve diğer en iyi uygulamaları da sunmaktadır. nir Ağı Uygulamaları Beşinci ve altıncı bölümler, resim altyazıları, görüntü sınıflandırması, takviye eğitimi ve metin analizi dahil olmak üzere çeşitli alanlarda sinir ağlarının pratik uygulamalarını göstermektedir.
الشبكات العصبية والتعلم العميق: كتاب مدرسي بينما نستمر في التطور في تقدمنا التكنولوجي، من الضروري أن نفهم عملية تطوير المعرفة الحديثة وتأثيرها على بقاء الإنسان. يقدم هذا الكتاب المدرسي نظرة عامة شاملة لكل من النماذج الكلاسيكية والحديثة في التعلم العميق، مع التركيز على نظرية وخوارزميات الشبكات العصبية وتطبيقاتها في مختلف المجالات. تتعمق أساسيات الشبكات العصبية في الفصلين الأول والثاني في أساسيات الشبكات العصبية، وتستكشف كيف يمكن فهم نماذج التعلم الآلي التقليدية على أنها حالات خاصة للشبكات العصبية. تغطي هذه الفصول آلات المتجهات المساعدة، والانحدار اللوجستي الخطي، وتحلل القيمة الفريدة، وعامل المصفوفة، وأنظمة التوصية، مما يوفر أساسًا صلبًا لفهم العلاقة بين التعلم الآلي التقليدي والشبكات العصبية. بالإضافة إلى ذلك، تمت مناقشة تقنيات تصميم الميزات الحديثة مثل word2vec. يتعمق الفصلان الثالث والرابع من التعلم والتسوية في طرق التعلم والتسوية، مما يوفر مناقشة مفصلة لهذه الجوانب الحرجة للشبكات العصبية. تغطي هذه الفصول شبكات دالة الأساس الشعاعي (RBF) وآلات بولتزمان المحدودة (RBMs) وغيرها من الموضوعات المتقدمة. تغطي الفصلان السابع والثامن من الموضوعات الممتدة موضوعات أكثر تقدمًا مثل الشبكات العصبية المتكررة (RNNs) والشبكات العصبية التلافيفية (CNNs) والتعلم المعزز العميق. تقدم هذه الفصول أيضًا خرائط Kohonen ذاتية التنظيم وشبكات الخصومة التوليدية (GANs) وأفضل الممارسات الأخرى. يوضح الفصلان الخامس والسادس من تطبيقات الشبكة العصبية التطبيقات العملية للشبكات العصبية في مجالات مختلفة، بما في ذلك ترجمة الصور وتصنيف الصور والتدريب على التعزيز وتحليلات النصوص.
