
BOOKS - Explainable AI: Interpreting, Explaining and Visualizing Deep Learning (Lectu...

Explainable AI: Interpreting, Explaining and Visualizing Deep Learning (Lecture Notes in Computer Science Book 11700)
Author: Wojciech Samek
Year: September 10, 2019
Format: PDF
File size: PDF 94 MB
Language: English
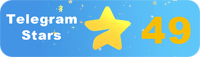
Year: September 10, 2019
Format: PDF
File size: PDF 94 MB
Language: English
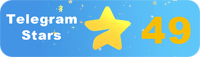
Book Explainable AI Interpreting Explaining and Visualizing Deep Learning Lecture Notes in Computer Science Book 11700 Introduction: Artificial Intelligence (AI) has come a long way since its inception, and with each passing day, it continues to evolve and advance at an unprecedented rate. The development of intelligent systems that can take decisions and perform autonomously has the potential to revolutionize various industries and aspects of our lives. However, the inherent risks associated with giving up human control and oversight to these machines pose a significant limiting factor for their broader adoption. To mitigate these risks, it is crucial to validate the behavior of AI systems before deploying them in real-world environments. This requires developing ways for humans to verify the agreement between the AI decision structure and their own ground truth knowledge. The subfield of AI known as Explainable AI (XAI) has emerged as a solution to this problem. XAI focuses on exposing complex AI models to humans in a systematic and interpretable manner, enabling us to understand the reasoning behind their decisions. In this book, we explore the current state of XAI, including its theory, algorithms, and applications. The chapters are organized into six sections, each addressing a critical aspect of AI transparency, explaining AI systems, evaluating interpretability, and providing examples of explainable AI software. Section 1: AI Transparency Methods Chapter 1 delves into the various methods for interpreting AI systems, including feature attribution, model interpretability, and model-agnostic explanations.
Book Explainable AI Interpreting Explaining and Visualizing Deep arning cture Notes in Computer Science Book 11700 Introduction: Artificial Intelligence (AI) прошел долгий путь с момента своего создания, и с каждым днем продолжает развиваться и продвигаться с беспрецедентной скоростью. Развитие интеллектуальных систем, которые могут принимать решения и работать автономно, может революционизировать различные отрасли и аспекты нашей жизни. Тем не менее, неотъемлемые риски, связанные с отказом от человеческого контроля и надзора за этими машинами, представляют собой значительный ограничивающий фактор для их более широкого применения. Для снижения этих рисков крайне важно проверить поведение систем искусственного интеллекта перед их развертыванием в реальных средах. Это требует разработки способов для людей проверить согласие между структурой принятия решений ИИ и их собственным знанием истины. В качестве решения этой проблемы возникло подполе ИИ, известное как Explainable AI (XAI). XAI фокусируется на систематическом и интерпретируемом воздействии сложных моделей ИИ на людей, что позволяет нам понять причины их решений. В этой книге мы исследуем текущее состояние XAI, включая его теорию, алгоритмы и приложения. Главы состоят из шести разделов, в каждом из которых рассматривается критический аспект прозрачности ИИ, объясняются системы ИИ, оценивается интерпретируемость и приводятся примеры объяснимого программного обеспечения ИИ. Раздел 1: Методы прозрачности ИИ Глава 1 углубляется в различные методы интерпретации систем ИИ, включая атрибуцию признаков, интерпретируемость модели и модельно-агностические объяснения.
Book Exploitable AI Interpreting Explaining and Visualizing Deep arning cture Notes in Computer Science Book 11700 Introduction : Intelligence Artificielle (AI) a parcouru un long chemin depuis sa création, et continue d'évoluer et de progresser chaque jour une vitesse sans précédent. développement de systèmes intelligents capables de prendre des décisions et de fonctionner de manière autonome peut révolutionner différents secteurs et aspects de notre vie. Néanmoins, les risques inhérents à l'abandon du contrôle et de la surveillance humains de ces machines constituent un facteur limitatif important pour leur application plus large. Pour réduire ces risques, il est essentiel de vérifier le comportement des systèmes d'intelligence artificielle avant de les déployer dans des environnements réels. Pour cela, il faut mettre au point des méthodes permettant aux individus de vérifier l'accord entre la structure décisionnelle de l'IA et leur propre connaissance de la vérité. Comme solution à ce problème, un lieutenant d'IA, connu sous le nom d'AI Explosible (XAI), est apparu. XAI se concentre sur l'impact systématique et interprétable des modèles complexes d'IA sur les personnes, ce qui nous permet de comprendre les raisons de leurs décisions. Dans ce livre, nous examinons l'état actuel de XAI, y compris sa théorie, ses algorithmes et ses applications. s chapitres se composent de six sections, chacune traitant de l'aspect critique de la transparence de l'IA, expliquant les systèmes d'IA, évaluant l'interprétabilité et donnant des exemples de logiciels d'IA explicables. Section 1 : Méthodes de transparence de l'IA chapitre 1 explore diverses méthodes d'interprétation des systèmes d'IA, y compris l'attribution des caractéristiques, l'interprétabilité du modèle et les explications modélo-agnostiques.
Book Explainable AI Interpreting Exploring and Visualizing Deep Arning Cture Notes in Computer Science Book 11700 Introduction: Artificial Intelligence (AI) ha pasado mucho tiempo el camino desde sus inicios, y cada día sigue evolucionando y avanzando a una velocidad sin precedentes. desarrollo de sistemas inteligentes que puedan tomar decisiones y operar de manera autónoma puede revolucionar diferentes industrias y aspectos de nuestras vidas. n embargo, los riesgos inherentes de abandonar el control y la supervisión humanos de estas máquinas constituyen un importante factor limitante para su aplicación más amplia. Para reducir estos riesgos, es fundamental comprobar el comportamiento de los sistemas de inteligencia artificial antes de implementarlos en entornos reales. Esto requiere desarrollar formas para que la gente compruebe el acuerdo entre la estructura de toma de decisiones de IA y su propio conocimiento de la verdad. Como solución a este problema, surgió un podpolo de IA conocido como Explainable AI (XAI). XAI se centra en el impacto sistemático e interpretable de modelos complejos de IA en las personas, lo que nos permite entender las razones de sus decisiones. En este libro investigamos el estado actual de XAI, incluyendo su teoría, algoritmos y aplicaciones. capítulos constan de seis secciones, en cada una de las cuales se aborda el aspecto crítico de la transparencia de la IA, se explican los sistemas de IA, se evalúa la interpretabilidad y se dan ejemplos de software de IA explicable. Sección 1: Métodos de transparencia de la IA capítulo 1 profundiza en los diferentes métodos de interpretación de los sistemas de IA, incluyendo atribución de rasgos, interpretabilidad del modelo y explicaciones modelo-agnósticas.
Book Explorable AI Interpreting Exploring and Visualizing Deep arning Notes in Machine Science Book 11700 Inteligência (AI) percorreu um longo caminho desde a sua criação e, a cada dia, continua a desenvolver-se e a avançar a uma velocidade sem precedentes. O desenvolvimento de sistemas inteligentes que possam tomar decisões e funcionar de forma autônoma pode revolucionar vários setores e aspectos das nossas vidas. No entanto, os riscos inerentes associados à rejeição do controle humano e da supervisão dessas máquinas representam uma limitação significativa para o seu uso mais amplo. Para reduzir esses riscos, é crucial verificar o comportamento dos sistemas de inteligência artificial antes de serem implantados em ambientes reais. Isso requer o desenvolvimento de formas para as pessoas testarem o consenso entre a estrutura de decisão da IA e o seu próprio conhecimento da verdade. O subproduto da IA, conhecido como Explainable AI (XAI), surgiu como solução para este problema. XAI se concentra nos efeitos sistemáticos e interpretáveis de modelos complexos de IA sobre as pessoas, o que nos permite compreender as razões de suas decisões. Neste livro, investigamos o estado atual do XAI, incluindo sua teoria, algoritmos e aplicativos. Os capítulos constam de seis seções que abordam o aspecto crítico da transparência da IA, explicam os sistemas de IA, avaliam a interpretabilidade e dão exemplos de software de IA explicável. Secção 1: Os métodos de transparência da IA Capítulo 1 aprofundam-se em várias técnicas de interpretação de sistemas de IA, incluindo atribuição de sinais, interpretação do modelo e explicações modélicas e agnósticas.
Book Explainable AI Intraining Explaining and Visalizing Deep arning cute Note in Computer Science Book 11700 Intelligence (AI) ha fatto molta strada fin dalla sua creazione, e continua a svilupparsi e ad avanzare ogni giorno ad una velocità senza precedenti. Lo sviluppo di sistemi intelligenti in grado di prendere decisioni e operare autonomamente può rivoluzionare diversi settori e aspetti della nostra vita. Tuttavia, i rischi inerenti all'abbandono del controllo umano e della supervisione di queste macchine costituiscono un fattore limitante significativo per la loro maggiore applicazione. Per ridurre questi rischi, è fondamentale verificare il comportamento dei sistemi di intelligenza artificiale prima di installarli in ambienti reali. Ciò richiede lo sviluppo di modi per le persone di verificare il consenso tra la struttura decisionale dell'IA e la loro conoscenza della verità. Per risolvere questo problema è emerso un sottopoli di IA noto come Esplainable AI (XAI). XAI si concentra sull'impatto sistematico e interpretabile di modelli di IA complessi sulle persone, che ci permette di comprendere le ragioni delle loro decisioni. In questo libro stiamo esaminando lo stato attuale di XAI, inclusa la sua teoria, algoritmi e applicazioni. I capitoli sono costituiti da sei sezioni, ognuna delle quali affronta l'aspetto critico della trasparenza dell'IA, spiega i sistemi di IA, valuta l'interpretabilità e fornisce esempi di software IA spiegabili. Sezione 1: Metodi di trasparenza dell'IA Il capitolo 1 approfondisce i vari metodi di interpretazione dei sistemi IA, tra cui l'attribuzione dei segni, l'interpretabilità del modello e le spiegazioni modico-agnostiche.
Book Explainable AI Interpreting Explaining and Visualizing Deep arning cture Notes in Computer Science Book 11700 Einführung: Künstliche Intelligenz (KI) hat seit ihrer Gründung einen langen Weg zurückgelegt und entwickelt sich täglich weiter und schreitet mit beispielloser Geschwindigkeit voran. Die Entwicklung intelligenter Systeme, die Entscheidungen treffen und autonom arbeiten können, kann verschiedene Branchen und Aspekte unseres bens revolutionieren. Die inhärenten Risiken, die mit der Aufgabe der menschlichen Kontrolle und Überwachung dieser Maschinen verbunden sind, stellen jedoch einen erheblichen limitierenden Faktor für ihre breitere Anwendung dar. Um diese Risiken zu reduzieren, ist es entscheidend, das Verhalten von KI-Systemen zu überprüfen, bevor sie in realen Umgebungen eingesetzt werden. Dies erfordert die Entwicklung von Möglichkeiten für Menschen, die Übereinstimmung zwischen der Entscheidungsstruktur der KI und ihrem eigenen Wissen über die Wahrheit zu überprüfen. Als Lösung für dieses Problem ist ein KI-Teilfeld entstanden, das als Explainable AI (XAI) bekannt ist. XAI konzentriert sich auf die systematischen und interpretierten Auswirkungen komplexer KI-Modelle auf den Menschen und ermöglicht es uns, die Gründe für ihre Entscheidungen zu verstehen. In diesem Buch untersuchen wir den aktuellen Zustand von XAI, einschließlich seiner Theorie, Algorithmen und Anwendungen. Die Kapitel bestehen aus sechs Abschnitten, in denen jeweils der kritische Aspekt der KI-Transparenz behandelt, KI-Systeme erklärt, die Interpretierbarkeit bewertet und Beispiele für erklärbare KI-Software gegeben werden. Abschnitt 1: Methoden der KI-Transparenz Kapitel 1 befasst sich mit verschiedenen Methoden zur Interpretation von KI-Systemen, einschließlich der Attribution von Merkmalen, der Interpretierbarkeit des Modells und modellagnostischen Erklärungen.
Book Explainable AI Interpretacja Wyjaśnianie i wizualizacja Głębokie arning cture Notes in Computer Science Book 11700 Wprowadzenie: Sztuczna inteligencja (AI) przeszła długą drogę od jej powstania, i nadal ewoluować i postępować z bezprecedensową prędkością każdego dnia. Rozwój inteligentnych systemów, które mogą podejmować decyzje i samodzielnie pracować, może zrewolucjonizować różne branże i aspekty naszego życia. Jednakże nieodłączne ryzyko braku kontroli i nadzoru nad tymi maszynami stanowią istotny czynnik ograniczający ich szersze zastosowanie. Aby złagodzić te zagrożenia, konieczne jest sprawdzenie zachowania systemów AI przed ich wdrożeniem w środowiskach realnych. Wymaga to opracowania sposobów sprawdzania porozumienia pomiędzy strukturą decyzyjną AI a ich własną znajomością prawdy. Jako rozwiązanie tego problemu pojawiło się podfield AI znany jako Explainable AI (XAI). XAI koncentruje się na systematycznym i możliwym do interpretacji wpływie złożonych modeli grypy ptaków na ludzi, co pozwala nam zrozumieć przyczyny ich decyzji. W tej książce badamy aktualny stan XAI, w tym jego teorię, algorytmy i aplikacje. Rozdziały składają się z sześciu sekcji, z których każda zajmuje się krytycznym aspektem przejrzystości grypy ptaków, wyjaśniając systemy sztucznej inteligencji, oceniając interpretację i dostarczając przykłady zrozumiałego oprogramowania sztucznej inteligencji. Sekcja 1: AI Transparency Methods Rozdział 1 obejmuje różne metody interpretacji systemów AI, w tym przypisywanie funkcji, interpretację modelu oraz wyjaśnienia agnostyczne.
Book Explainable AI Interpreting and Visualing Deep Arning Coture Notes in Computer Science Book 11700 Introduction: AI) עבר דרך ארוכה מאז הקמתה, וממשיך להתפתח ולהתקדם במהירות במהירות חסרת תקדים מדי יום. פיתוח מערכות תבוניות שיכולות לקבל החלטות ולעבוד באופן עצמאי עלול לחולל מהפכה בתעשיות ובהיבטים שונים של חיינו. עם זאת, הסיכונים הטבועים באי שליטה ופיקוח אנושיים על מכונות אלה מהווים גורם מגביל משמעותי ליישום רחב יותר שלהן. כדי למתן סיכונים אלה, זה קריטי לבחון את ההתנהגות של מערכות בינה מלאכותית לפני פריסתן בסביבות העולם האמיתי. הדבר מצריך פיתוח דרכים לאנשים לבחון את ההסכם בין מבנה קבלת ההחלטות של אל לבין הידע שלהם על האמת. כפתרון לבעיה זו, נוצר תת-שדה AI הידוע בשם Explainable AI (XAI). XAI מתמקד בהשפעות השיטתיות והמתורגמות של מודלים מורכבים של בינה מלאכותית על בני אדם, ומאפשר לנו להבין את הסיבות מאחורי החלטותיהם. בספר זה, אנו חוקרים את המצב הנוכחי של XAI, כולל התאוריה שלו, אלגוריתמים, ויישומים. הפרקים מורכבים משישה חלקים, שכל אחד מהם מתייחס להיבט קריטי של שקיפות בינה מלאכותית, מסביר מערכות בינה מלאכותית, מעריך יכולות פרשנות, ומספק דוגמאות של תוכנות בינה מלאכותית מוסברות. סעיף 1: שיטות שקיפות AI פרק 1 מתעמק בשיטות שונות לפירוש מערכות AI, כולל ייחוס תכונה, פרשנות מודל והסברים מודלים-אגנוסטיים.''
Derin Öğrenmeyi Açıklayan ve Görselleştiren Açıklanabilir AI Kitabı Bilgisayar Bilimlerinde Ders Notları Kitap 11700 Giriş: Yapay Zeka (AI) kuruluşundan bu yana uzun bir yol kat etti ve her gün benzeri görülmemiş bir hızla gelişmeye ve ilerlemeye devam ediyor. Karar verebilen ve özerk olarak çalışabilen akıllı sistemlerin geliştirilmesi, çeşitli endüstrilerde ve hayatımızın yönlerinde devrim yaratabilir. Bununla birlikte, bu makineler üzerinde insan kontrolü ve denetimine sahip olmamanın doğal riskleri, daha geniş uygulamaları için önemli bir sınırlayıcı faktördür. Bu riskleri azaltmak için, AI sistemlerinin davranışlarını gerçek dünya ortamlarına dağıtmadan önce test etmek çok önemlidir. Bu, insanların AI'nın karar verme yapısı ile kendi gerçek bilgileri arasındaki anlaşmayı test etmeleri için yollar geliştirmeyi gerektirir. Bu soruna bir çözüm olarak, açıklanabilir AI (XAI) olarak bilinen bir AI alt alanı ortaya çıktı. XAI, karmaşık AI modellerinin insanlar üzerindeki sistematik ve yorumlanabilir etkilerine odaklanır ve kararlarının arkasındaki nedenleri anlamamızı sağlar. Bu kitapta, teorisi, algoritmaları ve uygulamaları da dahil olmak üzere XAI'ın mevcut durumunu araştırıyoruz. Bölümler, her biri AI şeffaflığının kritik bir yönünü ele alan, AI sistemlerini açıklayan, yorumlanabilirliği değerlendiren ve açıklanabilir AI yazılımı örnekleri sunan altı bölümden oluşmaktadır. Bölüm 1: AI Şeffaflık Yöntemleri Bölüm 1, özellik ilişkilendirme, model yorumlanabilirliği ve model-agnostik açıklamalar dahil olmak üzere AI sistemlerini yorumlamanın çeşitli yöntemlerini inceler.
كتاب | تفسير الذكاء الاصطناعي القابل للتفسير شرح وتصور ملاحظات محاضرة التعلم العميق في كتاب علوم الكمبيوتر 11700 المقدمة: لقد قطع الذكاء الاصطناعي (AI) شوطًا طويلاً منذ إنشائه، ويستمر في التطور والتقدم بسرعة غير مسبوقة كل يوم. إن تطوير أنظمة ذكية يمكنها اتخاذ القرارات والعمل بشكل مستقل يمكن أن يحدث ثورة في مختلف الصناعات وجوانب حياتنا. ومع ذلك، فإن المخاطر الكامنة في عدم وجود سيطرة بشرية وإشراف على هذه الآلات تمثل عاملاً مقيدًا كبيرًا لتطبيقها على نطاق أوسع. للتخفيف من هذه المخاطر، من الأهمية بمكان اختبار سلوك أنظمة الذكاء الاصطناعي قبل نشرها في بيئات العالم الحقيقي. يتطلب هذا تطوير طرق للناس لاختبار الاتفاقية بين هيكل صنع القرار في الذكاء الاصطناعي ومعرفتهم الخاصة بالحقيقة. كحل لهذه المشكلة، ظهر مجال فرعي للذكاء الاصطناعي يُعرف باسم Explainable AI (XAI). يركز XAI على الآثار المنهجية والقابلة للتفسير لنماذج الذكاء الاصطناعي المعقدة على البشر، مما يسمح لنا بفهم الأسباب الكامنة وراء قراراتهم. في هذا الكتاب، نستكشف الحالة الحالية لـ XAI، بما في ذلك نظريته وخوارزمياته وتطبيقاته. تتكون الفصول من ستة أقسام، يتناول كل منها جانبًا مهمًا من شفافية الذكاء الاصطناعي، ويشرح أنظمة الذكاء الاصطناعي، ويقيم قابلية التفسير، ويقدم أمثلة على برامج الذكاء الاصطناعي القابلة للتفسير. القسم 1: أساليب شفافية الذكاء الاصطناعي يتعمق الفصل 1 في طرق مختلفة لتفسير أنظمة الذكاء الاصطناعي، بما في ذلك إسناد الميزة، وإمكانية تفسير النموذج، والتفسيرات اللاأدرية النموذجية.
書籍解釋性AI解釋和可視化深層保護註釋在計算機科學書籍11700介紹:人工智能(AI)自成立以來已經走了很長一段路,並且每天都在不斷發展和進步以前所未有的速度。發展能夠自主決策和運作的智能系統可以徹底改變我們生活的不同行業和方面。然而,放棄對這些機器的人力控制和監督所帶來的固有風險構成了更廣泛使用這些機器的重大限制因素。為了降低這些風險,在人工智能系統部署到現實世界環境中之前檢查其行為至關重要。這需要開發人們測試AI決策結構與自己對真理的了解之間的一致性的方法。作為解決此問題的方法,出現了一個稱為Explainable AI(XAI)的AI子領域。XAI專註於復雜的AI模型對人類的系統和解釋性影響,使我們能夠了解其決策的原因。在本書中,我們探討了XAI的當前狀態,包括其理論,算法和應用。本章分為六個部分,每個部分都討論人工智能透明度的關鍵方面,解釋人工智能系統,評估可解釋性,並提供可解釋人工智能軟件的示例。第1節:AI透明度方法第一章深入研究了AI系統的不同解釋方法,包括特征歸屬,模型解釋和模態不可知論解釋。
