
BOOKS - PROGRAMMING - Observability for Large Language Models Understanding and Impro...

Observability for Large Language Models Understanding and Improving Your Use of LLMs
Author: Phillip Carter
Year: 2023-09-28
Format: PDF | MOBI | EPUB
File size: 10.2 MB
Language: ENG
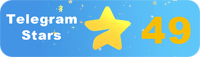
Year: 2023-09-28
Format: PDF | MOBI | EPUB
File size: 10.2 MB
Language: ENG
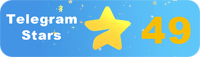
''
