
BOOKS - MLOps with Ray Best Practices and Strategies for Adopting Machine Learning Op...

MLOps with Ray Best Practices and Strategies for Adopting Machine Learning Operations
Author: Hien Luu, Max Pumperla, Zhe Zhang
Year: 2024
Pages: 342
Format: PDF | EPUB
File size: 11.8 MB
Language: ENG
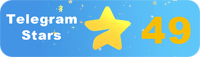
Year: 2024
Pages: 342
Format: PDF | EPUB
File size: 11.8 MB
Language: ENG
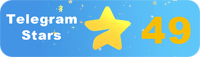
The book "MLOps with Ray Best Practices and Strategies for Adopting Machine Learning Operations" provides a comprehensive guide to implementing machine learning operations (MLOps) in organizations, focusing on the use of Ray, an open-source framework for building, deploying, and managing machine learning models. The book covers the entire lifecycle of MLOps, from data preparation and model training to deployment and maintenance, and offers practical strategies for overcoming common challenges and obstacles. The book begins by highlighting the importance of understanding the technology evolution process and its impact on society. As technology continues to advance at an unprecedented pace, it is essential to develop a personal paradigm for perceiving the technological process of developing modern knowledge. This involves recognizing the interconnectedness of technology and its role in shaping our worldview, values, and beliefs. By doing so, we can better understand the implications of technology on humanity and make informed decisions about its adoption and application. The book then delves into the concept of MLOps and its significance in the field of machine learning. MLOps refers to the practice of integrating machine learning into DevOps processes, enabling organizations to streamline their workflows and improve the efficiency of their machine learning projects. With the increasing demand for AI and machine learning applications, MLOps has become a crucial aspect of software development, particularly in industries such as healthcare, finance, and marketing. The authors emphasize the need for a holistic approach to MLOps, considering factors such as data management, model training, deployment, and monitoring. They provide practical strategies for each stage of the MLOps lifecycle, including data preparation, model selection, training and validation, deployment, and maintenance.
В книге «MLOps with Ray Best Practices and Strategies for Adopting Machine arning Operations» представлено всеобъемлющее руководство по внедрению операций машинного обучения (MLOps) в организациях с акцентом на использование Ray, инфраструктуры с открытым исходным кодом для построения, развертывания и управления моделями машинного обучения. Книга охватывает весь жизненный цикл MLOps, от подготовки данных и обучения модели до развертывания и обслуживания, и предлагает практические стратегии для преодоления общих проблем и препятствий. Книга начинается с того, что подчеркивается важность понимания процесса эволюции технологий и его влияния на общество. Поскольку технологии продолжают развиваться беспрецедентными темпами, важно разработать личную парадигму восприятия технологического процесса развития современных знаний. Это предполагает признание взаимосвязанности технологий и их роли в формировании нашего мировоззрения, ценностей и убеждений. Тем самым мы сможем лучше понять последствия технологии для человечества и принять обоснованные решения о ее принятии и применении. Затем книга углубляется в понятие MLOps и его значение в области машинного обучения. MLOps относится к практике интеграции машинного обучения в процессы DevOps, что позволяет организациям оптимизировать свои рабочие процессы и повысить эффективность своих проектов в области машинного обучения. С ростом спроса на приложения для искусственного интеллекта и машинного обучения MLOps стал важнейшим аспектом разработки программного обеспечения, особенно в таких отраслях, как здравоохранение, финансы и маркетинг. Авторы подчеркивают необходимость целостного подхода к MLOps с учетом таких факторов, как управление данными, обучение моделей, развертывание и мониторинг. Они предоставляют практические стратегии для каждого этапа жизненного цикла MLOps, включая подготовку данных, выбор модели, обучение и проверку, развертывание и обслуживание.
livre « MLOps with Ray Best Practices and Strategies for Adapting Machine Arning Operations » présente un guide complet sur la mise en œuvre des opérations d'apprentissage automatique (MLOps) dans les organisations, en mettant l'accent sur l'utilisation de Ray, l'infrastructure open source pour la construction, et gestion des modèles d'apprentissage automatique. livre couvre tout le cycle de vie des MLOps, de la préparation des données et de l'apprentissage du modèle au déploiement et à la maintenance, et propose des stratégies pratiques pour surmonter les défis et les obstacles communs. livre commence par souligner l'importance de comprendre l'évolution de la technologie et son impact sur la société. Alors que la technologie continue d'évoluer à un rythme sans précédent, il est important d'élaborer un paradigme personnel pour la perception du processus technologique du développement des connaissances modernes. Cela implique de reconnaître l'interdépendance des technologies et leur rôle dans la formation de notre vision du monde, de nos valeurs et de nos croyances. Nous pourrons ainsi mieux comprendre les conséquences de la technologie pour l'humanité et prendre des décisions éclairées sur son adoption et son application. livre est ensuite approfondi dans la notion de MLOps et son importance dans le domaine de l'apprentissage automatique. MLOps se réfère à la pratique d'intégration de l'apprentissage automatique dans les processus DevOps, ce qui permet aux organisations d'optimiser leurs flux de travail et d'améliorer l'efficacité de leurs projets d'apprentissage automatique. Avec la demande croissante d'applications d'intelligence artificielle et d'apprentissage automatique, MLOps est devenu un aspect essentiel du développement de logiciels, en particulier dans les secteurs de la santé, de la finance et du marketing. s auteurs soulignent la nécessité d'une approche globale des MLOps, en tenant compte de facteurs tels que la gestion des données, l'apprentissage des modèles, le déploiement et la surveillance. Ils fournissent des stratégies pratiques pour chaque étape du cycle de vie de MLOps, y compris la préparation des données, le choix du modèle, la formation et la validation, le déploiement et la maintenance.
libro «MLOps with Ray Best Practices and Strategies for Adopting Machine Arning Operations» presenta una guía completa para la implementación de operaciones de aprendizaje automático (MLOps) en organizaciones con énfasis en el uso de Ray, infraestructura con código abierto para construir, implementar y administrar modelos de aprendizaje automático. libro cubre todo el ciclo de vida de MLOps, desde la preparación de datos y la capacitación del modelo hasta la implementación y el mantenimiento, y ofrece estrategias prácticas para superar desafíos y obstáculos comunes. libro comienza subrayando la importancia de comprender el proceso de evolución de la tecnología y su impacto en la sociedad. A medida que la tecnología continúa evolucionando a un ritmo sin precedentes, es importante desarrollar un paradigma personal para percibir el proceso tecnológico del desarrollo del conocimiento moderno. Esto implica reconocer la interrelación de la tecnología y su papel en la formación de nuestra cosmovisión, valores y creencias. De esta manera podremos comprender mejor las consecuencias de la tecnología para la humanidad y tomar decisiones informadas sobre su adopción y aplicación. A continuación, el libro profundiza en el concepto de MLOps y su significado en el campo del aprendizaje automático. MLOps se refiere a la práctica de integrar el aprendizaje automático en los procesos DevOps, lo que permite a las organizaciones optimizar sus flujos de trabajo y mejorar la eficiencia de sus proyectos de aprendizaje automático. Con la creciente demanda de aplicaciones de inteligencia artificial y aprendizaje automático, MLOps se ha convertido en un aspecto crucial del desarrollo de software, especialmente en industrias como la salud, las finanzas y el marketing. autores subrayan la necesidad de un enfoque holístico de los MLOps, teniendo en cuenta factores como la gestión de datos, el aprendizaje de modelos, la implementación y el monitoreo. Proporcionan estrategias prácticas para cada etapa del ciclo de vida de MLOps, incluyendo preparación de datos, selección de modelos, capacitación y validación, implementación y mantenimiento.
MLOs with Ray Best Practices and Strategies for Adopting Machine Arning Operations fornisce una guida completa per l'implementazione delle attività di apprendimento automatico (MLOs) nelle organizzazioni, focalizzata sull'utilizzo di Ray, l'infrastruttura open source per costruire, implementare e gestire modelli di apprendimento automatico Il libro comprende l'intero ciclo di vita di MLOps, dalla preparazione dei dati all'apprendimento del modello fino all'installazione e alla manutenzione, e offre strategie pratiche per superare problemi e ostacoli comuni. Il libro inizia sottolineando l'importanza di comprendere l'evoluzione della tecnologia e il suo impatto sulla società. Poiché la tecnologia continua a crescere a un ritmo senza precedenti, è importante sviluppare un paradigma personale per la percezione del processo tecnologico di sviluppo della conoscenza moderna. Ciò implica il riconoscimento dell'interconnessione della tecnologia e del loro ruolo nella formazione della nostra visione, dei nostri valori e delle nostre convinzioni. In questo modo possiamo comprendere meglio le implicazioni della tecnologia per l'umanità e prendere decisioni giustificate sulla sua adozione e applicazione. Quindi il libro approfondisce il concetto di MLOs e il suo significato nel campo dell'apprendimento automatico. MLOps si riferisce alla pratica di integrazione dell'apprendimento automatico nei processi di DevOps, che consente alle organizzazioni di ottimizzare i propri flussi di lavoro e migliorare l'efficienza dei progetti di apprendimento automatico. Con l'aumento della domanda di applicazioni di intelligenza artificiale e apprendimento automatico, MLOps è diventato un aspetto fondamentale per lo sviluppo di software, soprattutto in settori come la salute, la finanza e il marketing. Gli autori sottolineano la necessità di un approccio olistico a MLOs in base a fattori quali la gestione dei dati, la formazione dei modelli, l'implementazione e il monitoraggio. Offrono strategie pratiche per ogni fase del ciclo di vita di MLOps, inclusa la preparazione dei dati, la scelta del modello, la formazione e la convalida, l'installazione e la manutenzione.
Das Buch „MLOps with Ray Best Practices and Strategies for Adopting Machine Arning Operations“ bietet einen umfassenden itfaden zur Implementierung von Machine arning Operations (MLOps) in Organisationen mit Schwerpunkt auf der Verwendung von Ray, einer Open-Source-Infrastruktur für den Aufbau, die Bereitstellung und das Management von Machine-arning-Modellen. Das Buch deckt den gesamten benszyklus von MLOps ab, von der Datenaufbereitung und Modellschulung bis hin zur Bereitstellung und Wartung, und bietet praktische Strategien zur Überwindung gemeinsamer Herausforderungen und Hindernisse. Das Buch beginnt mit der Betonung der Bedeutung des Verständnisses des technologischen Evolutionsprozesses und seiner Auswirkungen auf die Gesellschaft. Da sich die Technologie in einem beispiellosen Tempo weiterentwickelt, ist es wichtig, ein persönliches Paradigma für die Wahrnehmung des technologischen Prozesses der Entwicklung des modernen Wissens zu entwickeln. Dies beinhaltet die Anerkennung der Vernetzung von Technologien und ihrer Rolle bei der Gestaltung unserer Weltanschauung, Werte und Überzeugungen. Auf diese Weise können wir die Auswirkungen der Technologie auf die Menschheit besser verstehen und fundierte Entscheidungen über ihre Akzeptanz und Anwendung treffen. Das Buch geht dann auf das Konzept von MLOps und seine Bedeutung im Bereich des maschinellen rnens ein. MLOps bezieht sich auf die Praxis, maschinelles rnen in DevOps-Prozesse zu integrieren, was es Unternehmen ermöglicht, ihre Arbeitsabläufe zu optimieren und die Effizienz ihrer Projekte im Bereich des maschinellen rnens zu steigern. Mit der steigenden Nachfrage nach Anwendungen für künstliche Intelligenz und maschinelles rnen ist MLOps zu einem wichtigen Aspekt der Softwareentwicklung geworden, insbesondere in Branchen wie Gesundheitswesen, Finanzen und Marketing. Die Autoren betonen die Notwendigkeit eines ganzheitlichen Ansatzes für MLOps unter Berücksichtigung von Faktoren wie Datenmanagement, Modellschulung, Bereitstellung und Überwachung. e bieten praktische Strategien für jede Phase des MLOps-benszyklus, einschließlich Datenvorbereitung, Modellauswahl, Schulung und Validierung, Bereitstellung und Wartung.
Książka „MLOps with Ray Best Practices and Strategies for Adopting Machine arning Operations” stanowi kompleksowy przewodnik po wdrażaniu operacji uczenia maszynowego (MLOp) w organizacjach skupiających się na wykorzystaniu Raya, infrastruktury open source do budowania, wdrażania i zarządzania modelami uczenia maszynowego. Książka obejmuje cały cykl życia MLOp, od przygotowania danych i szkolenia modelowego po wdrażanie i utrzymanie oraz oferuje praktyczne strategie pokonywania wspólnych wyzwań i przeszkód. Książka zaczyna się od podkreślenia znaczenia zrozumienia procesu ewolucji technologii i jej wpływu na społeczeństwo. Ponieważ technologia nadal rozwija się w bezprecedensowym tempie, ważne jest opracowanie osobistego paradygmatu postrzegania technologicznego procesu rozwoju nowoczesnej wiedzy. Polega to na uznaniu wzajemnych powiązań technologii i jej roli w kształtowaniu naszego światopoglądu, wartości i przekonań. Dzięki temu możemy lepiej zrozumieć konsekwencje technologii dla ludzkości i podejmować świadome decyzje dotyczące jej przyjęcia i zastosowania. Następnie książka zagłębia się w koncepcję MLOp i jej konsekwencje w dziedzinie uczenia maszynowego. MLOp odnosi się do praktyki integracji uczenia maszynowego z procesami DevOps, co pozwala organizacjom zoptymalizować przepływ pracy i zwiększyć efektywność ich projektów uczenia maszynowego. Wraz z rosnącym zapotrzebowaniem na sztuczną inteligencję i aplikacje do uczenia maszynowego, MLOP stały się krytycznym aspektem rozwoju oprogramowania, zwłaszcza w takich branżach jak opieka zdrowotna, finanse i marketing. Autorzy podkreślają potrzebę całościowego podejścia do MLOP, z uwzględnieniem takich czynników, jak zarządzanie danymi, szkolenie modelowe, wdrażanie i monitorowanie. Zapewniają one praktyczne strategie dla każdego etapu cyklu życia MLOP, w tym przygotowanie danych, dobór modelu, szkolenie i walidację, wdrożenie i utrzymanie.
הספר MLops with Ray Best Practices and Strategies for Emuting Machine Arning Operations מספק מדריך מקיף ליישום פעולות למידת מכונה (MLops) בארגונים המתמקדים בשימוש בריי, תשתית קוד פתוח לבנייה, פריסה וניהול מודלים ללימוד מכונה. הספר מכסה את כל מחזור החיים של MLOPs, החל בהכנת נתונים והכשרת מודלים וכלה בפריסה ותחזוקה, ומציע אסטרטגיות מעשיות כדי להתגבר על אתגרים ומכשולים משותפים. הספר מתחיל בכך שהוא מדגיש את החשיבות של הבנת תהליך האבולוציה של הטכנולוגיה והשפעתה על החברה. כאשר הטכנולוגיה ממשיכה להתפתח בקצב חסר תקדים, חשוב לפתח פרדיגמה אישית לתפיסה של התהליך הטכנולוגי של פיתוח ידע מודרני. הדבר כרוך בהכרה בקישוריות של הטכנולוגיה ובתפקידה בעיצוב השקפת עולמנו, ערכינו ואמונתנו. כך נוכל להבין טוב יותר את ההשלכות של טכנולוגיה לאנושות ולקבל החלטות מושכלות על אימוץ ויישום שלה. לאחר מכן הספר מתעמק במושג MLops ובהשלכותיו בתחום למידת מכונה. MLOps מתייחס לפרקטיקה של שילוב למידת מכונה לתוך תהליכי DevOps, המאפשרים לארגונים לייעל את זרם העבודה שלהם ולהגביר את היעילות של מיזמי למידת המכונה שלהם. עם הביקוש הגובר לבינה מלאכותית ויישומי למידת מכונה, MLOps הפכה להיבט קריטי של פיתוח תוכנה, במיוחד בתעשיות כמו בריאות, פיננסים ושיווק. המחברים מדגישים את הצורך בגישה הוליסטית ל-MLops, תוך לקיחת בחשבון גורמים כגון ניהול נתונים, הדרכת מודלים, פריסה וניטור. הם מספקים אסטרטגיות מעשיות לכל שלב של מחזור החיים של MLOPs, כולל הכנת נתונים, בחירת מודלים, אימון ואימות, פריסה ותחזוקה.''
"MLOps with Ray Best Practices and Strategies for Adopting Machine arning Operations" kitabı, makine öğrenimi modellerini oluşturmak, dağıtmak ve yönetmek için açık kaynaklı bir altyapı olan Ray'i kullanmaya odaklanan kuruluşlarda makine öğrenimi operasyonlarının (MLOps) uygulanması için kapsamlı bir rehber sunmaktadır. Kitap, veri hazırlama ve model eğitiminden dağıtım ve bakıma kadar MLOps'nin tüm yaşam döngüsünü kapsar ve ortak zorlukların ve engellerin üstesinden gelmek için pratik stratejiler sunar. Kitap, teknolojinin evrim sürecini ve toplum üzerindeki etkisini anlamanın önemini vurgulayarak başlıyor. Teknoloji benzeri görülmemiş bir hızda gelişmeye devam ettikçe, modern bilginin geliştirilmesinin teknolojik sürecinin algılanması için kişisel bir paradigma geliştirmek önemlidir. Bu, teknolojinin birbirine bağlılığını ve dünya görüşümüzü, değerlerimizi ve inançlarımızı şekillendirmedeki rolünü kabul etmeyi içerir. Bunu yaparken, teknolojinin insanlık için etkilerini daha iyi anlayabilir ve benimsenmesi ve uygulanması hakkında bilinçli kararlar verebiliriz. Kitap daha sonra MLOps kavramını ve makine öğrenimi alanındaki etkilerini inceliyor. MLOps, makine öğrenimini DevOps süreçlerine entegre etme pratiğini ifade eder; bu, kuruluşların iş akışlarını optimize etmelerini ve makine öğrenimi projelerinin verimliliğini artırmalarını sağlar. Yapay zeka ve makine öğrenimi uygulamalarına olan talebin artmasıyla MLOps, özellikle sağlık, finans ve pazarlama gibi sektörlerde yazılım geliştirmenin kritik bir yönü haline geldi. Yazarlar, veri yönetimi, model eğitimi, dağıtım ve izleme gibi faktörleri dikkate alarak MLOps'a bütünsel bir yaklaşımın gerekliliğini vurgulamaktadır. Veri hazırlama, model seçimi, eğitim ve doğrulama, dağıtım ve bakım dahil olmak üzere MLOps yaşam döngüsünün her aşaması için pratik stratejiler sağlarlar.
يقدم كتاب "MLOps with Ray Best Practices and Strategies for Capting Machine Arching Operations'دليلاً شاملاً لتنفيذ عمليات التعلم الآلي (MLOps) في المؤسسات مع التركيز على استخدام RA، وهي بنية أساسية مفتوحة المصدر لبناء ونشر وإدارة نماذج التعلم الآلة. يغطي الكتاب دورة حياة MLOps بأكملها، من إعداد البيانات والتدريب النموذجي إلى النشر والصيانة، ويقدم استراتيجيات عملية للتغلب على التحديات والعقبات المشتركة. يبدأ الكتاب بالتأكيد على أهمية فهم عملية تطور التكنولوجيا وتأثيرها على المجتمع. مع استمرار تطور التكنولوجيا بوتيرة غير مسبوقة، من المهم تطوير نموذج شخصي لتصور العملية التكنولوجية لتطوير المعرفة الحديثة. وهذا ينطوي على الاعتراف بالترابط بين التكنولوجيا ودورها في تشكيل نظرتنا للعالم وقيمنا ومعتقداتنا. وبذلك، يمكننا أن نفهم بشكل أفضل آثار التكنولوجيا على البشرية وأن نتخذ قرارات مستنيرة بشأن اعتمادها وتطبيقها. ثم يتعمق الكتاب في مفهوم MLOps وآثاره في مجال التعلم الآلي. تشير MLOps إلى ممارسة دمج التعلم الآلي في عمليات DevOps، والتي تسمح للمؤسسات بتحسين سير عملها وزيادة كفاءة مشاريع التعلم الآلي الخاصة بها. مع الطلب المتزايد على تطبيقات الذكاء الاصطناعي والتعلم الآلي، أصبحت MLOps جانبًا مهمًا لتطوير البرامج، خاصة في صناعات مثل الرعاية الصحية والتمويل والتسويق. يؤكد المؤلفون على الحاجة إلى نهج شامل تجاه MLOps، مع مراعاة عوامل مثل إدارة البيانات، والتدريب النموذجي، والنشر، والرصد. توفر استراتيجيات عملية لكل مرحلة من مراحل دورة حياة MLOps، بما في ذلك إعداد البيانات واختيار النموذج والتدريب والتصديق والنشر والصيانة.
「使用雷的最佳實踐和策略進行機器挖掘操作的MLOps」一書為組織中機器學習操作(MLOps)的實施提供了全面的指南,重點是使用Ray,開源基礎架構來構建,部署和管理機器學習模型。該書涵蓋了MLOps的整個生命周期,從數據準備和模型培訓到部署和維護,並提出了克服常見問題和障礙的實用策略。這本書首先強調了了解技術演變過程及其對社會影響的重要性。由於技術繼續以前所未有的速度發展,因此必須制定一個個人範例,以便了解技術進程如何發展現代知識。這意味著承認技術的相互聯系及其在塑造我們的世界觀、價值觀和信仰方面的作用。這樣,我們就能夠更好地了解技術對人類的影響,並就技術的采用和應用作出知情的決定。然後,該書深入研究了MLOps的概念及其在機器學習領域的意義。MLOps是指將機器學習集成到DevOps流程中的實踐,這使組織可以優化其工作流並提高其機器學習項目的效率。隨著對人工智能和機器學習應用程序的需求激增,MLOps已成為軟件開發的重要方面,尤其是在醫療保健,金融和市場營銷等行業。作者強調需要對MLOps采取整體方法,同時考慮到數據管理,模型培訓,部署和監視等因素。它們為MLOps生命周期的每個階段提供實用策略,包括數據準備、模型選擇、培訓和驗證、部署和維護。
