
BOOKS - PROGRAMMING - Implementing MLOps in the Enterprise A Production-First Approac...

Implementing MLOps in the Enterprise A Production-First Approach
Author: Yaron Haviv, Noah Gift
Year: 2024
Pages: 377
Format: EPUB
File size: 15.5 MB
Language: ENG
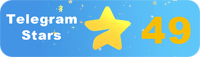
Year: 2024
Pages: 377
Format: EPUB
File size: 15.5 MB
Language: ENG
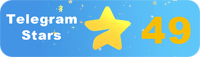
''
