
BOOKS - AI and Data Engineering Solutions for Effective Marketing

AI and Data Engineering Solutions for Effective Marketing
Author: Lhoussaine Alla, Aziz Hmioui, Badr Bentalha
Year: 2024
Pages: 520
Format: PDF | EPUB
File size: 26.4 MB
Language: ENG
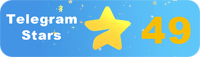
Year: 2024
Pages: 520
Format: PDF | EPUB
File size: 26.4 MB
Language: ENG
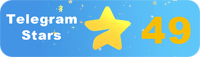
The book "AI and Data Engineering Solutions for Effective Marketing" provides a comprehensive overview of the current state of artificial intelligence (AI) and data engineering solutions in marketing, highlighting their potential benefits and challenges. It covers topics such as machine learning, natural language processing, computer vision, and predictive analytics, and how they can be applied to various marketing strategies. The book also explores the ethical implications of using AI and data engineering in marketing, including privacy concerns and biases. The book begins by discussing the history and development of AI and data engineering, from their early beginnings to the present day. It then delves into the various applications of AI and data engineering in marketing, including personalization, segmentation, and customer profiling. The book also examines the role of AI in content creation, social media monitoring, and influencer marketing. One of the key themes of the book is the need for marketers to understand the technological process of developing modern knowledge and its impact on society. The author argues that this understanding is essential for effective marketing and for ensuring that AI and data engineering are used responsibly and ethically. The book emphasizes the importance of developing a personal paradigm for perceiving the technological process of developing modern knowledge, which can help individuals and organizations navigate the rapidly changing landscape of technology and marketing.
Книга «Решения для искусственного интеллекта и инженерии данных для эффективного маркетинга» содержит всесторонний обзор текущего состояния искусственного интеллекта (ИИ) и решений для инженерии данных в маркетинге, подчеркивая их потенциальные преимущества и проблемы. Он охватывает такие темы, как машинное обучение, обработка естественного языка, компьютерное зрение и предиктивная аналитика, а также то, как их можно применить к различным маркетинговым стратегиям. В книге также рассматриваются этические последствия использования ИИ и инженерии данных в маркетинге, включая проблемы конфиденциальности и предубеждения. Книга начинается с обсуждения истории и развития ИИ и инженерии данных, от их раннего начала до наших дней. Затем он углубляется в различные приложения искусственного интеллекта и инженерии данных в маркетинге, включая персонализацию, сегментацию и профилирование клиентов. В книге также рассматривается роль ИИ в создании контента, мониторинге социальных сетей и маркетинге влияния. Одна из ключевых тем книги - необходимость понимания маркетологами технологического процесса развития современного знания и его влияния на общество. Автор утверждает, что это понимание имеет важное значение для эффективного маркетинга и для обеспечения того, чтобы ИИ и инженерия данных использовались ответственно и этично. В книге подчеркивается важность выработки личностной парадигмы восприятия технологического процесса развития современных знаний, которая может помочь отдельным лицам и организациям ориентироваться в быстро меняющемся ландшафте технологий и маркетинга.
''
