
BOOKS - Machine Learning Approaches in Financial Analytics

Machine Learning Approaches in Financial Analytics
Author: Leandros A. Maglaras, Sonali Das, Naliniprava Tripathy, Srikanta Patnaik
Year: 2024
Pages: 485
Format: PDF | EPUB
File size: 53.1 MB
Language: ENG
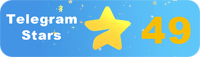
Year: 2024
Pages: 485
Format: PDF | EPUB
File size: 53.1 MB
Language: ENG
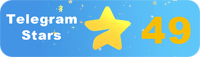
Machine Learning Approaches in Financial Analytics In today's fast-paced and ever-evolving world of finance, the need for comprehensive and practical guides to the application of Machine Learning in financial analytics has become increasingly important. This book addresses this growing need by offering a valuable resource for both beginners and experienced professionals in finance and Data Science. It bridges the gap between theory and practice, providing readers with the tools and knowledge they need to leverage the power of Machine Learning in the financial sector responsibly. The financial world has always been a realm of complexity marked by volatility, uncertainty, and dynamic interconnectedness. Traditional models and tools have often struggled to capture the multifaceted nature of this domain. However, Machine Learning techniques offer a paradigm shift, providing the capability to process vast amounts of data, identify patterns, and generate insights that were previously unimaginable. Throughout the chapters of this book, we explore the fundamental principles of Machine Learning and how they can be applied to tackle a myriad of financial challenges. From predictive modeling, risk assessment, algorithmic trading, portfolio optimization, fraud detection, to customer segmentation, the potential applications are boundless. Object-oriented programming in Python combined with the power of NumPy, Matplotlib, and Jupyter fits the bill perfectly for design and visualization in financial engineering.
Подходы к машинному обучению в финансовой аналитике В современном быстро развивающемся и постоянно развивающемся мире финансов все большее значение приобретает необходимость в комплексных и практических руководствах по применению машинного обучения в финансовой аналитике. Эта книга удовлетворяет эту растущую потребность, предлагая ценный ресурс как для начинающих, так и для опытных специалистов в области финансов и Data Science. Он устраняет разрыв между теорией и практикой, предоставляя читателям инструменты и знания, необходимые для ответственного использования возможностей машинного обучения в финансовом секторе. Финансовый мир всегда был сферой сложности, отмеченной волатильностью, неопределенностью и динамической взаимосвязанностью. Традиционные модели и инструменты часто изо всех сил пытались охватить многогранную природу этой области. Однако методы машинного обучения предлагают смену парадигмы, предоставляя возможность обрабатывать огромные объемы данных, определять закономерности и генерировать идеи, которые ранее были невообразимы. В главах этой книги мы исследуем фундаментальные принципы машинного обучения и то, как их можно применять для решения множества финансовых проблем. От прогнозного моделирования, оценки рисков, алгоритмической торговли, оптимизации портфеля, обнаружения мошенничества до сегментации клиентов - потенциальные приложения безграничны. Объектно-ориентированное программирование на Python в сочетании с мощностями NumPy, Matplotlib и Jupyter идеально подходит для проектирования и визуализации в финансовой инженерии.
Approches du Machine arning en analyse financière Dans le monde de la finance en évolution rapide et constante d'aujourd'hui, il est de plus en plus important de disposer de guides intégrés et pratiques pour l'application du Machine arning en analyse financière. Ce livre répond à ce besoin croissant en offrant une ressource précieuse pour les débutants et les professionnels expérimentés de la finance et de la science des données. Il comble le fossé entre la théorie et la pratique en fournissant aux lecteurs les outils et les connaissances nécessaires pour exploiter de manière responsable les possibilités d'apprentissage automatique dans le secteur financier. monde financier a toujours été une sphère de complexité marquée par la volatilité, l'incertitude et l'interconnexion dynamique. s modèles et outils traditionnels ont souvent eu du mal à couvrir la nature multidimensionnelle de ce domaine. Cependant, les méthodes d'apprentissage automatique offrent un changement de paradigme, permettant de traiter d'énormes quantités de données, de définir des schémas et de générer des idées qui étaient auparavant inimaginables. Dans les chapitres de ce livre, nous explorons les principes fondamentaux de l'apprentissage automatique et comment ils peuvent être appliqués pour résoudre de nombreux problèmes financiers. De la modélisation prédictive, l'évaluation des risques, le trading algorithmique, l'optimisation du portefeuille, la détection de la fraude à la segmentation des clients, les applications potentielles sont illimitées. La programmation orientée objet sur Python, combinée aux capacités NumPy, Matplotlib et Jupyter, est idéale pour la conception et la visualisation en ingénierie financière.
Enfoques del aprendizaje automático en la analítica financiera En el mundo de las finanzas en rápida evolución y en constante evolución, es cada vez más importante contar con guías integrales y prácticas para la aplicación del aprendizaje automático en la analítica financiera. Este libro satisface esta creciente necesidad ofreciendo un recurso valioso tanto para principiantes como para profesionales experimentados en finanzas y ciencia de datos. Cierra la brecha entre la teoría y la práctica al proporcionar a los lectores las herramientas y los conocimientos necesarios para aprovechar de manera responsable las oportunidades de aprendizaje automático en el sector financiero. mundo financiero siempre ha sido un ámbito de complejidad marcado por la volatilidad, la incertidumbre y la interconexión dinámica. modelos e instrumentos tradicionales a menudo han luchado por abrazar la naturaleza multifacética de esta zona. n embargo, las técnicas de aprendizaje automático ofrecen un cambio de paradigma, proporcionando la capacidad de procesar enormes cantidades de datos, definir patrones y generar ideas que antes eran inimaginables. En los capítulos de este libro exploramos los principios fundamentales del aprendizaje automático y cómo se pueden aplicar para resolver muchos problemas financieros. Desde simulaciones predictivas, evaluación de riesgos, trading algorítmico, optimización de carteras, detección de fraudes hasta segmentación de clientes, las aplicaciones potenciales son ilimitadas. La programación orientada a objetos en Python, combinada con las capacidades NumPy, Matplotlib y Jupyter, es ideal para el diseño y la visualización en ingeniería financiera.
Ansätze für maschinelles rnen in der Finanzanalyse In der heutigen schnelllebigen und sich ständig weiterentwickelnden Finanzwelt wird die Notwendigkeit umfassender und praktischer itlinien für die Anwendung von maschinellem rnen in der Finanzanalyse immer wichtiger. Dieses Buch erfüllt diesen wachsenden Bedarf und bietet eine wertvolle Ressource für Anfänger und erfahrene Fachleute in den Bereichen Finanzen und Data Science. Es schließt die Lücke zwischen Theorie und Praxis, indem es den sern die Werkzeuge und das Wissen zur Verfügung stellt, die sie benötigen, um die Möglichkeiten des maschinellen rnens im Finanzsektor verantwortungsvoll zu nutzen. Die Finanzwelt war schon immer ein Bereich der Komplexität, geprägt von Volatilität, Unsicherheit und dynamischer Vernetzung. Traditionelle Modelle und Werkzeuge hatten oft Schwierigkeiten, die facettenreiche Natur dieses Gebiets zu erfassen. Methoden des maschinellen rnens bieten jedoch einen Paradigmenwechsel, der die Möglichkeit bietet, riesige Datenmengen zu verarbeiten, Muster zu erkennen und Ideen zu generieren, die zuvor unvorstellbar waren. In den Kapiteln dieses Buches untersuchen wir die grundlegenden Prinzipien des maschinellen rnens und wie sie angewendet werden können, um eine Vielzahl von finanziellen Problemen zu lösen. Von Predictive Modeling, Risikobewertung, algorithmischem Handel, Portfoliooptimierung, Betrugserkennung bis hin zur Kundensegmentierung - die Einsatzmöglichkeiten sind grenzenlos. Die objektorientierte Programmierung in Python in Kombination mit den Fähigkeiten von NumPy, Matplotlib und Jupyter ist ideal für Design und Visualisierung im Financial Engineering.
''
Finansal Analitikte Makine Öğrenimi Yaklaşımları Günümüzün hızlı ve sürekli gelişen finans dünyasında, makine öğrenimini finansal analitiğe uygulamak için kapsamlı ve pratik kılavuzlara duyulan ihtiyaç giderek daha önemli hale geliyor. Bu kitap, hem yeni başlayanlar hem de finans ve Veri Bilimi alanında deneyimli profesyoneller için değerli bir kaynak sunarak bu artan ihtiyacı karşılamaktadır. Okuyuculara, finansal sektördeki makine öğreniminin gücünü sorumlu bir şekilde kullanmak için ihtiyaç duydukları araçları ve bilgileri sağlayarak teori ve pratik arasındaki boşluğu kapatır. Finansal dünya her zaman volatilite, belirsizlik ve dinamik birbirine bağlılık ile işaretlenmiş bir karmaşıklık alanı olmuştur. Geleneksel modeller ve araçlar genellikle alanın çok yönlü doğasını yakalamak için mücadele etmişlerdir. Bununla birlikte, makine öğrenme teknikleri, çok miktarda veriyi işleme, kalıpları tanımlama ve daha önce hayal edilemeyen fikirler üretme yeteneği sağlayan bir paradigma değişimi sunar. Bu kitabın bölümlerinde, makine öğreniminin temel prensiplerini ve çeşitli finansal problemleri çözmek için nasıl uygulanabileceklerini araştırıyoruz. Tahmini modelleme, risk değerlendirmesi, algoritmik ticaret, portföy optimizasyonu, dolandırıcılık tespitinden müşteri segmentasyonuna kadar potansiyel uygulamalar sonsuzdur. NumPy, Matplotlib ve Jupyter'in gücüyle birleştirilen nesne yönelimli Python programlama, finansal mühendislikte tasarım ve görselleştirme için idealdir.
مناهج التعلم الآلي في التحليلات المالية في عالم التمويل سريع الخطى والمتطور باستمرار اليوم، أصبحت الحاجة إلى إرشادات شاملة وعملية لتطبيق التعلم الآلي على التحليلات المالية ذات أهمية متزايدة. يلبي هذا الكتاب هذه الحاجة المتزايدة من خلال تقديم مورد قيم لكل من المبتدئين والمهنيين ذوي الخبرة في التمويل وعلوم البيانات. إنه يسد الفجوة بين النظرية والممارسة من خلال تزويد القراء بالأدوات والمعرفة التي يحتاجون إليها لتسخير قوة التعلم الآلي في القطاع المالي بمسؤولية. لطالما كان العالم المالي مجال تعقيد يتسم بالتقلب وعدم اليقين والترابط الدينامي. غالبًا ما كافحت النماذج والأدوات التقليدية لالتقاط الطبيعة متعددة الأوجه لهذا المجال. ومع ذلك، تقدم تقنيات التعلم الآلي نقلة نوعية، حيث توفر القدرة على معالجة كميات هائلة من البيانات، وتحديد الأنماط، وتوليد أفكار لم يكن من الممكن تصورها في السابق. في فصول هذا الكتاب، نستكشف المبادئ الأساسية للتعلم الآلي وكيف يمكن تطبيقها لحل مجموعة متنوعة من المشكلات المالية. من النمذجة التنبؤية، وتقييم المخاطر، والتداول الخوارزمي، وتحسين المحفظة، والكشف عن الاحتيال إلى تجزئة العملاء، فإن التطبيقات المحتملة لا حصر لها. تعد برمجة بايثون الموجهة للكائنات جنبًا إلى جنب مع قوة NumPy و Matplotlib و Jupyter مثالية للتصميم والتصور في الهندسة المالية.
