
BOOKS - Federated Learning Techniques and Its Application in the Healthcare Industry

Federated Learning Techniques and Its Application in the Healthcare Industry
Author: H L Gururaj, Tanuja Kayarga, Francesco Flammini, Dalibor Dobrilovic
Year: 2024
Pages: 235
Format: PDF
File size: 13.1 MB
Language: ENG
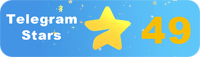
Year: 2024
Pages: 235
Format: PDF
File size: 13.1 MB
Language: ENG
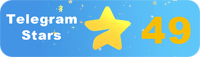
Federated Learning Techniques and Its Application in the Healthcare Industry Introduction: In today's digital age, technology has become an integral part of our lives, and machine learning has revolutionized the way we approach problems in various industries, including healthcare. One such emerging technology that has gained significant attention in recent times is Federated Learning (FL). This book delves into the concept of Federated Learning and its applications in the healthcare industry, highlighting its potential to transform the field while preserving patient data confidentiality. Chapter 1: Fundamentals of Federated Learning The first chapter provides a solid foundation for readers to understand the underlying principles of Federated Learning. It covers the following topics: 1. Definition and explanation of Federated Learning 2. Key features and benefits 3. Comparison with traditional machine learning methods 4. Importance and relevance in the healthcare industry Chapter 2: Federated Learning and its Classifications This chapter offers a comprehensive understanding of the diverse approaches and techniques used in different scenarios. It explores the following topics: 1. Types of Federated Learning (e. g. , horizontal, vertical, and hierarchical) 2. Applications in healthcare (e. g. , medical imaging, patient data analysis) 3. Advantages and limitations of each type Chapter 3: Federated Learning in Healthcare This chapter focuses on the application of Federated Learning in the healthcare industry, covering the following aspects: 1. Securing patient data privacy 2. Improving treatment outcomes 3. Predicting hospitalization due to cardiac events 4.
Федеративные методы обучения и их применение в отрасли здравоохранения Введение: В современную цифровую эпоху технологии стали неотъемлемой частью нашей жизни, а машинное обучение произвело революцию в подходе к проблемам в различных отраслях, включая здравоохранение. Одной из таких новых технологий, которая привлекла значительное внимание в последнее время, является Federated arning (FL). Эта книга углубляется в концепцию федеративного обучения и его приложений в отрасли здравоохранения, подчеркивая его потенциал для преобразования этой области при сохранении конфиденциальности данных пациентов. Глава 1: Основы федеративного обучения Первая глава предоставляет читателям прочную основу для понимания основополагающих принципов федеративного обучения. Он охватывает следующие темы: 1. Определение и объяснение федеративного обучения 2. Основные функции и преимущества 3. Сравнение с традиционными методами машинного обучения 4. Важность и актуальность в отрасли здравоохранения Глава 2: Федеративное обучение и его классификации Эта глава предлагает всестороннее понимание различных подходов и методов, используемых в различных сценариях. В ней исследуются следующие темы: 1. Типы федеративного обучения (например, горизонтальное, вертикальное и иерархическое) 2. Приложения в здравоохранении (например, медицинская визуализация, анализ данных пациентов) 3. Преимущества и ограничения каждого типа Глава 3: Федеративное обучение в здравоохранении Эта глава посвящена применению федеративного обучения в отрасли здравоохранения и охватывает следующие аспекты: 1. Обеспечение конфиденциальности данных пациентов 2. Улучшение результатов лечения 3. Прогнозирование госпитализации из-за сердечных событий 4.
Méthodes d'apprentissage fédérées et leur application dans l'industrie de la santé Introduction : À l'ère numérique moderne, la technologie est devenue une partie intégrante de nos vies, et l'apprentissage automatique a révolutionné l'approche des problèmes dans divers secteurs, y compris les soins de santé. L'une de ces nouvelles technologies, qui a attiré beaucoup d'attention récemment, est Federated arning (FL). Ce livre approfondit le concept de l'apprentissage fédératif et de ses applications dans l'industrie de la santé, soulignant sa capacité à transformer ce domaine tout en préservant la confidentialité des données des patients. Chapitre 1 : s fondements de l'apprentissage fédéral premier chapitre fournit aux lecteurs une base solide pour comprendre les principes fondamentaux de l'apprentissage fédéral. Il couvre les sujets suivants : 1. Définition et explication de l'apprentissage fédératif 2. Principales fonctions et avantages 3. Comparaison avec les méthodes traditionnelles d'apprentissage automatique 4. Importance et pertinence dans l'industrie des soins de santé Chapitre 2 : La formation fédérale et ses classifications Ce chapitre offre une compréhension approfondie des différentes approches et méthodes utilisées dans différents scénarios. Il explore les sujets suivants : 1. Types de formation fédérée (p. ex. horizontale, verticale et hiérarchique) 2. Applications dans le domaine des soins de santé (p. ex. imagerie médicale, analyse des données sur les patients) 3. Avantages et limites de chaque type Chapitre 3 : Formation fédérale en soins de santé Ce chapitre traite de l'application de la formation fédérale dans l'industrie de la santé et traite des aspects suivants : 1. Garantir la confidentialité des données des patients 2. Amélioration des résultats du traitement 3. Prédiction de l'hospitalisation due à des événements cardiaques 4.
Métodos de aprendizaje federados y su aplicación en la industria de la salud Introducción: En la era digital moderna, la tecnología se ha convertido en una parte integral de nuestras vidas, y el aprendizaje automático ha revolucionado el enfoque de los problemas en diversas industrias, incluida la salud. Una de estas nuevas tecnologías que ha atraído una atención considerable en los últimos tiempos es Federated Arning (FL). Este libro profundiza en el concepto de formación federada y sus aplicaciones en la industria sanitaria, destacando su potencial para transformar esta área al tiempo que mantiene la confidencialidad de los datos de los pacientes. Capítulo 1: Fundamentos del aprendizaje federado primer capítulo proporciona a los lectores una base sólida para comprender los principios fundamentales del aprendizaje federado. Abarca los siguientes temas: 1. Definición y explicación de la formación federada 2. Características y beneficios principales 3. Comparación con los métodos tradicionales de aprendizaje automático 4. Importancia y relevancia en la industria de la salud Capítulo 2: Formación federal y sus clasificaciones Este capítulo ofrece una comprensión integral de los diferentes enfoques y métodos utilizados en los diferentes escenarios. Explora los siguientes temas: 1. Tipos de aprendizaje federado (por ejemplo, horizontal, vertical y jerárquico) 2. Aplicaciones en salud (por ejemplo, imágenes médicas, análisis de datos de pacientes) 3. Beneficios y limitaciones de cada tipo Capítulo 3: Formación federal en salud Este capítulo se centra en la aplicación del aprendizaje federado en la industria de la salud y abarca los siguientes aspectos: 1. Garantizar la confidencialidad de los datos de los pacientes 2. Mejora de los resultados del tratamiento 3. Predicción de hospitalización debido a eventos cardíacos 4.
Metodi federati di apprendimento e loro applicazione nel settore sanitario Introduzione: Nell'era digitale moderna, la tecnologia è diventata parte integrante della nostra vita e l'apprendimento automatico ha rivoluzionato l'approccio alle sfide in diversi settori, tra cui l'assistenza sanitaria. Una di queste nuove tecnologie che ha attirato notevole attenzione recentemente è Federated arning (FL). Questo libro approfondisce il concetto di formazione federale e le sue applicazioni nel settore sanitario, evidenziando il suo potenziale di trasformazione in questo campo mantenendo la riservatezza dei dati dei pazienti. Capitolo 1: basi della formazione federale Il primo capitolo fornisce ai lettori una solida base per comprendere i principi fondanti dell'apprendimento federale. Riguarda i seguenti argomenti: 1. Definizione e spiegazione dell'apprendimento federale 2. Funzioni e vantaggi principali 3. Confronto con i metodi tradizionali di apprendimento automatico 4. Importanza e rilevanza nel settore sanitario Capitolo 2: La formazione federale e la sua classificazione Questo capitolo offre una comprensione completa dei diversi approcci e metodi utilizzati in diversi scenari. In esso vengono esplorati i seguenti argomenti: 1. Tipi di apprendimento federale (ad esempio orizzontale, verticale e gerarchico) 2. Applicazioni sanitarie (ad esempio visualizzazione medica, analisi dei dati dei pazienti) 3. I vantaggi e le limitazioni di ogni tipo Capitolo 3: La formazione federale nella sanità Questo capitolo è dedicato all'applicazione della formazione federale nel settore sanitario e comprende i seguenti aspetti: 1. Riservatezza dei dati dei pazienti 2. Migliorare i risultati del trattamento 3. Prognosi ospedaliera per eventi cardiaci 4.
Föderierte rnmethoden und ihre Anwendung im Gesundheitswesen Einleitung: Im heutigen digitalen Zeitalter sind Technologien zu einem festen Bestandteil unseres bens geworden, und maschinelles rnen hat die Herangehensweise an Probleme in verschiedenen Branchen, einschließlich des Gesundheitswesens, revolutioniert. Eine solche neue Technologie, die in letzter Zeit viel Aufmerksamkeit auf sich gezogen hat, ist Federated arning (FL). Dieses Buch vertieft das Konzept des föderierten rnens und seiner Anwendungen in der Gesundheitsbranche und unterstreicht sein Potenzial, diesen Bereich zu transformieren und gleichzeitig die Vertraulichkeit der Patientendaten zu wahren. Kapitel 1: Grundlagen des föderalen rnens Das erste Kapitel bietet den sern eine solide Grundlage, um die Grundprinzipien des föderativen rnens zu verstehen. Es umfasst die folgenden Themen: 1. Definition und Erläuterung des Verbundtrainings 2. Hauptmerkmale und Vorteile 3. Vergleich mit traditionellen Methoden des maschinellen rnens 4. Bedeutung und Relevanz in der Gesundheitsbranche Kapitel 2: Föderales rnen und seine Klassifikationen Dieses Kapitel bietet einen umfassenden Einblick in die verschiedenen Ansätze und Methoden, die in verschiedenen Szenarien verwendet werden. Folgende Themen werden untersucht: 1. Arten des Verbundunterrichts (z. B. horizontal, vertikal und hierarchisch) 2. Anwendungen im Gesundheitswesen (z.B. medizinische Bildgebung, Analyse von Patientendaten) 3. Vorteile und Grenzen jeder Art Kapitel 3: Föderales rnen im Gesundheitswesen Dieses Kapitel befasst sich mit der Anwendung des föderalen rnens im Gesundheitswesen und behandelt folgende Aspekte: 1. Gewährleistung der Vertraulichkeit von Patientendaten 2. Verbesserung der Behandlungsergebnisse3. Vorhersage von Krankenhausaufenthalten aufgrund von kardialen Ereignissen 4.
Sfederowane metody uczenia się i ich zastosowania w sektorze opieki zdrowotnej Wprowadzenie: W nowoczesnej erze cyfrowej technologia stała się integralną częścią naszego życia, a uczenie maszynowe zrewolucjonizowało sposób, w jaki podchodzimy do problemów w różnych branżach, w tym w opiece zdrowotnej. Jedną z takich nowych technologii, która przyciągnęła ostatnio znaczącą uwagę jest Federated arning (FL). Książka ta zagłębia się w koncepcję uczenia się w sposób federalny i jego zastosowania w sektorze opieki zdrowotnej, podkreślając jej potencjał w zakresie przekształcania tej dziedziny przy jednoczesnym zachowaniu prywatności danych pacjentów. Rozdział 1: Podstawy uczenia się sfederowanego Pierwszy rozdział zapewnia czytelnikom solidny fundament dla zrozumienia fundamentalnych zasad uczenia się federowanego. Obejmuje on następujące tematy: 1. Definicja i wyjaśnienie uczenia się sfederowanego 2. Najważniejsze cechy i korzyści 3. Porównanie z tradycyjnymi metodami uczenia maszynowego 4. Znaczenie i znaczenie w sektorze opieki zdrowotnej Rozdział 2: Uczenie się w sposób federalny i jego klasyfikacje Ten rozdział oferuje kompleksowe zrozumienie różnych podejść i metod stosowanych w różnych scenariuszach. Bada następujące tematy: 1. Sfederowane typy uczenia się (na przykład poziomy, pionowy i hierarchiczny) 2. Zastosowania w opiece zdrowotnej (np. obrazowanie medyczne, analiza danych pacjentów) 3. Korzyści i ograniczenia dla każdego typu rozdziału 3: Uczenie się w opiece zdrowotnej W rozdziale tym skupiono się na stosowaniu uczenia się w sektorze opieki zdrowotnej i obejmuje następujące aspekty: 1. Prywatność pacjenta 2. psze efekty leczenia 3. Przewidywanie hospitalizacji z powodu zdarzeń sercowych 4.
''
Federe öğrenme yöntemleri ve sağlık sektöründeki uygulamaları Giriş: Modern dijital çağda, teknoloji hayatımızın ayrılmaz bir parçası haline geldi ve makine öğrenimi, sağlık hizmetleri de dahil olmak üzere çeşitli endüstrilerdeki sorunlara yaklaşım biçimimizde devrim yarattı. Son zamanlarda dikkat çeken bu yeni teknolojilerden biri Federe arning (FL). Bu kitap, federe öğrenme kavramını ve sağlık sektöründeki uygulamalarını inceleyerek, hasta veri gizliliğini korurken bu alanı dönüştürme potansiyelini vurgulamaktadır. Bölüm 1: Federe Öğrenmenin Temelleri İlk bölüm, okuyuculara federe öğrenmenin temel ilkelerini anlamak için sağlam bir temel sağlar. Aşağıdaki konuları kapsar: 1. Federe öğrenmenin tanımı ve açıklaması 2. Temel özellikler ve avantajlar 3. Geleneksel makine öğrenme yöntemleri ile karşılaştırma 4. Sağlık Sektöründe Önem ve Uygunluk Bölüm 2: Federe öğrenme ve sınıflandırmaları Bu bölüm, farklı senaryolarda kullanılan farklı yaklaşım ve yöntemlerin kapsamlı bir şekilde anlaşılmasını sağlar. Aşağıdaki konuları inceler: 1. Birleşik öğrenme türleri (örneğin yatay, dikey ve hiyerarşik) 2. Sağlık alanındaki uygulamalar (örneğin tıbbi görüntüleme, hasta veri analizi) 3. Her Türün Yararları ve Sınırlamaları Bölüm 3: Sağlık Hizmetlerinde Federe Öğrenme Bu bölüm, sağlık sektöründe federe öğrenmenin uygulanmasına odaklanır ve aşağıdaki hususları kapsar: 1. Hasta mahremiyeti 2. Tedavi sonuçlarının iyileştirilmesi 3. Kardiyak olaylar nedeniyle hastaneye yatış tahmini 4.
أساليب التعلم الموحدة وتطبيقاتها في صناعة الرعاية الصحية مقدمة: في العصر الرقمي الحديث، أصبحت التكنولوجيا جزءًا لا يتجزأ من حياتنا وقد أحدث التعلم الآلي ثورة في الطريقة التي نتعامل بها مع المشكلات في مختلف الصناعات، بما في ذلك الرعاية الصحية. إحدى هذه التكنولوجيا الجديدة التي جذبت اهتمامًا كبيرًا مؤخرًا هي التعلم الموحد (FL). يتعمق هذا الكتاب في مفهوم التعلم الفيدرالي وتطبيقاته في صناعة الرعاية الصحية، ويسلط الضوء على قدرته على تحويل هذا المجال مع الحفاظ على خصوصية بيانات المريض. الفصل 1: أساسيات التعلم الموحد يوفر الفصل الأول للقراء أساسًا متينًا لفهم المبادئ الأساسية للتعلم الفيدرالي. وهو يغطي المواضيع التالية: 1. تعريف وشرح 2 التعلم الاتحادية. السمات والفوائد الرئيسية 3. مقارنة مع طرق التعلم الآلي التقليدية 4. الأهمية والأهمية في صناعة الرعاية الصحية الفصل 2: التعلم الموحد وتصنيفاته يقدم هذا الفصل فهمًا شاملاً للمناهج والطرق المختلفة المستخدمة في السيناريوهات المختلفة. وهو يستكشف المواضيع التالية: 1. أنواع التعلم الموحدة (على سبيل المثال، أفقية ورأسية وهرمية) 2. التطبيقات في الرعاية الصحية (مثل التصوير الطبي وتحليل بيانات المريض) 3. الفوائد والقيود لكل نوع الفصل 3: التعلم الموحد في الرعاية الصحية يركز هذا الفصل على تطبيق التعلم الاتحادي في صناعة الرعاية الصحية ويغطي الجوانب التالية: 1. خصوصية المريض 2. النتائج العلاجية المحسنة 3. توقع دخول المستشفى بسبب أحداث القلب 4.
