
BOOKS - PROGRAMMING - Evaluating Machine Learning Models A Beginner's Guide to Key Co...

Evaluating Machine Learning Models A Beginner's Guide to Key Concepts and Pitfalls
Author: Alice Zheng
Year: 2015
Pages: 58
Format: PDF
File size: , MB
Language: ENG
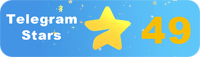
Year: 2015
Pages: 58
Format: PDF
File size: , MB
Language: ENG
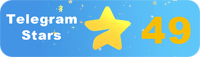
Book Evaluating Machine Learning Models A Beginner's Guide to Key Concepts and Pitfalls The world we live in today is constantly evolving, and technology plays a crucial role in shaping our future. One of the most significant technological advancements in recent years has been machine learning, which has revolutionized the way we approach problems and make decisions. However, as with any new technology, there are challenges and pitfalls that come with it, and it is essential to understand these key concepts to avoid costly mistakes. This book provides a beginner's guide to evaluating machine learning models, helping readers navigate the complex landscape of machine learning and make informed decisions about their use. The book begins by exploring the history and evolution of machine learning, providing context for the development of this technology. It then delves into the fundamental concepts of machine learning, including supervised and unsupervised learning, deep learning, and neural networks. The author explains these concepts in an accessible and simplified format, making them easy to understand for those who may not have a technical background. As the book progresses, it covers more advanced topics such as model evaluation, overfitting, and underfitting. Readers will learn how to assess the performance of their machine learning models and identify potential issues before they become major problems. The book also discusses common pitfalls in machine learning, such as bias and ethics, and provides practical tips on how to avoid them. Throughout the book, the author emphasizes the importance of understanding the process of technological evolution and its impact on society.
Book Evaluating Machine arning Models A Beginner's Guide to Key Concepts and Pitfalls Мир, в котором мы живем сегодня, постоянно развивается, и технологии играют решающую роль в формировании нашего будущего. Одним из самых значительных технологических достижений последних лет стало машинное обучение, которое произвело революцию в подходе к проблемам и принятию решений. Однако, как и с любой новой технологией, с ней связаны проблемы и подводные камни, и важно понимать эти ключевые понятия, чтобы избежать дорогостоящих ошибок. Эта книга представляет собой руководство для начинающих по оценке моделей машинного обучения, помогая читателям ориентироваться в сложном ландшафте машинного обучения и принимать обоснованные решения об их использовании. Книга начинается с изучения истории и эволюции машинного обучения, предоставляя контекст для развития этой технологии. Затем он углубляется в фундаментальные концепции машинного обучения, включая обучение с учителем и без учителя, глубокое обучение и нейронные сети. Автор объясняет эти понятия в доступном и упрощенном формате, благодаря чему их легко понять тем, у кого может не быть технической подготовки. По мере развития книги она охватывает более продвинутые темы, такие как оценка модели, переобучение и недообучение. Читатели узнают, как оценить эффективность своих моделей машинного обучения и выявить потенциальные проблемы до того, как они станут серьезными проблемами. В книге также обсуждаются общие подводные камни в машинном обучении, такие как предвзятость и этика, и даются практические советы о том, как их избежать. На протяжении всей книги автор подчеркивает важность понимания процесса технологической эволюции и его влияния на общество.
Book Evaluation Machine arning Models A Beginner's Guide to Key Concepts and Pitfalls monde dans lequel nous vivons aujourd'hui évolue constamment et la technologie joue un rôle crucial dans la formation de notre avenir. L'une des avancées technologiques les plus importantes de ces dernières années a été l'apprentissage automatique, qui a révolutionné l'approche des problèmes et la prise de décision. Cependant, comme pour toute nouvelle technologie, elle comporte des problèmes et des pièges, et il est important de comprendre ces concepts clés pour éviter les erreurs coûteuses. Ce livre est un guide pour les débutants sur l'évaluation des modèles d'apprentissage automatique, aidant les lecteurs à naviguer dans le paysage complexe de l'apprentissage automatique et à prendre des décisions éclairées sur leur utilisation. livre commence par une étude de l'histoire et de l'évolution de l'apprentissage automatique, fournissant un contexte pour le développement de cette technologie. Il approfondit ensuite les concepts fondamentaux de l'apprentissage automatique, y compris l'apprentissage avec et sans professeur, l'apprentissage profond et les réseaux neuronaux. L'auteur explique ces concepts dans un format accessible et simplifié, ce qui permet à ceux qui n'ont peut-être pas de formation technique de les comprendre facilement. Au fur et à mesure que le livre progresse, il aborde des sujets plus avancés tels que l'évaluation du modèle, le recyclage et le sous-enseignement. s lecteurs apprennent à évaluer l'efficacité de leurs modèles d'apprentissage automatique et à identifier les problèmes potentiels avant qu'ils ne deviennent des problèmes majeurs. livre examine également les pièges communs de l'apprentissage automatique, tels que les préjugés et l'éthique, et donne des conseils pratiques sur la façon de les éviter. Tout au long du livre, l'auteur souligne l'importance de comprendre le processus d'évolution technologique et son impact sur la société.
Book Evaluating Machine arning Models A Beginner's Guide to Key Concepts and Pitfalls mundo en el que vivimos hoy está en constante evolución y la tecnología juega un papel crucial en la configuración de nuestro futuro. Uno de los avances tecnológicos más significativos de los últimos ha sido el aprendizaje automático, que ha revolucionado el enfoque de los problemas y la toma de decisiones. n embargo, como con cualquier nueva tecnología, los problemas y los escollos están asociados con ella, y es importante entender estos conceptos clave para evitar costosos errores. Este libro es una guía para principiantes en la evaluación de modelos de aprendizaje automático, ayudando a los lectores a navegar por el complejo panorama del aprendizaje automático y tomar decisiones informadas sobre su uso. libro comienza con el estudio de la historia y evolución del aprendizaje automático, proporcionando un contexto para el desarrollo de esta tecnología. Luego profundiza en los conceptos fundamentales del aprendizaje automático, incluyendo el aprendizaje con y sin maestro, el aprendizaje profundo y las redes neuronales. autor explica estos conceptos en un formato accesible y simplificado, por lo que son fáciles de entender por aquellos que pueden no tener formación técnica. A medida que el libro avanza, abarca temas más avanzados como la evaluación del modelo, la re-enseñanza y la falta de comunicación. lectores aprenderán a evaluar la eficacia de sus modelos de aprendizaje automático e identificar posibles problemas antes de que se conviertan en problemas graves. libro también analiza los escollos comunes en el aprendizaje automático, como el sesgo y la ética, y da consejos prácticos sobre cómo evitarlos. A lo largo del libro, el autor destaca la importancia de entender el proceso de evolución tecnológica y su impacto en la sociedad.
Book Evaluating Machine arning Models A Beginner's Guia to Key Concepts and Pitfalls O mundo em que vivemos hoje está em constante evolução, e a tecnologia tem um papel crucial na formulação do nosso futuro. Um dos maiores avanços tecnológicos dos últimos anos foi o aprendizado de máquinas, que revolucionou a abordagem dos problemas e a tomada de decisões. No entanto, como com qualquer nova tecnologia, ela envolve problemas e pedras submarinas, e é importante compreender esses conceitos essenciais para evitar erros caros. Este livro é um guia para modelos de aprendizagem de máquina iniciantes, ajudando os leitores a navegar na complexa paisagem do aprendizado de máquinas e a tomar decisões razoáveis sobre o seu uso. O livro começa com o estudo da história e evolução do aprendizado de máquinas, fornecendo o contexto para o desenvolvimento desta tecnologia. Depois, aprofundou-se nos conceitos fundamentais da aprendizagem de máquinas, incluindo o ensino com o professor e sem o professor, o aprendizado profundo e as redes neurais. O autor explica estes conceitos em um formato acessível e simplificado, o que torna fácil compreendê-los para aqueles que podem não ter treinamento técnico. À medida que o livro avança, ele abrange temas mais avançados, como avaliação de modelo, reaproveitamento e subnutrição. Os leitores aprendem como avaliar a eficácia de seus modelos de aprendizagem de máquinas e identificar problemas potenciais antes que eles se tornem problemas sérios. O livro também discute pedras subaquáticas comuns no aprendizado de máquinas, como preconceito e ética, e dá conselhos práticos sobre como evitá-las. Ao longo do livro, o autor ressalta a importância de compreender o processo de evolução tecnológica e seus efeitos na sociedade.
Book Evaluating Machine arning Models A Beginner's Guide to Key Concept and Pitfalls Il mondo in cui viviamo oggi è in continua evoluzione e la tecnologia è fondamentale per la formazione del nostro futuro. Uno dei più importanti progressi tecnologici degli ultimi anni è stato l'apprendimento automatico, che ha rivoluzionato l'approccio alle sfide e alla decisione. Tuttavia, come con ogni nuova tecnologia, i problemi e le pietre sottomarine sono collegati, ed è importante comprendere questi concetti chiave per evitare errori costosi. Questo libro è un manuale per i modelli di apprendimento automatico iniziali, aiutando i lettori a orientarsi nel complesso panorama dell'apprendimento automatico e a prendere decisioni fondate sul loro utilizzo. Il libro inizia studiando la storia e l'evoluzione dell'apprendimento automatico, fornendo un contesto per lo sviluppo di questa tecnologia. Poi si approfondisce nei concetti fondamentali dell'apprendimento automatico, tra cui l'apprendimento con l'insegnante e senza l'insegnante, l'apprendimento profondo e le reti neurali. L'autore spiega questi concetti in un formato accessibile e semplificato, rendendoli facilmente comprensibili a coloro che potrebbero non avere una formazione tecnica. Man mano che il libro si sviluppa, si tratta di temi più avanzati, come la valutazione del modello, la riqualificazione e la mancanza di apprendimento. I lettori sapranno come valutare l'efficacia dei loro modelli di apprendimento automatico e identificare i potenziali problemi prima che diventino problemi seri. Il libro parla anche di pietre sottomarine comuni nell'apprendimento automatico, come il pregiudizio e l'etica, e fornisce consigli pratici su come evitarle. Durante tutto il libro, l'autore sottolinea l'importanza di comprendere l'evoluzione tecnologica e il suo impatto sulla società.
Book Evaluating Machine arning Models A Beginner 's Guide to Key Concepts and Pitfalls Die Welt, in der wir heute leben, entwickelt sich ständig weiter und Technologie gestaltet unsere Zukunft entscheidend mit. Einer der bedeutendsten technologischen Fortschritte der letzten Jahre war das maschinelle rnen, das die Herangehensweise an Probleme und die Entscheidungsfindung revolutionierte. Wie bei jeder neuen Technologie sind jedoch Probleme und Fallstricke damit verbunden, und es ist wichtig, diese Schlüsselkonzepte zu verstehen, um kostspielige Fehler zu vermeiden. Dieses Buch ist ein itfaden für Anfänger, um Modelle des maschinellen rnens zu bewerten und den sern zu helfen, durch die komplexe Landschaft des maschinellen rnens zu navigieren und fundierte Entscheidungen über ihre Verwendung zu treffen. Das Buch beginnt mit der Erforschung der Geschichte und Entwicklung des maschinellen rnens und bietet einen Kontext für die Entwicklung dieser Technologie. Anschließend vertieft er sich in grundlegende Konzepte des maschinellen rnens, darunter das rnen mit und ohne hrer, Deep arning und neuronale Netze. Der Autor erklärt diese Konzepte in einem zugänglichen und vereinfachten Format, so dass sie für diejenigen, die möglicherweise keine technische Ausbildung haben, leicht zu verstehen sind. Während sich das Buch entwickelt, deckt es fortgeschrittenere Themen wie Modellbewertung, Umschulung und Nicht-rnen ab. Die ser lernen, die Wirksamkeit ihrer Machine-arning-Modelle zu bewerten und potenzielle Probleme zu identifizieren, bevor sie zu ernsthaften Problemen werden. Das Buch diskutiert auch allgemeine Fallstricke im maschinellen rnen wie Voreingenommenheit und Ethik und gibt praktische Ratschläge, wie man sie vermeiden kann. Während des gesamten Buches betont der Autor die Bedeutung des Verständnisses des technologischen Evolutionsprozesses und seiner Auswirkungen auf die Gesellschaft.
Book Evaluating Machine aring Models A Beginner's Guide to Key Concepts and Pitfalls העולם בו אנו חיים כיום מתפתח ללא הרף, והטכנולוגיה ממלאת תפקיד מכריע בעיצוב עתידנו. אחת ההתקדמות הטכנולוגית המשמעותית ביותר בשנים האחרונות היא למידת מכונה, שחוללה מהפכה בפתרון בעיות ובקבלת החלטות. אולם, בדומה לכל טכנולוגיה חדשה, ישנן בעיות ומלכודות הקשורות אליה, וחשוב להבין את מושגי המפתח הללו כדי להימנע מטעויות יקרות. ספר זה הוא מדריך למתחילים להערכת מודלים ללימוד מכונה, מסייע לקוראים לנווט בנוף למידת המכונה המורכבת ולקבל החלטות מושכלות על השימוש בהם. הספר מתחיל בחקר ההיסטוריה והאבולוציה של למידת מכונה, ומספק הקשר להתפתחות טכנולוגיה זו. לאחר מכן הוא מתעמק במושגי למידת מכונה בסיסיים, כולל למידה מפוקחת ובלתי מפוקחת, למידה עמוקה ורשתות עצביות. המחבר מסביר את המושגים הללו בפורמט נגיש ומופשט, דבר המקל על הבנתם של מי שאין להם הכשרה טכנית. ככל שהספר מתקדם, הוא מכסה נושאים מתקדמים יותר כגון הערכת מודל, אימון מחדש ואימונים נמוכים. הקוראים ילמדו להעריך את יעילותם של מודלים ללימוד מכונה ולזהות בעיות פוטנציאליות לפני שהם יהפכו לבעיות רציניות. הספר גם דן במלכודות נפוצות בלימוד מכונה, כגון הטיה ואתיקה, ומספק עצות מעשיות כיצד להימנע מהן. לאורך הספר מדגיש המחבר את החשיבות של הבנת תהליך האבולוציה הטכנולוגית והשפעתה על החברה.''
Makine Öğrenimi Modellerini Değerlendirme Kitabı Temel Kavramlar ve Tuzaklar için Başlangıç Kılavuzu Bugün yaşadığımız dünya sürekli gelişiyor ve teknoloji geleceğimizi şekillendirmede çok önemli bir rol oynuyor. Son yılların en önemli teknolojik gelişmelerinden biri, problem çözme ve karar vermede devrim yaratan makine öğrenimi olmuştur. Bununla birlikte, herhangi bir yeni teknolojide olduğu gibi, bununla ilgili sorunlar ve tuzaklar vardır ve maliyetli hatalardan kaçınmak için bu temel kavramları anlamak önemlidir. Bu kitap, makine öğrenimi modellerini değerlendirmek, okuyucuların karmaşık makine öğrenimi ortamında gezinmelerine ve kullanımları hakkında bilinçli kararlar almalarına yardımcı olmak için bir başlangıç kılavuzudur. Kitap, makine öğreniminin tarihini ve evrimini keşfederek ve bu teknolojinin gelişimi için bağlam sağlayarak başlıyor. Daha sonra denetlenen ve denetlenmeyen öğrenme, derin öğrenme ve sinir ağları dahil olmak üzere temel makine öğrenimi kavramlarına girer. Yazar, bu kavramları erişilebilir ve basitleştirilmiş bir biçimde açıklar ve teknik eğitime sahip olmayanlar için anlaşılmasını kolaylaştırır. Kitap ilerledikçe, model değerlendirme, yeniden eğitim ve yetersiz eğitim gibi daha ileri konuları kapsar. Okuyucular, makine öğrenme modellerinin etkinliğini nasıl değerlendireceklerini ve ciddi problemler haline gelmeden önce potansiyel problemleri nasıl tanımlayacaklarını öğreneceklerdir. Kitap ayrıca önyargı ve etik gibi makine öğrenimindeki yaygın tuzakları tartışıyor ve bunlardan nasıl kaçınılacağı konusunda pratik tavsiyeler sunuyor. Kitap boyunca yazar, teknolojik evrim sürecini ve toplum üzerindeki etkisini anlamanın önemini vurgulamaktadır.
كتاب | تقييم نماذج التعلم الآلي دليل المبتدئين للمفاهيم والمزالق الرئيسية يتطور العالم الذي نعيش فيه اليوم باستمرار، وتلعب التكنولوجيا دورًا حاسمًا في تشكيل مستقبلنا. كان التعلم الآلي أحد أهم التطورات التكنولوجية في السنوات الأخيرة، مما أحدث ثورة في حل المشكلات وصنع القرار. ومع ذلك، كما هو الحال مع أي تقنية جديدة، هناك مشاكل ومزالق مرتبطة بها، ومن المهم فهم هذه المفاهيم الرئيسية لتجنب الأخطاء المكلفة. هذا الكتاب هو دليل للمبتدئين لتقييم نماذج التعلم الآلي، ومساعدة القراء على التنقل في مشهد التعلم الآلي المعقد واتخاذ قرارات مستنيرة بشأن استخدامها. يبدأ الكتاب باستكشاف تاريخ وتطور التعلم الآلي، مما يوفر سياقًا لتطوير هذه التكنولوجيا. ثم يتعمق في مفاهيم التعلم الآلي الأساسية، بما في ذلك التعلم الخاضع للإشراف وغير الخاضع للإشراف، والتعلم العميق، والشبكات العصبية. يشرح المؤلف هذه المفاهيم في شكل يسهل الوصول إليه ومبسط، مما يسهل فهمها لأولئك الذين قد لا يتلقون تدريبًا تقنيًا. مع تقدم الكتاب، فإنه يغطي مواضيع أكثر تقدمًا مثل تقييم النموذج وإعادة التدريب ونقص التدريب. سيتعلم القراء كيفية تقييم فعالية نماذج التعلم الآلي الخاصة بهم وتحديد المشكلات المحتملة قبل أن تصبح مشاكل خطيرة. يناقش الكتاب أيضًا المخاطر الشائعة في التعلم الآلي، مثل التحيز والأخلاق، ويقدم نصائح عملية حول كيفية تجنبها. في جميع أنحاء الكتاب، يؤكد المؤلف على أهمية فهم عملية التطور التكنولوجي وتأثيرها على المجتمع.
기계 학습 모델 평가 주요 개념과 함정에 대한 초보자 안내서 오늘날 우리가 살고있는 세상은 끊임없이 발전하고 있으며 기술은 미래를 형성하는 데 중요한 역할을합니다. 최근 몇 년 동안 가장 중요한 기술 발전 중 하나는 기계 학습으로 문제 해결 및 의사 결정에 혁명을 일으켰습니다. 그러나 새로운 기술과 마찬가지로 문제와 함정이 있으며 비용이 많이 드는 실수를 피하기 위해 이러한 주요 개념을 이해하는 것이 중요합니다. 이 책은 머신 러닝 모델을 평가하기위한 초보자 안내서로, 독자가 복잡한 머신 러닝 환경을 탐색하고 사용에 대한 정보에 입각 한 결정을 내릴 수 있도록 도와줍니다. 이 책은 머신 러닝의 역사와 진화를 탐구하여이 기술의 발전을위한 맥락을 제공함으로써 시작됩니다. 그런 다음 감독 및 감독되지 않은 학습, 딥 러닝 및 신경망을 포함한 기본 머신 러닝 개념을 탐구합니다. 저자는 이러한 개념을 액세스 가능하고 단순화 된 형식으로 설명하여 기술 교육을받지 않은 사람들에게는 쉽게 이해할 수 있습니다. 책이 진행됨에 따라 모델 평가, 재교육 및 교육 부족과 같은 고급 주제를 다룹니다. 독자는 머신 러닝 모델의 효과를 평가하고 심각한 문제가되기 전에 잠재적 인 문제를 식별하는 방법을 배웁니다. 이 책은 또한 편견 및 윤리와 같은 기계 학습의 일반적인 함정에 대해 설명하고이를 피하는 방법에 대한 실질적인 조언을 제공합니다. 이 책 전체에서 저자는 기술 진화 과정과 사회에 미치는 영향을 이해하는 것의 중요성을 강조합니다.
機械学習モデルを評価する本キーコンセプトと落とし穴の初心者ガイド今日の世界は絶えず進化しており、テクノロジーは私たちの未来を形作る上で重要な役割を果たしています。近の最も重要な技術進歩の1つは機械学習であり、問題解決と意思決定に革命をもたらしました。しかし、新しいテクノロジーと同様に、それに関連する問題や落とし穴があり、これらの重要な概念を理解してコストのかかるミスを回避することが重要です。この本は、機械学習モデルを評価するための初心者向けガイドであり、読者が複雑な機械学習の状況をナビゲートし、使用に関する情報に基づいた意思決定を行うのを支援します。この本は、機械学習の歴史と進化を探求し、この技術の発展のための文脈を提供することから始まります。その後、監督され、監視されていない学習、ディープラーニング、ニューラルネットワークなど、基本的な機械学習の概念を掘り下げます。著者は、これらの概念をアクセスしやすく簡略化された形式で説明し、技術トレーニングを受けていない人にとっては理解しやすいものにしています。本の進行に伴い、モデル評価、再訓練、アンダートレーニングなどのより高度なトピックをカバーしています。読者は、機械学習モデルの有効性を評価し、深刻な問題になる前に潜在的な問題を特定する方法を学びます。この本では、バイアスや倫理などの機械学習における一般的な落とし穴についても説明し、それらを回避する方法について実践的なアドバイスを提供しています。著者は本書を通じて、技術進化の過程を理解し、社会に与える影響の重要性を強調している。
Book Evaluating Machine arning Models A Beginner's Guide to Key Concepts and Pitfalls我們今天生活的世界正在不斷發展,技術在塑造我們的未來方面發揮著至關重要的作用。近來最重要的技術進步之一是機器學習,它徹底改變了解決問題和決策的方法。但是,與任何新技術一樣,它也存在問題和陷阱,重要的是要了解這些關鍵概念,以避免昂貴的錯誤。本書為初學者提供了評估機器學習模型的指南,幫助讀者導航復雜的機器學習環境並對其使用做出明智的決定。本書首先研究機器學習的歷史和演變,為該技術的發展提供了背景。然後,他深入研究機器學習的基本概念,包括與老師和無老師一起學習,深度學習和神經網絡。作者以易於理解和簡化的方式解釋了這些概念,使那些可能缺乏技術培訓的人很容易理解這些概念。隨著本書的發展,它涵蓋了更高級的主題,例如模型評估,再培訓和學習障礙。讀者將學習如何評估他們的機器學習模型的有效性,並在它們成為嚴重問題之前識別潛在的問題。該書還討論了機器學習中的常見陷阱,例如偏見和倫理,並就如何避免它們提供了實用建議。在整個書中,作者強調了了解技術進化過程及其對社會影響的重要性。
