
BOOKS - PROGRAMMING - Understanding Deep Learning Application in Rare Event Predictio...

Understanding Deep Learning Application in Rare Event Prediction
Author: Chitta Ranjan
Year: 2022
Pages: 428
Format: PDF
File size: 11.4 MB
Language: ENG
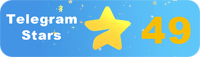
Year: 2022
Pages: 428
Format: PDF
File size: 11.4 MB
Language: ENG
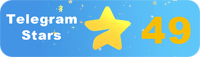
''
