
BOOKS - Statistical Prediction and Machine Learning

Statistical Prediction and Machine Learning
Author: John Tuhao Chen, Lincy Y. Chen, Clement Lee
Year: 2024
Pages: 315
Format: PDF
File size: 10.1 MB
Language: ENG
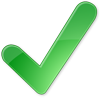
Year: 2024
Pages: 315
Format: PDF
File size: 10.1 MB
Language: ENG
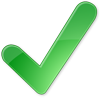
Book Description: Statistical Prediction and Machine Learning Author: Experienced Statistics Educator and Two Data Scientists Overview: This book bridges the knowledge gap between conventional statistics and contemporary machine learning, providing an accessible approach for readers with a basic statistics background to develop a mastery of machine learning. It starts by elucidating examples in Chapter 1 and fundamentals on refined optimization in Chapter 2, followed by common supervised learning methods such as regression, classification, support vector machines, tree algorithms, and range regressions. The book then delves into unsupervised learning methods and statistical learning with data sequentially or simultaneously from multiple resources. Chapter 1: Examples The first chapter provides concrete examples of how statistical prediction and machine learning can be applied to real-world problems. These examples illustrate the practicality and relevance of these techniques in various fields, making them easier to understand and relate to. Chapter 2: Fundamentals The second chapter covers the foundational concepts of statistical thinking and machine learning, including refined optimization methods that are essential for modern data analysis. This chapter prepares readers for the more advanced topics covered in the rest of the book.
Statistical Prediction and Machine arning Author: Experient Statistics Educator and Two Data Scientists Overview: This book перекрывает разрыв в знаниях между традиционной статистикой и современным машинным обучением, обеспечивая доступный подход для читателей с базовым статистическим опытом для развития мастерства машинного обучения. Он начинается с объяснения примеров в главе 1 и основ уточненной оптимизации в главе 2, за которыми следуют общие методы обучения с учителем, такие как регрессия, классификация, машины опорных векторов, алгоритмы деревьев и регрессии диапазонов. Затем книга углубляется в методы обучения без учителя и статистическое обучение с данными последовательно или одновременно из нескольких ресурсов. Глава 1: Примеры В первой главе приводятся конкретные примеры того, как статистическое прогнозирование и машинное обучение могут быть применены к реальным проблемам. Эти примеры иллюстрируют практичность и актуальность этих методов в различных областях, облегчая их понимание и связь с ними. Глава 2: Основы Вторая глава охватывает основополагающие концепции статистического мышления и машинного обучения, включая уточненные методы оптимизации, которые необходимы для современного анализа данных. Эта глава готовит читателей к более продвинутым темам, рассматриваемым в остальной части книги.
Statistical Prediction and Machine Arning Author : Experient Statistics Educator and Two Data Scientists Overview : Ce livre comble le fossé de connaissances entre les statistiques traditionnelles et l'apprentissage machine moderne en offrant une approche accessible aux lecteurs ayant une expérience statistique de base pour développer le savoir-faire machine. Il commence par expliquer les exemples du chapitre 1 et les bases de l'optimisation affinée du chapitre 2, suivies de méthodes d'apprentissage communes avec l'enseignant telles que la régression, la classification, les machines de vecteurs de référence, les algorithmes d'arbres et la régression des gammes. Ensuite, le livre approfondit les méthodes d'enseignement sans professeur et l'enseignement statistique avec des données successives ou simultanées à partir de plusieurs ressources. Chapitre 1 : Exemples premier chapitre donne des exemples concrets de la façon dont la prévision statistique et l'apprentissage automatique peuvent être appliqués à des problèmes réels. Ces exemples illustrent la faisabilité et la pertinence de ces techniques dans différents domaines, ce qui facilite leur compréhension et leur association. Chapitre 2 : Bases deuxième chapitre traite des concepts fondamentaux de la pensée statistique et de l'apprentissage automatique, y compris des méthodes d'optimisation perfectionnées qui sont nécessaires à l'analyse moderne des données. Ce chapitre prépare les lecteurs à des sujets plus avancés traités dans le reste du livre.
Predicación estadística y arning Author: Experience Statistics Educator and Two Data Scientists Overview: This book solapa la brecha de conocimiento entre las estadísticas tradicionales y el aprendizaje automático moderno, proporcionando un enfoque asequible para los lectores con experiencia estadística básica para desarrollar la habilidad del aprendizaje automático. Comienza explicando los ejemplos en el capítulo 1 y los fundamentos de la optimización refinada en el capítulo 2, seguidos de los métodos generales de aprendizaje con el maestro, tales como regresión, clasificación, máquinas de vectores de referencia, algoritmos de árboles y regresiones de rangos. A continuación, el libro profundiza en los métodos de enseñanza sin profesor y el aprendizaje estadístico con datos sucesivos o simultáneos de varios recursos. Capítulo 1: Ejemplos primer capítulo proporciona ejemplos específicos de cómo la predicción estadística y el aprendizaje automático pueden aplicarse a problemas reales. Estos ejemplos ilustran la practicidad y relevancia de estas técnicas en diferentes campos, facilitando su comprensión y conexión con ellas. Capítulo 2: Fundamentos segundo capítulo abarca los conceptos fundamentales del pensamiento estadístico y el aprendizaje automático, incluyendo técnicas refinadas de optimización que son necesarias para el análisis moderno de datos. Este capítulo prepara a los lectores para los temas más avanzados tratados en el resto del libro.
Statistical Prediction and Machine arning Autor: Experimental Statistics Educator and Two Data Scientists Übersicht: Dieses Buch schließt die Wissenslücke zwischen traditioneller Statistik und modernem maschinellen rnen und bietet einen zugänglichen Ansatz für ser mit grundlegender statistischer Erfahrung, um die Beherrschung des maschinellen rnens zu entwickeln. Es beginnt mit der Erläuterung der Beispiele in Kapitel 1 und der Grundlagen der verfeinerten Optimierung in Kapitel 2, gefolgt von allgemeinen Methoden des hrunterrichts wie Regression, Klassifizierung, Stützvektormaschinen, Baumalgorithmen und Bereichsregression. Das Buch geht dann tiefer in die Methoden des unbeaufsichtigten rnens und des statistischen rnens mit Daten nacheinander oder gleichzeitig aus mehreren Ressourcen. Kapitel 1: Beispiele Das erste Kapitel liefert konkrete Beispiele, wie statistische Prognosen und maschinelles rnen auf reale Probleme angewendet werden können. Diese Beispiele veranschaulichen die Praktikabilität und Relevanz dieser Methoden in verschiedenen Bereichen und erleichtern ihr Verständnis und ihre Verbindung mit ihnen. Kapitel 2: Grundlagen Das zweite Kapitel behandelt die grundlegenden Konzepte des statistischen Denkens und des maschinellen rnens, einschließlich verfeinerter Optimierungsmethoden, die für die moderne Datenanalyse unerlässlich sind. Dieses Kapitel bereitet die ser auf fortgeschrittenere Themen vor, die im Rest des Buches behandelt werden.
''
İstatistiksel Tahmin ve Makine Gelişimi Yazar: Uzman İstatistik Eğitimcisi ve İki Veri Bilimcisi Genel Bakış: Bu kitap, geleneksel istatistik ve modern makine öğrenimi arasındaki bilgi boşluğunu kapatarak, makine öğrenimi ustalığını geliştirmek için temel istatistiksel deneyime sahip okuyucular için erişilebilir bir yaklaşım sunmaktadır. Bölüm 1'deki örnekleri ve bölüm 2'deki rafine optimizasyonun temellerini açıklayarak başlar, ardından regresyon, sınıflandırma, destek vektör makineleri, ağaç algoritmaları ve aralık regresyonları gibi genel denetimli öğrenme yöntemleri izler. Kitap daha sonra denetlenmeyen öğretim yöntemlerine ve birden fazla kaynaktan sırayla veya eşzamanlı olarak verilerle istatistiksel öğrenmeye girer. Bölüm 1: Örnekler İlk bölüm, istatistiksel tahmin ve makine öğreniminin gerçek dünya problemlerine nasıl uygulanabileceğine dair özel örnekler sunmaktadır. Bu örnekler, bu yöntemlerin çeşitli alanlardaki pratikliğini ve alaka düzeyini gösterir ve onları anlamayı ve ilişkilendirmeyi kolaylaştırır. Bölüm 2: Temeller İkinci bölüm, modern veri analizi için gerekli olan rafine optimizasyon teknikleri de dahil olmak üzere istatistiksel düşünme ve makine öğreniminin temel kavramlarını kapsar. Bu bölüm, okuyucuları kitabın geri kalanında ele alınan daha gelişmiş konulara hazırlar.
مؤلف التنبؤ الإحصائي والتعلم الآلي: معلم إحصاءات خبير ونظرة عامة على اثنين من علماء البيانات: يسد هذا الكتاب فجوة المعرفة بين الإحصاءات التقليدية والتعلم الآلي الحديث، مما يوفر نهجًا يسهل الوصول إليه للقراء ذوي الخبرة الإحصائية الأساسية لتطوير إتقان التعلم الآلي. يبدأ بشرح الأمثلة الواردة في الفصل 1 وأساسيات التحسين المنقح في الفصل 2، تليها طرق عامة للتعلم الخاضع للإشراف مثل الانحدار والتصنيف وآلات ناقلات الدعم وخوارزميات الأشجار وانحدارات المدى. ثم يتعمق الكتاب في طرق التدريس غير الخاضعة للإشراف والتعلم الإحصائي باستخدام البيانات بشكل متسلسل أو متزامن من مصادر متعددة. الفصل 1: أمثلة يقدم الفصل الأول أمثلة محددة لكيفية تطبيق التنبؤ الإحصائي والتعلم الآلي على مشاكل العالم الحقيقي. وتوضح هذه الأمثلة الطابع العملي لهذه الأساليب وأهميتها في مختلف المجالات، مما يسهل فهمها والارتباط بها. الفصل 2: الأساسيات يغطي الفصل الثاني المفاهيم الأساسية للتفكير الإحصائي والتعلم الآلي، بما في ذلك تقنيات التحسين المحسنة الضرورية لتحليل البيانات الحديثة. يعد هذا الفصل القراء للمواضيع الأكثر تقدمًا التي يغطيها بقية الكتاب.
