
BOOKS - The Elements of Statistical Learning: Data Mining, Inference, and Prediction

The Elements of Statistical Learning: Data Mining, Inference, and Prediction
Author: Jerome Hastie, Trevor; Tibshirani, Robert; Friedman
Year: January 1, 2001
Format: PDF
File size: PDF 4.0 MB
Language: English
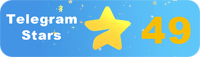
Year: January 1, 2001
Format: PDF
File size: PDF 4.0 MB
Language: English
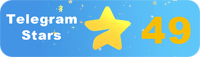
The Elements of Statistical Learning: Data Mining, Inference, and Prediction In the past decade, there has been an explosion in computation and information technology, leading to vast amounts of data in various fields such as medicine, biology, finance, and marketing. This deluge of data has spurred the development of new tools in statistics and spawned new areas like data mining, machine learning, and bioinformatics. However, understanding these data poses a significant challenge, which has led to the creation of new tools and techniques. The Elements of Statistical Learning: Data Mining, Inference, and Prediction is a comprehensive resource that provides a common conceptual framework for these diverse areas. Written by Trevor Hastie, Robert Tibshirani, and Jerome Friedman - all professors of statistics at Stanford University - this book offers a broad coverage of topics, from supervised learning prediction to unsupervised learning. It includes neural networks, support vector machines, classification trees, and boosting, making it the first comprehensive treatment of these subjects in any book. The authors are prominent researchers in the field, with Hastie and Tibshirani developing generalized additive models and inventing principal curves and surfaces, while Friedman co-invented many data mining tools, including CART, MARS, and projection pursuit.
Элементы статистического обучения: интеллектуальный анализ, вывод и прогнозирование данных В последнее десятилетие произошел взрыв в вычислительных и информационных технологиях, что привело к огромным объемам данных в различных областях, таких как медицина, биология, финансы и маркетинг. Этот поток данных стимулировал разработку новых инструментов в статистике и породил новые области, такие как интеллектуальный анализ данных, машинное обучение и биоинформатика. Однако понимание этих данных представляет собой серьезную проблему, которая привела к созданию новых инструментов и методов. The Elements of Statistical arning: Data Mining, Inference, and Prediction - комплексный ресурс, обеспечивающий общую концептуальную основу для этих разнообразных областей. Написанная Тревором Хасти, Робертом Тибширани и Джеромом Фридманом - всеми профессорами статистики в Стэнфордском университете - эта книга предлагает широкий охват тем, от прогнозирования с учителем до обучения без учителя. Он включает нейронные сети, машины опорных векторов, деревья классификации и бустинг, что делает его первым комплексным лечением этих предметов в любой книге. Авторы являются выдающимися исследователями в этой области, причем Hastie и Tibshirani разрабатывают обобщенные аддитивные модели и изобретают основные кривые и поверхности, в то время как Фридман совместно изобрел многие инструменты интеллектуального анализа данных, включая CART, MARS и поиск проекций.
Éléments de formation statistique : exploration, inférence et prévision des données Au cours de la dernière décennie, il y a eu une explosion des technologies de l'information et de l'informatique, ce qui a entraîné d'énormes quantités de données dans divers domaines tels que la médecine, la biologie, la finance et le marketing. Ce flux de données a stimulé le développement de nouveaux outils dans les statistiques et a donné naissance à de nouveaux domaines tels que l'exploration de données, l'apprentissage automatique et la bioinformatique. Toutefois, la compréhension de ces données constitue un défi majeur qui a conduit à la création de nouveaux outils et méthodes. The Elements of Statistical Arning : Data Mining, Inference, and Prediction est une ressource complète qui fournit un cadre conceptuel commun pour ces différents domaines. Écrit par Trevor Hasti, Robert Tibshirani et Jerome Friedman - tous professeurs de statistiques à l'Université de Stanford - ce livre offre une large couverture des sujets, de la prévision avec un professeur à l'apprentissage sans professeur. Il comprend des réseaux neuronaux, des machines de vecteurs de référence, des arbres de classification et de rappel, ce qui en fait le premier traitement complet de ces sujets dans n'importe quel livre. s auteurs sont des chercheurs exceptionnels dans ce domaine, Hastie et Tibshirani développant des modèles additifs généralisés et inventant des courbes et des surfaces de base, tandis que Friedman a co-inventé de nombreux outils d'exploration de données, y compris CART, MARS et la recherche de projections.
Elementos de aprendizaje estadístico: análisis inteligente, conclusión y predicción de datos En la última década se ha producido una explosión en la informática y la tecnología de la información, que ha dado lugar a enormes cantidades de datos en diversos campos como la medicina, la biología, las finanzas y el marketing. Este flujo de datos impulsó el desarrollo de nuevas herramientas en estadística y generó nuevos campos como la minería de datos, el aprendizaje automático y la bioinformática. n embargo, la comprensión de estos datos representa un desafío importante que ha llevado a la creación de nuevas herramientas y métodos. Elementos del Arco Estadístico: Minería de Datos, Inferencia, y Predicción es un recurso integral que proporciona un marco conceptual común para estas diversas áreas. Escrito por Trevor Hasty, Robert Tibshirani y Jerome Friedman - todos profesores de estadística en la Universidad de Stanford -, este libro ofrece una amplia cobertura de temas, desde la predicción con un profesor hasta el aprendizaje sin profesor. Incluye redes neuronales, máquinas de vectores de apoyo, árboles de clasificación y boosting, lo que lo convierte en el primer tratamiento integral de estos objetos en cualquier libro. autores son investigadores destacados en este campo, con Hastie y Tibshirani desarrollando modelos aditivos generalizados e inventando curvas y superficies básicas, mientras que Friedman ha inventado conjuntamente muchas herramientas de minería de datos, incluyendo CART, MARS y la búsqueda de proyecciones.
Elementos de aprendizado estatístico: análise inteligente, conclusão e previsão de dados Na última década, houve uma explosão na computação e na tecnologia da informação, resultando em grandes quantidades de dados em vários campos, tais como medicina, biologia, finanças e marketing. Este fluxo de dados estimulou o desenvolvimento de novas ferramentas nas estatísticas e gerou novas áreas, como análise inteligente de dados, aprendizagem de máquinas e bioinformática. No entanto, a compreensão desses dados representa um grande desafio que levou à criação de novas ferramentas e métodos. The Elents of Statical arning: Data Mining, Inference, and Precision é um recurso completo que fornece uma base conceitual comum para essas áreas variadas. Escrito por Trevor Husty, Robert Tebshirani e Jerome Friedman - todos os professores de estatística da Universidade de Stanford - este livro oferece um amplo alcance de temas que vão da previsão com o professor à formação sem o professor. Ele inclui redes neurais, máquinas de vetores de apoio, árvores de classificação e busting, tornando-o o o primeiro tratamento complexo para esses itens em qualquer livro. Os autores são grandes pesquisadores nesta área, e a Hasie e a Tibshirani desenvolvem modelos aditivos genéricos e inventam as principais curvas e superfícies, enquanto Friedman inventou em conjunto muitas ferramentas de análise inteligente de dados, incluindo CART, MARS e pesquisa de projeções.
Elementi di apprendimento statistico: analisi intelligente, acquisizione e previsione dei dati Nell'ultimo decennio si è verificata un'esplosione delle tecnologie informatiche e informatiche, che ha portato ad enormi quantità di dati in diversi settori quali medicina, biologia, finanza e marketing. Questo flusso di dati ha stimolato lo sviluppo di nuovi strumenti statistici e ha creato nuove aree come l'analisi intelligente dei dati, l'apprendimento automatico e la bioinformatica. Tuttavia, la comprensione di questi dati rappresenta un problema serio che ha portato alla creazione di nuovi strumenti e metodi. The Elents of Statistical arning: Data Mining, Inference, and Predition è una risorsa completa che fornisce una base concettuale comune per queste diverse aree. Scritto da Trevor Husty, Robert Tibschirani e Jerome Friedman - tutti i professori di statistica della Stanford University - questo libro offre una vasta gamma di argomenti che vanno dalla previsione con l'insegnante alla formazione senza insegnante. Include reti neurali, macchine di supporto vettori, alberi di classificazione e boosting che lo rendono il primo trattamento completo di questi oggetti in qualsiasi libro. Gli autori sono eccellenti ricercatori in questo campo, con Hastie e Tibshirani che sviluppano modelli additivi generalizzati e inventano curve e superfici di base, mentre Friedman ha inventato insieme molti strumenti di analisi intelligente dei dati, tra cui CART, MARS e ricerca di proiezioni.
Elemente des statistischen rnens: Intelligente Analyse, Ausgabe und Vorhersage von Daten Im letzten Jahrzehnt gab es eine Explosion in der Computer- und Informationstechnologie, die zu riesigen Datenmengen in verschiedenen Bereichen wie Medizin, Biologie, Finanzen und Marketing führte. Diese Datenflut hat die Entwicklung neuer Werkzeuge in der Statistik vorangetrieben und neue Bereiche wie Data Mining, maschinelles rnen und Bioinformatik hervorgebracht. Das Verständnis dieser Daten stellt jedoch eine große Herausforderung dar, die zur Schaffung neuer Werkzeuge und Methoden geführt hat. Die Elemente des statistischen rnens: Data Mining, Inference und Prediction sind eine umfassende Ressource, die einen gemeinsamen konzeptionellen Rahmen für diese vielfältigen Bereiche bietet. Geschrieben von Trevor Hastie, Robert Tibshirani und Jerome Friedman - alle Statistikprofessoren an der Stanford University - bietet dieses Buch eine breite Palette von Themen, von der Vorhersage mit dem hrer bis zum rnen ohne den hrer. Es umfasst neuronale Netze, Stützvektormaschinen, Klassifikationsbäume und Boosting und ist damit die erste umfassende Behandlung dieser Themen in jedem Buch. Die Autoren sind herausragende Forscher auf diesem Gebiet, wobei Hastie und Tibshirani generalisierte additive Modelle entwickeln und grundlegende Kurven und Oberflächen erfinden, während Friedman viele Data-Mining-Tools wie CART, MARS und Projektionssuche mitentwickelt hat.
Elements of Statistical arning: Data Mining, Inference and Forecasting W ostatnim dziesięcioleciu doszło do eksplozji w informatyce i informatyce, co spowodowało ogromne ilości danych w różnych dziedzinach, takich jak medycyna, biologia, finanse i marketing. Ten przepływ danych pobudził rozwój nowych narzędzi w statystyce i spowodował powstanie nowych dziedzin, takich jak eksploracja danych, uczenie maszynowe i bioinformatyka. Zrozumienie tych danych stanowi jednak poważne wyzwanie, które doprowadziło do stworzenia nowych narzędzi i metod. The Elements of Statistical arning: Data Mining, Inference, and Prediction jest kompleksowym zasobem, który zapewnia wspólne ramy koncepcyjne dla tych różnorodnych dziedzin. Napisane przez Trevora Hastie, Roberta Tibshiraniego i Hieronima Friedmana - wszystkich profesorów statystyki na Uniwersytecie Stanforda - ta książka oferuje szeroki zakres tematów, od prognozowania kierowanego przez nauczyciela po naukę bez nadzoru. Obejmuje sieci neuronowe, obsługę maszyn wektorowych, drzewa klasyfikacyjne i wzmacnianie, co czyni go pierwszym kompleksowym traktowaniem tych elementów w każdej książce. Autorzy są wybitnymi badaczami w tej dziedzinie, z Hastie i Tibshirani opracowuje uogólnione modele addytywne i wynalezienie podstawowych krzywych i powierzchni, podczas gdy Friedman współtworzył wiele narzędzi do eksploracji danych, w tym CART, MARS i wyszukiwania projekcji.
''
İstatistiksel Öğrenmenin Unsurları: Veri Madenciliği, Çıkarım ve Tahmin Son on yılda bilgisayar ve bilgi teknolojisinde bir patlama yaşandı ve tıp, biyoloji, finans ve pazarlama gibi çeşitli alanlarda büyük miktarda veri elde edildi. Bu veri akışı, istatistikte yeni araçların geliştirilmesini teşvik etti ve veri madenciliği, makine öğrenimi ve biyoinformatik gibi yeni alanlar ortaya çıkardı. Bununla birlikte, bu verileri anlamak, yeni araçların ve yöntemlerin oluşturulmasına yol açan büyük bir zorluktur. İstatistiksel Arting Elemanları: Veri Madenciliği, Çıkarım ve Tahmin bu farklı alanlar için ortak bir kavramsal çerçeve sağlayan kapsamlı bir kaynaktır. Stanford Üniversitesi'ndeki tüm istatistik profesörleri olan Trevor Hastie, Robert Tibshirani ve Jerome Friedman tarafından yazılan bu kitap, öğretmen liderliğindeki tahminlerden denetimsiz öğrenmeye kadar çok çeşitli konular sunmaktadır. nir ağlarını, destek vektör makinelerini, sınıflandırma ağaçlarını ve güçlendirmeyi içerir, bu da bu öğelerin herhangi bir kitaptaki ilk kapsamlı tedavisini yapar. Yazarlar, Hastie ve Tibshirani'nin genelleştirilmiş katkı modelleri geliştirmesi ve temel eğrileri ve yüzeyleri icat etmesiyle bu alanda önde gelen araştırmacılardır; Friedman ise CART, MARS ve projeksiyon araştırması da dahil olmak üzere birçok veri madenciliği aracını birlikte icat etmiştir.
عناصر التعلم الإحصائي: تعدين البيانات والاستدلال والتنبؤ شهد العقد الماضي انفجارًا في تكنولوجيا الحوسبة والمعلومات، مما أدى إلى كميات هائلة من البيانات في مجالات مختلفة مثل الطب وعلم الأحياء والتمويل والتسويق. حفز تدفق البيانات هذا على تطوير أدوات جديدة في الإحصاء وأنتج مجالات جديدة مثل التنقيب عن البيانات والتعلم الآلي والمعلوماتية الحيوية. ومع ذلك، فإن فهم هذه البيانات يمثل تحديًا كبيرًا أدى إلى إنشاء أدوات وطرق جديدة. عناصر التعلم الإحصائي: استخراج البيانات والاستدلال والتنبؤ هي مورد شامل يوفر إطارًا مفاهيميًا مشتركًا لهذه المجالات المتنوعة. كتبه تريفور هاستي وروبرت تبشيراني وجيروم فريدمان - جميع أساتذة الإحصاء في جامعة ستانفورد - يقدم هذا الكتاب مجموعة واسعة من الموضوعات، من التنبؤ بقيادة المعلم إلى التعلم غير الخاضع للإشراف. يتضمن الشبكات العصبية وآلات ناقلات الدعم وأشجار التصنيف والتعزيز، مما يجعله أول علاج شامل لهذه العناصر في أي كتاب. المؤلفون باحثون بارزون في هذا المجال، حيث قام Hastie و Tibshirani بتطوير نماذج مضافة عامة واختراع المنحنيات والأسطح الأساسية، بينما شارك فريدمان في اختراع العديد من أدوات تعدين البيانات، بما في ذلك CART و MARS والبحث عن الإسقاط.
統計学習の要素:データマイニング、推論、予測この10間でコンピューティングと情報技術が爆発的に普及し、医学、生物学、金融、マーケティングなどのさまざまな分野で膨大なデータが得られました。このデータフローは、統計における新しいツールの開発を促進し、データマイニング、機械学習、バイオインフォマティクスなどの新しい分野を生み出しました。しかし、このデータを理解することは、新しいツールやメソッドの作成につながっている大きな課題を提示します。統計アーニングの要素:データマイニング、推論、予測は、これらの多様な分野に共通の概念フレームワークを提供する包括的なリソースです。Trevor Hastie、 Robert Tibshirani、 Jerome Friedmanによって書かれたスタンフォード大学の統計学教授であるこの本は、教師主導の予測から教師なし学習まで、幅広いトピックを提供しています。ニューラルネットワーク、サポートベクターマシン、分類ツリー、およびブーストが含まれており、どの書籍においてもこれらの項目の最初の包括的な扱いとなっています。Friedmanは、CART、 MARS、投影検索などの多くのデータマイニングツールを共同で開発しました。
統計學習要素:知識分析、數據推斷和預測過去十中計算和信息技術發生了爆發,導致醫學、生物學、金融和市場營銷等各個領域的大量數據。這種數據流推動了統計學新工具的開發,並催生了新的領域,例如數據挖掘,機器學習和生物信息學。但是,了解這些數據是一個重大挑戰,導致了新工具和方法的建立。統計偵察元素:數據挖掘,地勢和預測是一種綜合資源,為這些不同領域提供了共同的概念框架。這本書由Trevor Hasty,Robert Tibshirani和Jerome Friedman(斯坦福大學的所有統計學教授)撰寫,涵蓋了從與老師的預測到沒有老師的學習等主題。它包括神經網絡,參考向量機,分類樹和助推器,使其成為任何書中對這些主題的首次綜合治療。作者是該領域的傑出研究人員,Hastie和Tibshirani開發了廣義加法模型並發明了基本曲線和曲面,而Friedman共同發明了許多數據挖掘工具,包括CART,MARS和投影搜索。
