
BOOKS - Machine Learning for Causal Inference

Machine Learning for Causal Inference
Author: Sheng Li
Year: November 26, 2023
Format: PDF
File size: PDF 12 MB
Language: English
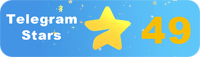
Year: November 26, 2023
Format: PDF
File size: PDF 12 MB
Language: English
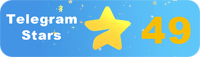
Book: Machine Learning for Causal Inference Introduction: In today's world, technology is rapidly evolving, and machine learning has become an integral part of our lives. With the advent of machine learning, we have witnessed tremendous growth in various sectors, including healthcare, finance, marketing, and more. However, as we delve deeper into the realm of machine learning, we need to understand the process of technological evolution and its impact on humanity. This book, "Machine Learning for Causal Inference," provides a profound insight into the relationship between machine learning and causal inference, exploring the challenges associated with it and presenting potential solutions. The book is a valuable resource for researchers, teachers, practitioners, and students interested in these fields, offering a deep understanding of the relationship between machine learning and causal inference. Chapter 1: Preliminary Foundations of Causal Inference The first chapter of the book begins by covering the preliminary foundations of causal inference, including basic definitions, illustrative examples, and assumptions. It sets the stage for the rest of the book, providing a solid foundation for readers to build upon. The chapter covers topics such as causality, confounding, selection bias, and identification, laying the groundwork for the subsequent chapters. Chapter 2: Classical Causal Inference Methods The second chapter delves into different types of classical causal inference methods, including matching, weighting, tree-based models, and more. These methods are essential for understanding the causal relationships between variables and are crucial in developing trustworthy machine learning systems. The chapter discusses the strengths and weaknesses of each method, allowing readers to appreciate their limitations and potential applications.
Book: Machine arning for Causal Inference Введение: В современном мире технологии быстро развиваются, и машинное обучение стало неотъемлемой частью нашей жизни. С появлением машинного обучения мы стали свидетелями огромного роста в различных секторах, включая здравоохранение, финансы, маркетинг и многое другое. Однако по мере того, как мы углубляемся в область машинного обучения, нам необходимо понимать процесс технологической эволюции и ее влияние на человечество. Эта книга «Машинное обучение для причинного вывода» дает глубокое понимание взаимосвязи между машинным обучением и причинным выводом, исследуя проблемы, связанные с ним, и представляя потенциальные решения. Книга является ценным ресурсом для исследователей, преподавателей, практиков и студентов, заинтересованных в этих областях, предлагая глубокое понимание взаимосвязи между машинным обучением и причинно-следственным выводом. Глава 1: Предварительные основания причинного вывода Первая глава книги начинается с освещения предварительных оснований причинного вывода, включая основные определения, иллюстративные примеры и предположения. Он закладывает основу для остальной части книги, обеспечивая прочную основу для читателей. Глава охватывает такие темы, как причинно-следственная связь, путаница, предвзятость выбора и идентификация, закладывая основу для последующих глав. Глава 2: Классические методы причинного вывода Во второй главе рассматриваются различные типы классических методов причинного вывода, включая сопоставление, взвешивание, модели на основе деревьев и многое другое. Эти методы необходимы для понимания причинно-следственных связей между переменными и имеют решающее значение при разработке заслуживающих доверия систем машинного обучения. В главе обсуждаются сильные и слабые стороны каждого метода, что позволяет читателям оценить их ограничения и потенциальные применения.
Book : Machine arning for Causal Inference Introduction : Dans le monde d'aujourd'hui, la technologie évolue rapidement et l'apprentissage automatique est devenu une partie intégrante de nos vies. Avec l'avènement du machine learning, nous avons connu une croissance spectaculaire dans divers secteurs, dont la santé, la finance, le marketing et bien plus encore. Cependant, alors que nous nous enfoncons dans le domaine de l'apprentissage automatique, nous devons comprendre le processus d'évolution technologique et son impact sur l'humanité. Ce livre « Machine arning for Causal Outdoor » fournit une compréhension approfondie de la relation entre Machine arning et Causal Outdoor, explorant les problèmes qui y sont liés et présentant des solutions potentielles. livre est une ressource précieuse pour les chercheurs, les enseignants, les praticiens et les étudiants intéressés par ces domaines, offrant une compréhension approfondie de la relation entre l'apprentissage automatique et la conclusion causale. Chapitre 1 : Motifs préliminaires de la conclusion causale premier chapitre du livre commence par mettre en évidence les motifs préliminaires de la conclusion causale, y compris les définitions de base, les exemples illustratifs et les hypothèses. Il jette les bases du reste du livre, fournissant une base solide pour les lecteurs. chapitre traite de sujets tels que la causalité, la confusion, le biais de choix et l'identification, jetant les bases des chapitres suivants. Chapitre 2 : Méthodes classiques d'inférence causale deuxième chapitre traite de différents types de méthodes classiques d'inférence causale, y compris la juxtaposition, la pondération, les modèles à base d'arbres et bien plus encore. Ces méthodes sont nécessaires pour comprendre les relations de cause à effet entre les variables et sont essentielles au développement de systèmes d'apprentissage automatique crédibles. chapitre examine les forces et les faiblesses de chaque méthode, ce qui permet aux lecteurs d'évaluer leurs limites et leurs applications potentielles.
Book: Machine arning for Causal Inference Introducción: En el mundo actual, la tecnología evoluciona rápidamente y el aprendizaje automático se ha convertido en una parte integral de nuestras vidas. Con la llegada del aprendizaje automático, hemos sido testigos de un enorme crecimiento en una variedad de sectores, incluyendo salud, finanzas, marketing y más. n embargo, a medida que profundizamos en el campo del aprendizaje automático, necesitamos entender el proceso de evolución tecnológica y su impacto en la humanidad. Este libro, «Machine arning for Causal Inference», proporciona una comprensión profunda de la relación entre el aprendizaje automático y la inferencia causal, investigando los problemas asociados con él y presentando soluciones potenciales. libro es un recurso valioso para investigadores, profesores, profesionales y estudiantes interesados en estos campos, ofreciendo una comprensión profunda de la relación entre el aprendizaje automático y la inferencia causal. Capítulo 1: bases preliminares de la inferencia causal primer capítulo del libro comienza con la iluminación de las bases preliminares de la inferencia causal, incluyendo definiciones básicas, ejemplos ilustrativos y suposiciones. enta las bases para el resto del libro, proporcionando una base sólida para los lectores. capítulo abarca temas como la causalidad, la confusión, el sesgo de elección y la identificación, sentando las bases para capítulos posteriores. Capítulo 2: Métodos clásicos de inferencia causal En el segundo capítulo se examinan diferentes tipos de métodos clásicos de inferencia causal, incluyendo la yuxtaposición, pesaje, modelos basados en árboles y más. Estas técnicas son necesarias para entender las relaciones causales entre variables y son cruciales en el desarrollo de sistemas de aprendizaje automático creíbles. capítulo analiza las fortalezas y debilidades de cada método, lo que permite a los lectores evaluar sus limitaciones y posibles aplicaciones.
Book: Machine arning for Causal Inference Introdução: No mundo atual, a tecnologia evolui rapidamente, e o aprendizado de máquinas tornou-se parte integrante das nossas vidas. Com o surgimento do aprendizado de máquinas, temos assistido a um enorme crescimento em vários setores, incluindo saúde, finanças, marketing e muito mais. No entanto, à medida que nos aprofundamos para o aprendizado de máquinas, precisamos compreender o processo de evolução tecnológica e seus efeitos na humanidade. Este livro «A aprendizagem de máquinas para a conclusão causal» oferece uma profunda compreensão da relação entre o aprendizado de máquinas e a conclusão causal, explorando os problemas associados a ele e apresentando soluções potenciais. O livro é um recurso valioso para pesquisadores, professores, praticantes e estudantes interessados nessas áreas, oferecendo uma compreensão profunda da relação entre o aprendizado de máquinas e a conclusão causal. Capítulo 1: Fundamentos preliminares da conclusão causal O primeiro capítulo do livro começa com a cobertura das bases preliminares da conclusão causal, incluindo definições básicas, exemplos ilustrativos e suposições. Ele estabelece as bases para o resto do livro, fornecendo uma base sólida para os leitores. O capítulo abrange temas como causalidade, confusão, preconceito de escolha e identificação, estabelecendo as bases para os capítulos seguintes. Capítulo 2: Métodos clássicos de causalidade O segundo capítulo aborda vários tipos de métodos clássicos de causalidade, incluindo comparação, ponderação, modelos baseados em árvores e muito mais. Estes métodos são essenciais para compreender os laços de causa e efeito entre as variáveis e essenciais para o desenvolvimento de sistemas credíveis de aprendizagem de máquinas. O capítulo discute os pontos fortes e fracos de cada método, permitindo que os leitores avaliem suas limitações e potenciais aplicações.
Machine arning for Causal Inference Introduzione: Nel mondo moderno la tecnologia si sta evolvendo rapidamente e l'apprendimento automatico è diventato parte integrante della nostra vita. Con l'apprendimento automatico abbiamo visto una crescita enorme in diversi settori, tra cui sanità, finanza, marketing e altro ancora. Tuttavia, mentre ci approfondiamo nel campo dell'apprendimento automatico, dobbiamo comprendere il processo di evoluzione tecnologica e il suo impatto sull'umanità. Questo libro «Apprendimento automatico per l'output causale» fornisce una profonda comprensione della relazione tra apprendimento automatico e causale, esplorando i problemi che ne derivano e presentando potenziali soluzioni. Il libro è una preziosa risorsa per ricercatori, insegnanti, professionisti e studenti interessati a queste aree, offrendo una profonda comprensione del rapporto tra apprendimento automatico e la conclusione causale. Capitolo 1: Basi preliminari del risultato causale Il primo capitolo del libro inizia con la copertura delle basi preliminari del risultato causale, comprese le definizioni di base, gli esempi illustrativi e i presupposti. Pone le basi per il resto del libro, fornendo solide basi per i lettori. Il capitolo riguarda argomenti quali causale, confusione, pregiudizio di scelta e identificazione, ponendo le basi per i successivi capitoli. Capitolo 2: Metodi classici di causale Il secondo capitolo affronta diversi tipi di metodi di output causale classici, tra cui mappatura, pesatura, modelli a base di alberi e molto altro ancora. Questi metodi sono necessari per comprendere i rapporti causali tra le variabili e sono fondamentali per sviluppare sistemi di apprendimento automatico credibili. Nel capitolo si discutono i punti forti e deboli di ogni metodo, permettendo ai lettori di valutare i loro limiti e potenziali applicazioni.
Buch: Machine arning for Causal Inference Einführung: In der heutigen Welt entwickelt sich die Technologie rasant weiter und maschinelles rnen ist zu einem festen Bestandteil unseres bens geworden. Mit dem Aufkommen des maschinellen rnens haben wir ein enormes Wachstum in einer Vielzahl von Sektoren erlebt, darunter Gesundheitswesen, Finanzen, Marketing und mehr. Wenn wir jedoch tiefer in den Bereich des maschinellen rnens eintauchen, müssen wir den Prozess der technologischen Evolution und ihre Auswirkungen auf die Menschheit verstehen. Dieses Buch, Machine arning for Causal Inferenz, bietet einen tiefen Einblick in die Beziehung zwischen maschinellem rnen und kausaler Inferenz, indem es die damit verbundenen Probleme untersucht und mögliche Lösungen vorstellt. Das Buch ist eine wertvolle Ressource für Forscher, hrer, Praktiker und Studenten, die an diesen Bereichen interessiert sind, und bietet ein tiefes Verständnis für die Beziehung zwischen maschinellem rnen und kausaler Inferenz. Kapitel 1: Vorläufige Gründe für eine kausale Schlussfolgerung Das erste Kapitel des Buches beginnt mit der Hervorhebung der vorläufigen Gründe für eine kausale Schlussfolgerung, einschließlich grundlegender Definitionen, illustrativer Beispiele und Annahmen. Es legt den Grundstein für den Rest des Buches und bietet eine solide Grundlage für die ser. Das Kapitel behandelt Themen wie Kausalität, Verwirrung, Selektionsverzerrung und Identifikation und legt die Grundlage für die nachfolgenden Kapitel. Kapitel 2: Klassische Methoden der kausalen Inferenz Im zweiten Kapitel werden verschiedene Arten klassischer Methoden der kausalen Inferenz untersucht, darunter Matching, Gewichtung, baumbasierte Modelle und vieles mehr. Diese Methoden sind notwendig, um kausale Zusammenhänge zwischen Variablen zu verstehen und sind entscheidend für die Entwicklung vertrauenswürdiger maschineller rnsysteme. Das Kapitel diskutiert die Stärken und Schwächen jeder Methode und ermöglicht es den sern, ihre Einschränkungen und möglichen Anwendungen zu bewerten.
''
Kitap: Nedensel Çıkarım için Makine Öğrenimi Giriş: Günümüz dünyasında teknoloji hızla gelişiyor ve makine öğrenimi hayatımızın ayrılmaz bir parçası haline geldi. Makine öğreniminin gelişiyle birlikte, sağlık, finans, pazarlama ve daha fazlası dahil olmak üzere sektörlerde büyük bir büyüme gördük. Bununla birlikte, makine öğrenimi alanına daha derinlemesine baktığımızda, teknolojik evrim sürecini ve insanlık üzerindeki etkisini anlamamız gerekir. "Machine arning for Causal Inference" (Nedensel Çıkarım için Makine Öğrenimi) adlı bu kitap, makine öğrenimi ile nedensel çıkarım arasındaki ilişkinin, onunla ilgili sorunları keşfederek ve potansiyel çözümler sunarak derinlemesine anlaşılmasını sağlar. Kitap, bu alanlarla ilgilenen araştırmacılar, eğitimciler, uygulayıcılar ve öğrenciler için değerli bir kaynaktır ve makine öğrenimi ile nedensel çıkarım arasındaki ilişkinin derinlemesine anlaşılmasını sağlar. Bölüm 1: Nedensel Çıkarım İçin Ön Gerekçeler Kitabın ilk bölümü, temel tanımlar, açıklayıcı örnekler ve varsayımlar dahil olmak üzere nedensel çıkarım için ön gerekçeleri vurgulayarak başlar. Kitabın geri kalanı için zemin hazırlar ve okuyucular için sağlam bir temel sağlar. Bölüm, nedensellik, karışıklık, seçim yanlılığı ve tanımlama gibi konuları kapsar ve sonraki bölümler için zemin hazırlar. Bölüm 2: Klasik Nedensel Çıkarım Yöntemleri İkinci bölüm, eşleştirme, ağırlıklandırma, ağaç tabanlı modeller ve daha fazlasını içeren farklı klasik nedensel çıkarım yöntemlerine bakar. Bu yöntemler, değişkenler arasındaki nedensel ilişkileri anlamak için gereklidir ve güvenilir makine öğrenme sistemlerinin geliştirilmesinde kritik öneme sahiptir. Bölüm, her bir yöntemin güçlü ve zayıf yönlerini tartışarak okuyucuların sınırlamalarını ve potansiyel uygulamalarını değerlendirmelerini sağlar.
كتاب |: التعلم الآلي للاستدلال السببي مقدمة: في عالم اليوم، تتطور التكنولوجيا بسرعة وأصبح التعلم الآلي جزءًا لا يتجزأ من حياتنا. مع ظهور التعلم الآلي، شهدنا نموًا هائلاً عبر القطاعات بما في ذلك الرعاية الصحية والتمويل والتسويق والمزيد. ومع ذلك، بينما نتعمق أكثر في مجال التعلم الآلي، نحتاج إلى فهم عملية التطور التكنولوجي وتأثيرها على البشرية. يوفر هذا الكتاب، «التعلم الآلي للاستدلال السببي»، فهمًا عميقًا للعلاقة بين التعلم الآلي والاستدلال السببي من خلال استكشاف المشكلات المرتبطة به وتقديم الحلول المحتملة. يعد الكتاب مصدرًا قيمًا للباحثين والمعلمين والممارسين والطلاب المهتمين بهذه المجالات، ويقدم فهمًا عميقًا للعلاقة بين التعلم الآلي والاستدلال السببي. الفصل 1: الأسباب الأولية للاستدلال السببي يبدأ الفصل الأول من الكتاب بتسليط الضوء على الأسباب الأولية للاستدلال السببي، بما في ذلك التعاريف الأساسية والأمثلة التوضيحية والافتراضات. إنه يضع الأساس لبقية الكتاب، ويوفر أساسًا متينًا للقراء. يغطي الفصل مواضيع مثل السببية والارتباك والتحيز للاختيار وتحديد الهوية، مما يضع الأساس للفصول اللاحقة. الفصل 2: الطرق الكلاسيكية للاستدلال السببي يبحث الفصل الثاني في أنواع مختلفة من الأساليب الكلاسيكية للاستدلال السببي، بما في ذلك المطابقة والترجيح والنماذج القائمة على الأشجار والمزيد. هذه الأساليب ضرورية لفهم العلاقات السببية بين المتغيرات وهي حاسمة في تطوير أنظمة تعلم آلي موثوقة. يناقش الفصل نقاط القوة والضعف في كل طريقة، مما يسمح للقراء بتقييم قيودهم وتطبيقاتهم المحتملة.
