
BOOKS - PROGRAMMING - Machine Learning Upgrade A Data Scientist's Guide to MLOps, LLM...

Machine Learning Upgrade A Data Scientist's Guide to MLOps, LLMs, and ML Infrastructure
Author: Kristen Kehrer, Caleb Kaiser
Year: 2024
Pages: 240
Format: PDF | EPUB
File size: 10.8 MB
Language: ENG
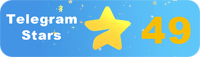
Year: 2024
Pages: 240
Format: PDF | EPUB
File size: 10.8 MB
Language: ENG
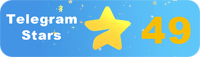
It is a much-needed guide for implementing new technology in workspaces, from experts in the field. Machine Learning Upgrade: A Data Scientist's Guide to MLOps, LLMs, and ML Infrastructure As technology continues to evolve at an unprecedented pace, it is essential for data scientists and managers to stay ahead of the curve and embrace the latest advancements in the field of machine learning (ML). This groundbreaking book provides a comprehensive overview of the modern ML landscape, offering a holistic approach to the development and implementation of large language models (LLMs) and other emerging technologies. The authors, experts in the field, introduce readers to the concept of "datacentricity which views unstructured data and LLMs as the foundation upon which countless applications and business solutions can be built. The book begins by exploring the challenges associated with modern ML, including data versioning, experiment tracking, post-production model monitoring, and deployment. These challenges are addressed through the adoption of best practices that enable data professionals and business leaders to leverage the full potential of ML. The authors provide real-world examples and code to help readers understand the practical applications of these techniques.
Это крайне необходимое руководство для внедрения новых технологий на рабочих местах от экспертов в данной области. Обновление машинного обучения: руководство Data Scientist по MLOps, LLM и инфраструктуре ML Поскольку технология продолжает развиваться беспрецедентными темпами, для специалистов по данным и менеджеров важно оставаться на опережение и использовать последние достижения в области машинного обучения (ML). Эта новаторская книга предоставляет всесторонний обзор современного ландшафта ML, предлагая целостный подход к разработке и внедрению больших языковых моделей (LLM) и других новых технологий. Авторы, эксперты в данной области, знакомят читателей с концепцией «центричности данных», которая рассматривает неструктурированные данные и LLM как основу, на которой могут быть построены бесчисленные приложения и бизнес-решения. Книга начинается с изучения проблем, связанных с современным ML, включая управление версиями данных, отслеживание экспериментов, мониторинг постпроизводственной модели и развертывание. Эти проблемы решаются путем внедрения передовых практик, которые позволяют специалистам по данным и лидерам бизнеса использовать весь потенциал ML. Авторы приводят реальные примеры и код, чтобы помочь читателям понять практическое применение этих методов.
C'est un guide essentiel pour l'introduction de nouvelles technologies sur le lieu de travail par des experts dans le domaine. Mise à jour du Machine arning : guide de Data Scientist sur les MLOps, les LLM et l'infrastructure ML Alors que la technologie continue d'évoluer à un rythme sans précédent, il est important pour les professionnels et les gestionnaires de données de rester à l'avant-garde et de tirer parti des dernières avancées du Machine arning (ML). Ce livre novateur offre un aperçu complet du paysage moderne de ML, offrant une approche holistique pour le développement et la mise en œuvre de grands modèles linguistiques (LLM) et d'autres nouvelles technologies. s auteurs, experts dans ce domaine, présentent aux lecteurs le concept de « centralité des données », qui considère les données non structurées et les LLM comme la base sur laquelle d'innombrables applications et solutions commerciales peuvent être construites. livre commence par une étude des problèmes liés aux LM modernes, y compris la gestion de la version des données, le suivi des expériences, la surveillance du modèle post-production et le déploiement. Ces défis sont résolus par la mise en œuvre de meilleures pratiques qui permettent aux professionnels des données et aux chefs d'entreprise d'exploiter tout le potentiel de ML. s auteurs donnent des exemples réels et un code pour aider les lecteurs à comprendre l'application pratique de ces méthodes.
Esta es una guía muy necesaria para la introducción de nuevas tecnologías en los lugares de trabajo por parte de expertos en la materia. Actualización del aprendizaje automático: guía de Data Scientist sobre MLOps, LLM e infraestructura ML A medida que la tecnología continúa evolucionando a un ritmo sin precedentes, es importante que los profesionales de datos y gerentes se mantengan al frente y aprovechen los últimos avances en aprendizaje automático (ML). Este libro pionero ofrece una visión global del panorama moderno del ML, ofreciendo un enfoque holístico para el desarrollo e implementación de grandes modelos lingüísticos (LLM) y otras nuevas tecnologías. autores, expertos en la materia, introducen a los lectores en el concepto de «centralidad de datos», que considera los datos no estructurados y la LLM como la base sobre la que se pueden construir innumerables aplicaciones y soluciones empresariales. libro comienza con el estudio de los problemas relacionados con el ML moderno, incluyendo la administración de versiones de datos, el seguimiento de experimentos, la supervisión del modelo post-producción y la implementación. Estos desafíos se resuelven mediante la implementación de mejores prácticas que permiten a los profesionales de datos y líderes empresariales aprovechar todo el potencial de ML. autores dan ejemplos reales y código para ayudar a los lectores a entender la aplicación práctica de estas técnicas.
É uma orientação essencial para a implementação de novas tecnologias no local de trabalho por especialistas na área. Atualização do aprendizado de máquina: Guia de Dados de MLOps, LLM e infraestrutura ML Como a tecnologia continua a evoluir a um ritmo sem precedentes, é importante que os especialistas em dados e gerentes se mantenham à frente e usem os avanços mais recentes no aprendizado de máquinas (ML). Este livro inovador oferece uma visão completa da paisagem moderna da ML, oferecendo uma abordagem holística para o desenvolvimento e implementação de grandes modelos linguísticos (LLM) e outras novas tecnologias. Os autores, especialistas na área, apresentam aos leitores o conceito de «centralidade de dados», que considera os dados não estruturados e o LLM como a base sobre a qual podem ser construídos inúmeros aplicativos e soluções de negócios. O livro começa com o estudo de problemas relacionados com o ML moderno, incluindo gerenciamento de versões de dados, rastreamento de experiências, monitoramento do modelo pós-produção e implantação. Estes desafios são resolvidos através da implementação de práticas avançadas que permitem aos especialistas em dados e líderes empresariais aproveitar todo o potencial da ML. Os autores citam exemplos reais e um código para ajudar os leitores a entender a aplicação prática destes métodos.
È una guida essenziale per l'implementazione di nuove tecnologie sul posto di lavoro da parte di esperti del settore. Aggiornamento dell'apprendimento automatico: guida di Data Scientist su MLOs, LLM e infrastruttura ML Poiché la tecnologia continua ad evolversi a un ritmo senza precedenti, è importante per gli esperti di dati e i gestori rimanere in anticipo e sfruttare i progressi più recenti nell'apprendimento automatico (ML). Questo innovativo libro offre una panoramica completa del panorama attuale di ML, offrendo un approccio olistico allo sviluppo e all'implementazione di modelli linguistici di grandi dimensioni (LLM) e di altre nuove tecnologie. Gli autori, esperti in questo campo, presentano ai lettori il concetto dì centralità dei dati ", che considera i dati non strutturati e LLM come la base su cui costruire innumerevoli applicazioni e soluzioni aziendali. Il libro inizia con l'analisi dei problemi legati all'attuale ML, tra cui la gestione delle versioni dei dati, il monitoraggio degli esperimenti, il monitoraggio dei modelli post-produzione e l'implementazione. Questi problemi vengono affrontati implementando procedure ottimali che consentono agli esperti di dati e ai leader aziendali di sfruttare tutto il potenziale di ML. Gli autori forniscono esempi reali e un codice per aiutare i lettori a comprendere l'applicazione pratica di questi metodi.
Dies ist ein dringend benötigter itfaden für die Einführung neuer Technologien am Arbeitsplatz durch Experten auf diesem Gebiet. Machine arning Update: Data Scientist Guide zu MLOps, LLM und ML-Infrastruktur Da sich die Technologie in einem beispiellosen Tempo weiterentwickelt, ist es für Data Scientists und Manager wichtig, immer einen Schritt voraus zu sein und die neuesten Fortschritte im Bereich Machine arning (ML) zu nutzen. Dieses wegweisende Buch bietet einen umfassenden Überblick über die aktuelle ML-Landschaft und bietet einen ganzheitlichen Ansatz für die Entwicklung und Implementierung von großen Sprachmodellen (LLM) und anderen neuen Technologien. Die Autoren, Experten auf diesem Gebiet, führen die ser in das Konzept der „Datenzentrizität“ ein, das unstrukturierte Daten und LLM als Grundlage für unzählige Anwendungen und Geschäftsentscheidungen sieht. Das Buch beginnt mit der Untersuchung der Probleme, die mit der modernen ML verbunden sind, einschließlich der Datenversionierung, der Verfolgung von Experimenten, der Überwachung des Postproduktionsmodells und der Bereitstellung. Diese Herausforderungen werden durch die Implementierung von Best Practices angegangen, die es Datenwissenschaftlern und Führungskräften ermöglichen, das volle Potenzial von ML auszuschöpfen. Die Autoren geben reale Beispiele und Code, um den sern zu helfen, die praktische Anwendung dieser Techniken zu verstehen.
Jest to podstawowy przewodnik do wdrażania nowych technologii w miejscu pracy od ekspertów w dziedzinie. Aktualizacja uczenia maszynowego: Przewodnik naukowca ds. danych do MLOp, LLM i ML Infrastructure Ponieważ technologia ta nadal rozwija się w bezprecedensowym tempie, ważne jest, aby naukowcy i menedżerowie danych utrzymali się przed krzywą i wykorzystali najnowsze postępy w nauce maszyn (ML). Ta przełomowa książka zapewnia kompleksowy przegląd nowoczesnego krajobrazu ML, oferując holistyczne podejście do rozwoju i wdrażania dużych modeli językowych (LLM) i innych nowych technologii. Autorzy, eksperci w tej dziedzinie, wprowadzają czytelników do koncepcji „centralności danych”, która postrzega niestrukturalne dane i LLM jako podstawę, na której można budować niezliczone aplikacje i rozwiązania biznesowe. Książka rozpoczyna się od zbadania wyzwań związanych z nowoczesnym ML, w tym weryfikacją danych, śledzeniem eksperymentów, monitorowaniem modelu postprodukcji i wdrożeniem. Wyzwania te są rozwiązywane poprzez wdrażanie najlepszych praktyk, które umożliwiają naukowcom i przywódcom przedsiębiorstw wykorzystanie pełnego potencjału ML. Autorzy podają przykłady i kod świata rzeczywistego, aby pomóc czytelnikom zrozumieć praktyczne zastosowanie tych metod.
זהו מדריך חיוני ליישום טכנולוגיות חדשות במקום העבודה ממומחים בתחום. עדכון למידת מכונה (Machine arning Adduction): מדריך למדעי המידע ל-MLOps, ל-LLM ולתשתית ML, כאשר הטכנולוגיה ממשיכה להתקדם בקצב חסר תקדים, חשוב למדעני נתונים ומנהלים להקדים את העקום ולמנף את ההתקדמות האחרונה בלימוד מכונה (ML). ספר פורץ דרך זה מספק סקירה מקיפה של נוף ה-ML המודרני, ומציע גישה הוליסטית לפיתוח ויישום של מודלים לשוניים גדולים (LLMs) וטכנולוגיות מתעוררות אחרות. המחברים, מומחים בתחום, מציגים בפני הקוראים את המושג ”מרכז נתונים”, הרואה בנתונים לא מובנים ובבסיס LLM אינספור יישומים ופתרונות עסקיים. הספר מתחיל בחקר האתגרים הקשורים ל-ML המודרנית, כולל איתור נתונים, מעקב אחר ניסויים, ניטור מודל לאחר ייצור, ופריסה. אתגרים אלה מופנים על ידי יישום שיטות טובות ביותר המאפשרות למדעני נתונים ומנהיגים עסקיים לרתום את מלוא הפוטנציאל של ML. המחברים מספקים דוגמאות וקודים מהעולם האמיתי כדי לעזור לקוראים להבין את היישום המעשי של שיטות אלה.''
Bu, alandaki uzmanlardan işyerinde yeni teknolojilerin uygulanması için önemli bir kılavuzdur. Makine Öğrenimi Güncellemesi: MLOps, LLM ve ML Altyapısı için Veri Bilimcisinin Kılavuzu Teknoloji benzeri görülmemiş bir hızda ilerlemeye devam ederken, veri bilimcileri ve yöneticileri için eğrinin önünde kalmak ve makine öğrenimindeki (ML) en son gelişmelerden yararlanmak önemlidir. Bu çığır açan kitap, büyük dil modellerinin (LLM'ler) ve diğer gelişmekte olan teknolojilerin geliştirilmesi ve uygulanması için bütünsel bir yaklaşım sunan modern ML peyzajına kapsamlı bir genel bakış sunmaktadır. Alanında uzman olan yazarlar, okuyucuları yapılandırılmamış verileri ve LLM'yi sayısız uygulama ve iş çözümünün inşa edilebileceği temel olarak gören "veri merkezlilik" kavramına tanıtmaktadır. Kitap, veri sürümü oluşturma, deney izleme, üretim sonrası model izleme ve dağıtım dahil olmak üzere modern ML ile ilgili zorlukları keşfederek başlıyor. Bu zorluklar, veri bilimcilerinin ve iş liderlerinin ML'nin tüm potansiyelini kullanmalarını sağlayan en iyi uygulamaları uygulayarak ele alınmaktadır. Yazarlar, okuyucuların bu yöntemlerin pratik uygulamasını anlamalarına yardımcı olmak için gerçek dünyadan örnekler ve kodlar sunmaktadır.
هذا دليل أساسي لتنفيذ التكنولوجيات الجديدة في مكان العمل من الخبراء في هذا المجال. تحديث التعلم الآلي: دليل عالم البيانات إلى MLOps و LLM و ML Infrastructure مع استمرار تقدم التكنولوجيا بوتيرة غير مسبوقة، من المهم لعلماء البيانات والمديرين البقاء في طليعة المنحنى والاستفادة من أحدث التطورات في التعلم الآلي (ML). يقدم هذا الكتاب الرائد لمحة عامة شاملة عن مشهد ML الحديث، ويقدم نهجًا شاملاً لتطوير وتنفيذ نماذج لغوية كبيرة (LLMs) وغيرها من التقنيات الناشئة. يقدم المؤلفون، الخبراء في هذا المجال، القراء إلى مفهوم «مركزية البيانات»، الذي يرى أن البيانات غير المنظمة و LLM هي الأساس الذي يمكن على أساسه بناء عدد لا يحصى من التطبيقات وحلول الأعمال. يبدأ الكتاب باستكشاف التحديات المرتبطة بـ ML الحديثة، بما في ذلك إصدار البيانات، وتتبع التجارب، ومراقبة نموذج ما بعد الإنتاج، والنشر. تتم معالجة هذه التحديات من خلال تنفيذ أفضل الممارسات التي تمكن علماء البيانات وقادة الأعمال من تسخير الإمكانات الكاملة لـ ML. يقدم المؤلفون أمثلة واقعية وشفرة لمساعدة القراء على فهم التطبيق العملي لهذه الأساليب.
이것은 해당 분야의 전문가로부터 작업장에서 새로운 기술을 구현하기위한 필수 지침입니다. 기계 학습 업데이트: MLops, LLM 및 ML 인프라에 대한 데이터 과학자 안내서 기술이 전례없는 속도로 계속 발전함에 따라 데이터 과학자와 관리자는 앞서 나가서 기계 학습 (ML) 의 최신 발전을 활용하는 것이 중요합니다.. 이 획기적인 책은 현대 ML 환경에 대한 포괄적 인 개요를 제공하여 대형 언어 모델 (LLM) 및 기타 신흥 기술의 개발 및 구현에 대한 전체적인 접근 방식을 제공합니다. 이 분야의 전문가 인 저자는 독자들에게 "데이터 중심성" 이라는 개념을 소개합니다.이 개념은 구조화되지 않은 데이터와 LLM을 수많은 응용 프로그램 및 비즈니스 솔루션을 구축 할 수있는 기초로보고 있습니다. 이 책은 데이터 버전 지정, 실험 추적, 후반 작업 모델 모니터링 및 배포를 포함하여 최신 ML과 관련된 문제를 탐색하는 것으로 시작됩니다. 이러한 과제는 데이터 과학자와 비즈니스 리더가 ML의 모든 잠재력을 활용할 수있는 모범 사례를 구현함으로써 해결 저자는 독자가 이러한 방법의 실제 적용을 이해하도록 돕기 위해 실제 예제와 코드를 제공합니다.
これは、現場の専門家から職場に新しい技術を実装するための不可欠なガイドです。機械学習のアップデート:MLOps、 LLM、およびMLインフラストラクチャへのデータサイエンティストのガイドテクノロジーは前例のないペースで進歩し続けているため、データサイエンティストや管理者は曲線を先取りし、機械学習(ML)の最新の進歩を活用することが重要です。この画期的な本は、現代のMLランドスケープの包括的な概要を提供し、大規模な言語モデル(LLM)およびその他の新興技術の開発と実装に全体的なアプローチを提供します。著者、分野の専門家は、無数のアプリケーションやビジネスソリューションを構築することができる基礎として非構造化データとLLMを見ている「データ中心性」の概念に読者を紹介します。本書は、データバージョニング、実験追跡、ポストプロダクションモデル監視、展開など、現代のMLに関連する課題を探ることから始まります。これらの課題は、データサイエンティストやビジネスリーダーがMLの可能性を最大限に活用できるベストプラクティスを実装することによって解決されます。著者たちは、実際の例とコードを提供して、読者がこれらの方法の実用的な応用を理解するのを助ける。
