
BOOKS - SCIENCE AND STUDY - Entropy Randomization in Machine Learning

Entropy Randomization in Machine Learning
Author: Yuri S. Popkov, Alexey Yu. Popkov, Yuri A. Dubnov
Year: 2023
Pages: 405
Format: PDF
File size: 13.4 MB
Language: ENG
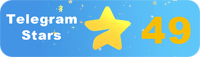
Year: 2023
Pages: 405
Format: PDF
File size: 13.4 MB
Language: ENG
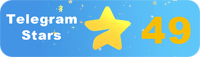
. The authors propose a new method of entropy randomization that allows to improve the accuracy of the model and reduce computational costs The book's main idea is to provide an accessible and simplified explanation of the concept of entropy randomization in machine learning, which is a powerful tool for solving complex problems of machine learning and data analysis. The author emphasizes the importance of understanding the process of technological evolution, including the development of new knowledge and its impact on society, as well as the need to develop a personal paradigm for perceiving this process as the basis for human survival and unity in a world of rapid technological change. The book begins by discussing the challenges of machine learning and data analysis in the modern world, where the amount of data is growing exponentially, and the complexity of the processes being studied is increasing. The author highlights the limitations of traditional machine learning methods and the need for new approaches to solve these problems. Then, he introduces the concept of entropy randomization, which involves using randomness in the modeling process to obtain optimal solutions under uncertainty. The author explains how entropy randomization works by using models with random parameters and maximum entropy estimates of the probability density functions of the model parameters under balance conditions with measured data. He provides examples of how this approach can be applied to various machine learning tasks, such as classification, regression, and clustering. The book also covers the theoretical foundations of entropy randomization, including information theory and probability theory, and shows how it can be used to improve the accuracy of the model and reduce computational costs. Throughout the book, the author emphasizes the importance of understanding the process of technological evolution and its impact on society.
.Авторы предлагают новый метод энтропийной рандомизации, позволяющий повысить точность модели и снизить вычислительные затраты Основная идея книги - дать доступное и упрощенное объяснение концепции энтропийной рандомизации в машинном обучении, что является мощным инструментом для решения сложных задач машинного обучения и анализа данных. Автор подчеркивает важность понимания процесса технологической эволюции, включая развитие новых знаний и их влияние на общество, а также необходимость выработки личностной парадигмы восприятия этого процесса как основы выживания и единства человека в мире быстрых технологических изменений. Книга начинается с обсуждения проблем машинного обучения и анализа данных в современном мире, где количество данных растет в геометрической прогрессии, а сложность изучаемых процессов увеличивается. Автор подчеркивает ограниченность традиционных методов машинного обучения и необходимость новых подходов для решения этих задач. Затем, он вводит понятие энтропийной рандомизации, которая предполагает использование случайности в процессе моделирования для получения оптимальных решений в условиях неопределённости. Автор объясняет, как работает рандомизация энтропии, используя модели со случайными параметрами и максимальными оценками энтропии функций плотности вероятности параметров модели в условиях баланса с измеренными данными. Он приводит примеры того, как этот подход может быть применен к различным задачам машинного обучения, таким как классификация, регрессия и кластеризация. Книга также охватывает теоретические основы энтропийной рандомизации, включая теорию информации и теорию вероятностей, и показывает, как её можно использовать для повышения точности модели и снижения вычислительных затрат. На протяжении всей книги автор подчеркивает важность понимания процесса технологической эволюции и его влияния на общество.
.Auters propose une nouvelle méthode de randomisation entropique qui permet d'améliorer la précision du modèle et de réduire les coûts de calcul L'idée de base du livre est de fournir une explication accessible et simplifiée du concept de randomisation entropique dans l'apprentissage automatique, un outil puissant pour relever les défis complexes de l'apprentissage automatique et de l'analyse des données. L'auteur souligne l'importance de comprendre le processus d'évolution technologique, y compris le développement de nouvelles connaissances et leur impact sur la société, ainsi que la nécessité d'élaborer un paradigme personnel pour percevoir ce processus comme la base de la survie et de l'unité de l'homme dans un monde de changements technologiques rapides. livre commence par discuter des problèmes de l'apprentissage automatique et de l'analyse des données dans le monde d'aujourd'hui, où la quantité de données augmente de manière exponentielle et la complexité des processus étudiés augmente. L'auteur souligne les limites des méthodes traditionnelles d'apprentissage automatique et la nécessité de nouvelles approches pour relever ces défis. Puis, il introduit la notion de randomisation entropique, qui implique l'utilisation du hasard dans le processus de modélisation pour obtenir les meilleures solutions dans des conditions d'incertitude. L'auteur explique comment fonctionne la randomisation de l'entropie en utilisant des modèles avec des paramètres aléatoires et des estimations maximales de l'entropie des fonctions de densité de probabilité des paramètres du modèle dans des conditions d'équilibre avec les données mesurées. Il donne des exemples de la façon dont cette approche peut être appliquée à différentes tâches d'apprentissage automatique, telles que la classification, la régression et le regroupement. livre couvre également les fondements théoriques de la randomisation entropique, y compris la théorie de l'information et la théorie des probabilités, et montre comment il peut être utilisé pour améliorer la précision du modèle et réduire les coûts de calcul. Tout au long du livre, l'auteur souligne l'importance de comprendre le processus d'évolution technologique et son impact sur la société.
. autores proponen un nuevo método de aleatorización entropical que permite mejorar la precisión del modelo y reducir los costos computacionales La idea básica del libro es dar una explicación accesible y simplificada del concepto de aleatorización entropical en el aprendizaje automático, que es una herramienta poderosa para resolver problemas complejos de aprendizaje automático y análisis de datos. autor destaca la importancia de comprender el proceso de evolución tecnológica, incluyendo el desarrollo de nuevos conocimientos y su impacto en la sociedad, así como la necesidad de generar un paradigma personal para percibir este proceso como la base de la supervivencia y unidad humana en un mundo de rápidos cambios tecnológicos. libro comienza discutiendo los problemas del aprendizaje automático y el análisis de datos en el mundo actual, donde la cantidad de datos crece exponencialmente y la complejidad de los procesos estudiados aumenta. autor subraya las limitaciones de los métodos tradicionales de aprendizaje automático y la necesidad de nuevos enfoques para hacer frente a estos desafíos. Luego, introduce el concepto de aleatorización entropical, que implica el uso de la aleatoriedad en el proceso de modelado para obtener soluciones óptimas en condiciones de incertidumbre. autor explica cómo funciona la aleatorización de la entropía utilizando modelos con parámetros aleatorios y estimaciones máximas de entropía de las funciones de densidad de probabilidad de los parámetros del modelo en condiciones de equilibrio con los datos medidos. Proporciona ejemplos de cómo se puede aplicar este enfoque a diferentes tareas de aprendizaje automático, como la clasificación, regresión y agrupamiento. libro también cubre los fundamentos teóricos de la aleatorización entropical, incluyendo la teoría de la información y la teoría de la probabilidad, y muestra cómo se puede utilizar para mejorar la precisión del modelo y reducir los costos computacionales. A lo largo del libro, el autor destaca la importancia de entender el proceso de evolución tecnológica y su impacto en la sociedad.
Os autores oferecem um novo método de randomização entropia para melhorar a precisão do modelo e reduzir os custos computacionais A ideia básica do livro é fornecer uma explicação acessível e simplificada para o conceito de randomização entropia no aprendizado de máquinas, que é uma ferramenta poderosa para lidar com as complexas tarefas de aprendizagem e análise de dados. O autor ressalta a importância de compreender o processo de evolução tecnológica, incluindo o desenvolvimento de novos conhecimentos e seus efeitos na sociedade, e a necessidade de estabelecer um paradigma pessoal para a percepção deste processo como base para a sobrevivência e unidade humana em um mundo de mudanças tecnológicas rápidas. O livro começa com um debate sobre a aprendizagem de máquinas e análise de dados no mundo atual, onde a quantidade de dados aumenta exponencialmente e a complexidade dos processos estudados aumenta. O autor ressalta a limitação dos métodos tradicionais de aprendizagem de máquinas e a necessidade de novas abordagens para lidar com esses desafios. Em seguida, introduz o conceito de randomização entropia, que envolve o uso do acaso no processo de modelagem para obter as melhores soluções em condições de incerteza. O autor explica como funciona a randomização da entropia usando modelos com parâmetros aleatórios e estimativas máximas da entropia das funções de densidade de probabilidade dos parâmetros do modelo em um equilíbrio com dados medidos. Ele cita exemplos de como esta abordagem pode ser aplicada a várias tarefas de aprendizado de máquina, como classificação, regressão e clusterização. O livro também abrange os fundamentos teóricos da entropia randomização, incluindo a teoria da informação e a teoria da probabilidade, e mostra como ele pode ser usado para melhorar a precisão do modelo e reduzir os custos computacionais. Ao longo do livro, o autor ressalta a importância de compreender o processo de evolução tecnológica e seus efeitos na sociedade.
Gli autori offrono un nuovo metodo di randomizzazione entropica per migliorare l'accuratezza del modello e ridurre i costi di calcolo L'idea di base del libro è quella di fornire una spiegazione accessibile e semplificata del concetto di randomizzazione entropica nell'apprendimento automatico, che è uno strumento potente per affrontare le complesse sfide dell'apprendimento automatico e dell'analisi dei dati. L'autore sottolinea l'importanza di comprendere il processo di evoluzione tecnologica, tra cui lo sviluppo di nuove conoscenze e la loro influenza sulla società, e la necessità di sviluppare un paradigma personale per la percezione di questo processo come base per la sopravvivenza e l'unità umana in un mondo di rapidi cambiamenti tecnologici. Il libro inizia discutendo i problemi dell'apprendimento automatico e dell'analisi dei dati nel mondo moderno, dove il numero di dati cresce esponenzialmente e la complessità dei processi da studiare aumenta. L'autore sottolinea la limitazione dei metodi tradizionali di apprendimento automatico e la necessità di nuovi approcci per affrontare queste sfide. Esso introduce poi il concetto di randomizzazione entropica, che prevede l'utilizzo della casualità nel processo di simulazione per ottenere soluzioni ottimali in condizioni di incertezza. L'autore spiega come funziona la randomizzazione dell'entropia utilizzando modelli con parametri casuali e valutazioni massime dell'entropia delle funzioni di densità dei parametri del modello in equilibrio con i dati misurati. Fornisce esempi di come questo approccio può essere applicato a diverse attività di apprendimento automatico, quali classificazione, regressione e clustering. Il libro comprende anche le basi teoriche della randomizzazione entropica, inclusa la teoria delle informazioni e la teoria delle probabilità, e mostra come può essere utilizzato per migliorare l'accuratezza del modello e ridurre i costi di calcolo. Durante tutto il libro, l'autore sottolinea l'importanza di comprendere l'evoluzione tecnologica e il suo impatto sulla società.
.Die Autoren schlagen eine neue Methode der entropischen Randomisierung vor, um die Genauigkeit des Modells zu verbessern und die Rechenkosten zu senken. Die Grundidee des Buches ist es, eine zugängliche und vereinfachte Erklärung des Konzepts der entropischen Randomisierung im maschinellen rnen zu geben, das ein leistungsfähiges Werkzeug zur Lösung komplexer Probleme des maschinellen rnens und der Datenanalyse ist. Der Autor betont die Bedeutung des Verständnisses des technologischen Evolutionsprozesses, einschließlich der Entwicklung neuen Wissens und seiner Auswirkungen auf die Gesellschaft, sowie die Notwendigkeit, ein persönliches Paradigma für die Wahrnehmung dieses Prozesses als Grundlage für das Überleben und die Einheit des Menschen in einer Welt des schnellen technologischen Wandels zu entwickeln. Das Buch beginnt mit einer Diskussion über die Herausforderungen des maschinellen rnens und der Datenanalyse in der heutigen Welt, in der die Datenmenge exponentiell wächst und die Komplexität der untersuchten Prozesse zunimmt. Der Autor betont die Grenzen traditioneller Methoden des maschinellen rnens und die Notwendigkeit neuer Ansätze zur Lösung dieser Probleme. Dann führt er das Konzept der entropischen Randomisierung ein, die die Verwendung von Zufälligkeit im Modellierungsprozess beinhaltet, um optimale Lösungen unter Bedingungen der Unsicherheit zu erhalten. Der Autor erklärt, wie die Randomisierung der Entropie funktioniert, indem er Modelle mit zufälligen Parametern und maximalen Schätzungen der Entropie der Wahrscheinlichkeitsdichtefunktionen der Modellparameter unter Bilanzbedingungen mit gemessenen Daten verwendet. Er nennt Beispiele, wie dieser Ansatz auf verschiedene Aufgaben des maschinellen rnens wie Klassifizierung, Regression und Clustering angewendet werden kann. Das Buch behandelt auch die theoretischen Grundlagen der entropischen Randomisierung, einschließlich Informationstheorie und Wahrscheinlichkeitstheorie, und zeigt, wie es verwendet werden kann, um die Modellgenauigkeit zu verbessern und die Rechenkosten zu senken. Während des gesamten Buches betont der Autor die Bedeutung des Verständnisses des technologischen Evolutionsprozesses und seiner Auswirkungen auf die Gesellschaft.
Autorzy oferują nową metodę randomizacji entropii w celu poprawy dokładności modelu i zmniejszenia kosztów obliczeniowych Główną ideą książki jest zapewnienie dostępnego i uproszczonego wyjaśnienia pojęcia randomizacji entropii w uczeniu maszynowym, które jest potężnym narzędziem do rozwiązywania złożonych problemów z nauką maszyn i analizą danych. Autor podkreśla znaczenie zrozumienia procesu ewolucji technologicznej, w tym rozwoju nowej wiedzy i jej wpływu na społeczeństwo, a także potrzebę opracowania osobistego paradygmatu postrzegania tego procesu jako podstawy ludzkiego przetrwania i jedności w świecie szybkich zmian technologicznych. Książka rozpoczyna się od omówienia problemów uczenia maszynowego i analizy danych we współczesnym świecie, gdzie ilość danych rośnie wykładniczo, a złożoność badanych procesów rośnie. Autor podkreśla ograniczenia tradycyjnych metod uczenia maszynowego oraz potrzebę nowych podejść do rozwiązywania tych problemów. Następnie wprowadza koncepcję randomizacji entropii, która polega na wykorzystaniu losowości w procesie modelowania w celu uzyskania optymalnych rozwiązań w niepewności. Autor wyjaśnia, w jaki sposób randomizacja entropii działa przy użyciu modeli o losowych parametrach i maksymalnych szacunkach entropii funkcji gęstości prawdopodobieństwa parametrów modelu w warunkach równowagi z mierzonymi danymi. Podaje przykłady tego podejścia do różnych problemów z nauką maszynową, takich jak klasyfikacja, regresja i klastrowanie. Książka obejmuje również teoretyczne podstawy randomizacji entropii, w tym teorię informacji i teorię prawdopodobieństwa, i pokazuje, jak można ją wykorzystać do poprawy dokładności modelu i zmniejszenia kosztów obliczeniowych. W książce autor podkreśla znaczenie zrozumienia procesu ewolucji technologicznej i jej wpływu na społeczeństwo.
סופרים מציעים שיטת אנטרופיה אקראית חדשה לשיפור דיוק המודל ולהפחתת עלויות חישוביות הרעיון העיקרי של הספר הוא לספק הסבר נגיש ומופשט של מושג אנטרופיה אקראית בלמידת מכונה, שהוא כלי רב עוצמה לפתרון בעיות למידת מכונה מורכבות וניתוח נתונים. המחבר מדגיש את חשיבות הבנת תהליך האבולוציה הטכנולוגית, לרבות התפתחות הידע החדש והשפעתו על החברה, וכן את הצורך לפתח פרדיגמה אישית לתפיסת תהליך זה כבסיס להישרדות ולאחדות של האדם בעולם של שינוי טכנולוגי מהיר. הספר מתחיל על ידי דיון בבעיות של למידת מכונה וניתוח נתונים בעולם המודרני, שבו כמות הנתונים גדלה באופן אקספוננציאלי, והמורכבות של התהליכים הנחקרים גדלה. המחבר מדגיש את המגבלות של שיטות לימוד מכונה מסורתיות ואת הצורך בגישות חדשות לפתרון בעיות אלה. לאחר מכן, הוא מציג את הרעיון של אקראיות אנטרופיה, אשר כרוך בשימוש אקראי בתהליך המודול כדי להשיג פתרונות אופטימליים באי ודאות. המחבר מסביר כיצד אנטרופיה אקראית פועלת באמצעות מודלים עם פרמטרים אקראיים והערכות מקסימליות של אנטרופיה של פונקציות צפיפות הסתברות של פרמטרים במודל תחת תנאים של שיווי משקל עם נתונים מדודים. הוא מציג דוגמאות לאופן שבו ניתן ליישם גישה זו על בעיות למידה שונות של מכונה, כגון סיווג, רגרסיה וקיבוצים. הספר גם מכסה את היסודות התאורטיים של אקראיות אנטרופיה, כולל תורת האינפורמציה ותורת ההסתברות, ומראה כיצד ניתן לשפר את דיוק המודל ולהפחית עלויות חישוביות. לאורך הספר מדגיש המחבר את החשיבות של הבנת תהליך האבולוציה הטכנולוגית והשפעתה על החברה.''
Yazarlar, model doğruluğunu artırmak ve hesaplama maliyetlerini azaltmak için yeni bir entropi randomizasyon yöntemi sunar Kitabın ana fikri, karmaşık makine öğrenme problemlerini ve veri analizini çözmek için güçlü bir araç olan makine öğreniminde entropi randomizasyonu kavramının erişilebilir ve basitleştirilmiş bir açıklamasını sağlamaktır. Yazar, yeni bilginin gelişimi ve toplum üzerindeki etkisi de dahil olmak üzere teknolojik evrim sürecini anlamanın önemini ve bu sürecin hızlı bir teknolojik değişim dünyasında insanın hayatta kalması ve birliği için temel olarak algılanması için kişisel bir paradigma geliştirme ihtiyacını vurgulamaktadır. Kitap, veri miktarının katlanarak arttığı ve çalışılan süreçlerin karmaşıklığının arttığı modern dünyada makine öğrenimi ve veri analizi sorunlarını tartışarak başlıyor. Yazar, geleneksel makine öğrenme yöntemlerinin sınırlamalarını ve bu sorunları çözmek için yeni yaklaşımlara duyulan ihtiyacı vurgulamaktadır. Daha sonra, belirsizlikte optimal çözümler elde etmek için modelleme sürecinde rastgelelik kullanmayı içeren entropi randomizasyonu kavramını tanıtır. Yazar, entropi randomizasyonunun rastgele parametrelere sahip modelleri ve ölçülen verilerle denge koşulları altında model parametrelerinin olasılık yoğunluk fonksiyonlarının entropisinin maksimum tahminlerini kullanarak nasıl çalıştığını açıklar. Bu yaklaşımın sınıflandırma, regresyon ve kümeleme gibi çeşitli makine öğrenme problemlerine nasıl uygulanabileceğine dair örnekler verir. Kitap ayrıca bilgi teorisi ve olasılık teorisi de dahil olmak üzere entropi randomizasyonunun teorik temellerini kapsar ve model doğruluğunu artırmak ve hesaplama maliyetlerini azaltmak için nasıl kullanılabileceğini gösterir. Kitap boyunca yazar, teknolojik evrim sürecini ve toplum üzerindeki etkisini anlamanın önemini vurgulamaktadır.
يقدم المؤلفون طريقة جديدة للتوزيع العشوائي للإنتروبيا لتحسين دقة النموذج وتقليل التكاليف الحسابية تتمثل الفكرة الرئيسية للكتاب في تقديم شرح يسهل الوصول إليه ومبسط لمفهوم التوزيع العشوائي للإنتروبيا في التعلم الآلي، وهو أداة قوية لحل مشاكل التعلم الآلي المعقدة وتحليل البيانات. ويشدد المؤلف على أهمية فهم عملية التطور التكنولوجي، بما في ذلك تطوير المعارف الجديدة وأثرها على المجتمع، فضلا عن الحاجة إلى وضع نموذج شخصي لتصور هذه العملية كأساس لبقاء الإنسان ووحدته في عالم يشهد تغيرا تكنولوجيا سريعا. يبدأ الكتاب بمناقشة مشاكل التعلم الآلي وتحليل البيانات في العالم الحديث، حيث تنمو كمية البيانات بشكل كبير، ويتزايد تعقيد العمليات المدروسة. يؤكد المؤلف على قيود أساليب التعلم الآلي التقليدية والحاجة إلى مناهج جديدة لحل هذه المشكلات. بعد ذلك، قدم مفهوم الانتروبي العشوائي، والذي يتضمن استخدام العشوائية في عملية النمذجة للحصول على الحلول المثلى في حالة عدم اليقين. يشرح المؤلف كيف يعمل التوزيع العشوائي للإنتروبيا باستخدام نماذج ذات معلمات عشوائية وتقديرات قصوى لوظائف كثافة الاحتمالات لمعلمات النموذج في ظل ظروف التوازن مع البيانات المقاسة. يقدم أمثلة على كيفية تطبيق هذا النهج على العديد من مشاكل التعلم الآلي مثل التصنيف والانحدار والتكتل. يغطي الكتاب أيضًا الأسس النظرية للإنتروبيا العشوائية، بما في ذلك نظرية المعلومات ونظرية الاحتمالات، ويوضح كيف يمكن استخدامها لتحسين دقة النموذج وتقليل التكاليف الحسابية. في جميع أنحاء الكتاب، يؤكد المؤلف على أهمية فهم عملية التطور التكنولوجي وتأثيرها على المجتمع.
저자는 모델 정확도를 향상시키고 계산 비용을 줄이기위한 새로운 엔트로피 무작위 화 방법을 제공합니다.이 책의 주요 아이디어는 기계 학습의 엔트로피 무작위 화 개념에 대한 액세스 가능하고 단순화 된 설명을 제공하는 것입니다. 저자는 새로운 지식의 개발과 사회에 미치는 영향을 포함하여 기술 진화 과정을 이해하는 것의 중요성뿐만 아니라이 과정을 인간의 생존과 통일의 기초로 인식하기위한 개인적인 패러다임을 개발할 필요성을 강조합니다. 빠른 기술 변화의 세계. 이 책은 데이터의 양이 기하 급수적으로 증가하고 연구 프로세스의 복잡성이 증가하는 현대 세계의 머신 러닝 및 데이터 분석 문제에 대해 논의함으로써 시작됩니다. 저자는 전통적인 머신 러닝 방법의 한계와 이러한 문제를 해결하기위한 새로운 접근 방식의 필요성을 강조합니다. 그런 다음 엔트로피 무작위 화의 개념을 소개합니다. 엔트로피 무작위 화는 모델링 프로세스에서 무작위성을 사용하여 불확실성에서 최적의 솔루션을 얻습니다. 저자는 엔트로피 무작위 화가 임의의 매개 변수를 가진 모델과 측정 된 데이터와 균형 조건 하에서 모델 매개 변수의 확률 밀도 함수 엔트로피의 최대 추정치를 사용하여 어떻게 작동하는지 설명합 그는이 접근 방식이 분류, 회귀 및 클러스터링과 같은 다양한 머신 러닝 문제에 어떻게 적용될 수 있는지에 대한 예를 제공합니다. 이 책은 또한 정보 이론 및 확률 이론을 포함하여 엔트로피 무작위 화의 이론적 기초를 다루고 모델 정확도를 향상시키고 계산 비용을 줄이는 데 어떻게 사용될 수 있는지 보여줍니다. 이 책 전체에서 저자는 기술 진화 과정과 사회에 미치는 영향을 이해하는 것의 중요성을 강조합니다.
著者は、モデルの精度を向上させ、計算コストを削減するための新しいエントロピー・ランダム化方法を提供している。この本の主な考え方は、複雑な機械学習の問題とデータ分析を解決するための強力なツールである機械学習におけるエントロピー・ランダム化の概念について、アクセスしやすく簡単に説明することである。著者は、新しい知識の開発と社会への影響を含む技術進化のプロセスを理解することの重要性と、急速な技術変化の世界における人間の生存と団結の基礎としてこのプロセスの認識のための個人的なパラダイムを開発する必要性を強調しています。この本は、データ量が指数関数的に増加し、研究プロセスの複雑さが増している現代の世界における機械学習とデータ分析の問題を議論することから始まります。著者は、従来の機械学習方法の限界と、これらの問題を解決するための新しいアプローチの必要性を強調しています。その後、モデリング過程でランダム性を用いて不確実性の中で最適な解を得ることを含むエントロピー・ランダム化の概念を導入する。ランダムパラメータを持つモデルを用いてエントロピーのランダム化がどのように機能するか、また、測定データとのバランス条件下でのモデルパラメータの確率密度関数のエントロピーの最大推定値を用いて説明する。このアプローチが、分類、回帰、クラスタリングなどのさまざまな機械学習の問題にどのように適用できるかの例を示しています。また、情報理論や確率理論を含むエントロピーのランダム化の理論的基礎についても取り上げ、モデル精度の向上と計算コストの削減にどのように役立つかを示している。著者は本書を通じて、技術進化の過程を理解し、社会に与える影響の重要性を強調している。
. Autors提出了一種新的熵隨機化方法,可以提高模型準確性並降低計算成本。本書的基本思想是對機器學習中的熵隨機化概念提供可用且簡化的解釋,這是解決復雜機器學習和數據分析問題的有力工具。作者強調了理解技術演變過程的重要性,包括新知識的發展及其對社會的影響,以及有必要建立個人範式,將過程視為人類在快速技術變革世界中生存和團結的基礎。本書首先討論了現代世界中的機器學習和數據分析問題,其中數據數量呈指數增長,研究過程的復雜性增加。作者強調了傳統機器學習方法的局限性,以及應對這些挑戰的新方法的必要性。然後,他介紹了熵隨機化的概念,該概念涉及在模擬過程中使用隨機性以在不確定性條件下獲得最佳解。作者通過使用具有隨機參數和模型參數概率密度函數的最大熵估計的模型來解釋熵隨機化是如何在與測量數據平衡的情況下工作的。他舉例說明了如何將這種方法應用於不同的機器學習任務,例如分類,回歸和聚類。該書還涵蓋了熵隨機化的理論基礎,包括信息理論和概率論,並展示了如何將其用於提高模型準確性和降低計算成本。在整個書中,作者強調了了解技術進化過程及其對社會影響的重要性。
