
BOOKS - PROGRAMMING - Dive into Deep Learning (Release 0.16.6)

Dive into Deep Learning (Release 0.16.6)
Author: Aston Zhang, Zachary C. Lipton, Mu Li, Alexander J. Smola
Year: 2021
Pages: 1029
Format: PDF
File size: 27,3 MB
Language: ENG
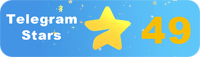
Year: 2021
Pages: 1029
Format: PDF
File size: 27,3 MB
Language: ENG
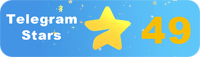
''
