
BOOKS - PROGRAMMING - Deep Learning with Python, 2nd Edition

Deep Learning with Python, 2nd Edition
Author: Francios Chollet
Year: 2021
Pages: 504
Format: PDF
File size: 14 MB
Language: ENG
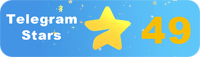
Year: 2021
Pages: 504
Format: PDF
File size: 14 MB
Language: ENG
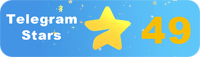
with this comprehensive guide. Long Description: The second edition of Deep Learning with Python provides an in-depth introduction to the most recent advancements in deep learning methods and their applications using Python. This book covers the full range of deep learning, from the basics of neural networks to the most advanced topics such as segmentation, scene understanding, and generative models. It is written in an approachable style with numerous illustrations and code examples to help you quickly grasp the ideas and implement them effectively. The book has been completely updated to cover the most recent developments in deep learning, including the use of transformers, convolutional neural networks (CNNs), recurrent neural networks (RNNs), long short-term memory (LSTM) networks, autoencoders, generative adversarial networks (GANs), and more. You will learn how to put these methods into practice with Python code examples and projects. With the advent of technology, we have reached a point where our survival depends on our ability to adapt and evolve alongside it. As humans, we must recognize the significance of developing a personal paradigm for perceiving the technological process of creating modern knowledge and how it affects our survival and the unification of people in a warring state. In this context, studying deep learning with Python can be considered a crucial step toward achieving this goal. Deep learning is an area of artificial intelligence that focuses on developing algorithms capable of analyzing large amounts of data and recognizing patterns within them. This capability can be used in various applications such as image and speech recognition, natural language processing, and predictive modeling. Python is a popular programming language used in deep learning due to its simplicity and flexibility. It provides a wide range of libraries and frameworks, including TensorFlow, Keras, PyTorch, and scikit-learn, that simplify the process of implementing and experimenting with different deep learning models.
с этим всеобъемлющим руководством. Long Description: Второе издание Deep arning with Python содержит подробное введение в самые последние достижения в методах глубокого обучения и их приложениях с использованием Python. Эта книга охватывает весь спектр глубокого обучения, от основ нейронных сетей до самых передовых тем, таких как сегментация, понимание сцены и генеративные модели. Он написан в доступном стиле с многочисленными иллюстрациями и примерами кода, которые помогут вам быстро понять идеи и эффективно их реализовать. Книга была полностью обновлена, чтобы охватить самые последние разработки в области глубокого обучения, включая использование трансформаторов, сверточных нейронных сетей (CNN), рекуррентных нейронных сетей (RNN), сетей длительной краткосрочной памяти (LSTM), автоэнкодеров, генеративных состязательных сетей (GAN) и многое другое. О том, как применять эти методы на практике, вы узнаете из примеров кода на Python и проектов. С появлением технологий мы достигли точки, когда наше выживание зависит от нашей способности адаптироваться и развиваться вместе с ним. Как люди, мы должны признать значимость выработки личностной парадигмы восприятия технологического процесса создания современных знаний и того, как он влияет на наше выживание и объединение людей в воюющем государстве. В этом контексте изучение глубокого обучения с помощью Python можно считать решающим шагом на пути к достижению этой цели. Глубокое обучение - это область искусственного интеллекта, которая фокусируется на разработке алгоритмов, способных анализировать большие объемы данных и распознавать закономерности внутри них. Эта возможность может использоваться в различных приложениях, таких как распознавание изображений и речи, обработка естественного языка и прогнозное моделирование. Python - популярный язык программирования, используемый в глубоком обучении благодаря своей простоте и гибкости. Он предоставляет широкий спектр библиотек и фреймворков, включая TensorFlow, Keras, PyTorch и scikit-learn, которые упрощают процесс внедрения и экспериментирования с различными моделями глубокого обучения.
avec ce guide complet. Description longue : La deuxième édition de Deep arning with Python contient une introduction détaillée aux dernières avancées des techniques d'apprentissage profond et de leurs applications en utilisant Python. Ce livre couvre toute la gamme de l'apprentissage profond, des bases des réseaux neuronaux aux sujets les plus avancés tels que la segmentation, la compréhension de la scène et les modèles génératifs. Il est écrit dans un style abordable avec de nombreuses illustrations et exemples de code qui vous aideront à comprendre rapidement les idées et à les mettre en œuvre efficacement. livre a été entièrement mis à jour pour couvrir les développements les plus récents dans le domaine de l'apprentissage profond, y compris l'utilisation de transformateurs, de réseaux neuronaux convolutifs (CNN), de réseaux neuronaux récurrents (RNN), de réseaux de mémoire à long terme (LSTM), d'encodeurs automatiques, de réseaux de compétition générative (GAN) et bien plus encore. Vous apprendrez comment mettre ces méthodes en pratique à partir d'exemples de code sur Python et de projets. Avec l'avènement de la technologie, nous sommes arrivés à un point où notre survie dépend de notre capacité à nous adapter et à évoluer avec elle. En tant qu'êtres humains, nous devons reconnaître l'importance d'élaborer un paradigme personnel pour la perception du processus technologique de la création de connaissances modernes et la façon dont il affecte notre survie et l'unification des personnes dans un État en guerre. Dans ce contexte, l'apprentissage profond avec Python peut être considéré comme une étape décisive vers la réalisation de cet objectif. L'apprentissage profond est un domaine de l'intelligence artificielle qui se concentre sur le développement d'algorithmes capables d'analyser de grandes quantités de données et de reconnaître les schémas en eux. Cette possibilité peut être utilisée dans diverses applications telles que la reconnaissance d'images et de la parole, le traitement du langage naturel et la modélisation prédictive. Python est un langage de programmation populaire utilisé dans l'apprentissage profond en raison de sa simplicité et de sa flexibilité. Il propose un large éventail de bibliothèques et de cadres, dont TensorFlow, Keras, PyTorch et scikit-learn, qui simplifient la mise en œuvre et l'expérimentation de différents modèles d'apprentissage profond.
con esta guía integral. Long Description: La segunda edición de Deep arning with Python contiene una introducción detallada a los avances más recientes en técnicas de aprendizaje profundo y sus aplicaciones utilizando Python. Este libro abarca todo el espectro del aprendizaje profundo, desde los fundamentos de las redes neuronales hasta los temas más avanzados como la segmentación, la comprensión de la escena y los modelos generativos. Está escrito en un estilo asequible con numerosas ilustraciones y ejemplos de código que le ayudarán a entender rápidamente las ideas y a implementarlas de manera efectiva. libro ha sido completamente actualizado para abarcar los desarrollos más recientes en el campo del aprendizaje profundo, incluyendo el uso de transformadores, redes neuronales perforadas (CNN), redes neuronales recurrativas (RNN), redes de memoria a corto plazo (LSTM), codificadores automáticos, redes competitivas generadoras (GAN) y más. Aprenderá sobre cómo poner en práctica estos métodos a partir de ejemplos de código en Python y proyectos. Con la llegada de la tecnología hemos llegado a un punto en el que nuestra supervivencia depende de nuestra capacidad para adaptarnos y evolucionar con ella. Como seres humanos, debemos reconocer la importancia de generar un paradigma personal para percibir el proceso tecnológico de crear conocimiento moderno y cómo afecta nuestra supervivencia y la unión de las personas en un estado en guerra. En este contexto, el estudio del aprendizaje profundo con Python puede considerarse un paso decisivo hacia el logro de este objetivo. aprendizaje profundo es un campo de inteligencia artificial que se centra en el desarrollo de algoritmos capaces de analizar grandes cantidades de datos y reconocer patrones dentro de ellos. Esta capacidad se puede utilizar en diferentes aplicaciones, como reconocimiento de imágenes y voz, procesamiento de lenguaje natural y simulación predictiva. Python es un lenguaje de programación popular utilizado en el aprendizaje profundo debido a su simplicidad y flexibilidad. Ofrece una amplia gama de bibliotecas y marcos, incluyendo TensorFlow, Keras, PyTorch y scikit-learn, que simplifican el proceso de implementación y experimentación con diferentes modelos de aprendizaje profundo.
com este manual completo. Long Descrição: A segunda edição do Deep arning with Python traz uma introdução detalhada aos avanços mais recentes dos métodos de aprendizagem profunda e seus aplicativos com Python. Este livro abrange toda a gama de aprendizagens profundas, desde os fundamentos das redes neurais até os temas mais avançados, como segmentação, compreensão da cena e modelos genéricos. Ele é escrito em um estilo acessível, com inúmeras ilustrações e exemplos de código que podem ajudá-lo a compreender rapidamente as ideias e realizá-las de forma eficaz. O livro foi totalmente atualizado para cobrir os mais recentes desenvolvimentos de aprendizagem profunda, incluindo transformadores, redes neurais personalizadas (CNN), redes neurais recorrentes (RNN), redes de memória de curto prazo (LSTM), encodedores automáticos, redes de competição genérica (GAN) e muito mais. Sobre como aplicar estes métodos na prática, você aprenderá a partir de exemplos de código em Python e projetos. Com a tecnologia, chegamos a um ponto em que a nossa sobrevivência depende da nossa capacidade de se adaptar e evoluir com ela. Como seres humanos, devemos reconhecer a importância de criar um paradigma pessoal para a percepção do processo tecnológico de criação do conhecimento moderno e como ele afeta a nossa sobrevivência e a união das pessoas num estado em guerra. Nesse contexto, estudar a aprendizagem profunda com Python pode ser considerado um passo crucial para alcançar este objetivo. O aprendizado profundo é uma área de inteligência artificial que se concentra no desenvolvimento de algoritmos capazes de analisar grandes quantidades de dados e reconhecer padrões dentro deles. Esta capacidade pode ser usada em vários aplicativos, como reconhecimento de imagem e voz, processamento de linguagem natural e simulação de previsão. Python é uma linguagem popular de programação usada no aprendizado profundo por sua simplicidade e flexibilidade. Oferece uma ampla gama de bibliotecas e quadros, incluindo TensorFlow, Keras, PyTorch e scikit-learn, que simplificam o processo de implementação e experimentação com diferentes modelos de aprendizagem profunda.
con questa guida completa. Long Communication: La seconda edizione di Deep arning with Python contiene un'introduzione dettagliata ai più recenti progressi nei metodi di apprendimento approfondito e nelle loro applicazioni con Python. Questo libro comprende un'ampia gamma di apprendimenti, dalle basi delle reti neurali ai temi più avanzati, come la segmentazione, la comprensione della scena e i modelli generali. È scritto in uno stile accessibile con numerose illustrazioni e esempi di codice per aiutarvi a comprendere rapidamente le idee e realizzarle efficacemente. Il libro è stato completamente aggiornato per coprire i più recenti sviluppi di formazione profonda, tra cui l'uso di trasformatori, reti neurali compresse (CNN), reti neurali ricettive (RNN), reti di memoria a breve termine (LSTM), autocoder, reti di competizione generative (GAN) e molto altro ancora. Su come applicare questi metodi in pratica si imparerà da esempi di codice su Python e progetti. Con l'avvento della tecnologia, siamo arrivati ad un punto in cui la nostra sopravvivenza dipende dalla nostra capacità di adattarci e svilupparci con essa. Come esseri umani, dobbiamo riconoscere l'importanza di sviluppare un paradigma personale per la percezione del processo tecnologico per la creazione di conoscenze moderne e il modo in cui esso influisce sulla nostra sopravvivenza e sull'unione delle persone in uno stato in guerra. In questo contesto, l'apprendimento approfondito con Python può essere considerato un passo decisivo verso questo obiettivo. L'apprendimento approfondito è un campo di intelligenza artificiale che si concentra sullo sviluppo di algoritmi in grado di analizzare e riconoscere grandi quantità di dati. Questa funzionalità può essere utilizzata in diverse applicazioni, come il riconoscimento di immagini e vocali, l'elaborazione del linguaggio naturale e la simulazione predittiva. Python è un linguaggio di programmazione popolare, utilizzato nell'apprendimento profondo grazie alla sua semplicità e flessibilità. Offre una vasta gamma di librerie e framework, tra cui TensorFlow, Keras, PyTorch e scikit-learn, che semplificano l'implementazione e la sperimentazione di diversi modelli di apprendimento approfondito.
mit diesem umfassenden itfaden. Lange Beschreibung: Die zweite Ausgabe von Deep arning with Python bietet eine detaillierte Einführung in die neuesten Fortschritte bei Deep arning-Techniken und deren Anwendungen mit Python. Dieses Buch deckt das gesamte Spektrum des Deep arning ab, von den Grundlagen neuronaler Netzwerke bis hin zu den fortschrittlichsten Themen wie Segmentierung, Szenenverständnis und generative Modelle. Es ist in einem zugänglichen Stil mit zahlreichen Illustrationen und Codebeispielen geschrieben, die Ihnen helfen, Ideen schnell zu verstehen und effektiv umzusetzen. Das Buch wurde vollständig aktualisiert, um die neuesten Entwicklungen im Bereich Deep arning abzudecken, einschließlich der Verwendung von Transformatoren, Convolutional Neural Networks (CNNs), Recurrent Neural Networks (RNNs), Long-Term Short Memory Networks (LSTMs), Auto-Encodern, generativen Contracting Networks (GANs) und mehr. Wie e diese Techniken in der Praxis anwenden, erfahren e anhand von Python-Codebeispielen und Projekten. Mit dem Aufkommen der Technologie haben wir einen Punkt erreicht, an dem unser Überleben von unserer Fähigkeit abhängt, uns anzupassen und uns mit ihr zu entwickeln. Als Menschen müssen wir die Bedeutung der Entwicklung eines persönlichen Paradigmas der Wahrnehmung des technologischen Prozesses der Schaffung modernen Wissens erkennen und wie es unser Überleben und die Vereinigung von Menschen in einem kriegführenden Staat beeinflusst. In diesem Zusammenhang kann das Erlernen von Deep arning mit Python als entscheidender Schritt zur Erreichung dieses Ziels angesehen werden. Deep arning ist ein Bereich der künstlichen Intelligenz, der sich auf die Entwicklung von Algorithmen konzentriert, die in der Lage sind, große Datenmengen zu analysieren und Muster in ihnen zu erkennen. Diese Fähigkeit kann in einer Vielzahl von Anwendungen wie Bild- und Spracherkennung, natürlicher Sprachverarbeitung und prädiktiver Modellierung eingesetzt werden. Python ist eine beliebte Programmiersprache, die aufgrund ihrer Einfachheit und Flexibilität im Deep arning verwendet wird. Es bietet eine breite Palette von Bibliotheken und Frameworks, darunter TensorFlow, Keras, PyTorch und scikit-learn, die den Prozess der Implementierung und des Experimentierens mit verschiedenen Deep-arning-Modellen vereinfachen.
z tym kompleksowym przewodnikiem. Długi opis: Druga edycja Deep arning z Pythonem zawiera szczegółowe wprowadzenie do najnowszych postępów w technice głębokiego uczenia się i ich zastosowań za pomocą Pythona. Ta książka obejmuje pełne spektrum głębokiego uczenia się, od fundamentów sieci neuronowych po najbardziej zaawansowane tematy, takie jak segmentacja, zrozumienie sceny i modele generacyjne. Jest napisany w dostępnym stylu z licznymi ilustracjami i przykładami kodów, aby pomóc Ci szybko zrozumieć pomysły i skutecznie je wdrożyć. Książka została całkowicie zaktualizowana, aby objąć najnowsze wydarzenia w dziedzinie głębokiego uczenia się, w tym wykorzystanie transformatorów, konwolucyjnych sieci neuronowych (CNN), powtarzających się sieci neuronowych (RNN), długoterminowych sieci pamięci krótkoterminowej (LSTM), autoenkoderów, generacyjnych sieci przeciwnych (GAN) i więcej. Dowiesz się, jak wprowadzić te metody w życie na podstawie przykładów kodu Pythona i projektów. Wraz z pojawieniem się technologii osiągnęliśmy punkt, w którym nasze przetrwanie zależy od naszej zdolności adaptacji i ewolucji. Jako ludzie musimy uznać znaczenie rozwijania osobistego paradygmatu postrzegania technologicznego procesu tworzenia nowoczesnej wiedzy i tego, jak wpływa ona na nasze przetrwanie i zjednoczenie ludzi w stanie wojennym. W tym kontekście naukę głębokiego uczenia się z Pythonem można uznać za kluczowy krok w kierunku osiągnięcia tego celu. Głębokie uczenie się jest obszarem sztucznej inteligencji, która koncentruje się na rozwijaniu algorytmów zdolnych do analizy dużych ilości danych i rozpoznawania w nich wzorców. Możliwość ta może być wykorzystywana w różnych zastosowaniach, takich jak rozpoznawanie obrazu i mowy, przetwarzanie języka naturalnego i modelowanie predykcyjne. Python jest popularnym językiem programowania używanym w głębokiej nauce ze względu na prostotę i elastyczność. Oferuje szeroką gamę bibliotek i ram, w tym TensorFlow, Keras, PyTorch i scikit-learn, które upraszczają proces wdrażania i eksperymentowania z różnymi modelami głębokiego uczenia się.
עם המדריך המקיף הזה. תיאור ארוך: המהדורה השנייה של למידה עמוקה עם פייתון מכילה הקדמה מפורטת להתקדמות האחרונה בטכניקות למידה עמוקה ויישומיהם באמצעות פייתון. ספר זה מכסה את כל הספקטרום של למידה עמוקה, מהיסודות של רשתות עצביות ועד לנושאים המתקדמים ביותר כמו קטגוריות, הבנת זירות ומודלים מחוללים. הוא נכתב בסגנון נגיש עם איורים רבים ודוגמאות קוד כדי לעזור לך במהירות להבין רעיונות וליישם אותם ביעילות. הספר עודכן לחלוטין כדי לסקור את ההתפתחויות האחרונות בלמידה עמוקה, כולל שימוש בשנאים, רשתות עצביות קונבנציונליות (ALL), רשתות עצביות חוזרות ונשנות (RNNs), רשתות זיכרון לטווח קצר (LSTM), רשתות אוטואנקודרים (Autoencoders), רשתות אדברסריות מחוללות (GANs) ועוד. אתם תלמדו איך ליישם את השיטות האלה מדוגמאות של קוד פייתון ופרויקטים. עם הופעת הטכנולוגיה, הגענו לנקודה בה ההישרדות שלנו תלויה ביכולת שלנו להסתגל ולהתפתח איתה. כאנשים, עלינו להכיר בחשיבות של פיתוח פרדיגמה אישית לתפיסה של התהליך הטכנולוגי של יצירת ידע מודרני וכיצד הוא משפיע על הישרדותנו ועל איחוד האנשים במדינה לוחמת. בהקשר זה, למידה מעמיקה עם פייתון יכולה להיחשב כצעד מכריע להשגת מטרה זו. למידה עמוקה היא תחום בבינה מלאכותית המתמקד בפיתוח אלגוריתמים המסוגלים לנתח כמויות גדולות של נתונים ולזהות תבניות בתוכו. יכולת זו יכולה לשמש במגוון יישומים כגון זיהוי תמונה ודיבור, עיבוד שפה טבעית ודוגמנות חיזוי. פייתון (באנגלית: Python) היא שפת תכנות פופולרית המשמשת ללמידה עמוקה בשל פשטותה וגמישותה. היא מספקת מגוון רחב של ספריות ומסגרות, כולל TensorFlow, Kras, PyTorch ו-Scikit-arch, המפשטות את תהליך היישום והניסוי של מודלים למידה מעמיקה שונים.''
bu kapsamlı kılavuz ile. Uzun Açıklama: Python ile Derin Öğrenmenin ikinci baskısı, derin öğrenme tekniklerindeki en son gelişmelere ve Python kullanan uygulamalarına ayrıntılı bir giriş içerir. Bu kitap, sinir ağlarının temellerinden segmentasyon, sahne anlayışı ve üretken modeller gibi en gelişmiş konulara kadar derin öğrenmenin tüm spektrumunu kapsar. Fikirleri hızlı bir şekilde anlamanıza ve etkili bir şekilde uygulamanıza yardımcı olmak için çok sayıda illüstrasyon ve kod örneği içeren erişilebilir bir tarzda yazılmıştır. Kitap, transformatörler, konvolüsyonel sinir ağları (CNN'ler), tekrarlayan sinir ağları (RNN'ler), uzun süreli kısa süreli bellek (LSTM) ağları, otoenkoderler, üretken düşmanca ağlar (GAN'lar) ve daha fazlası dahil olmak üzere derin öğrenmedeki en son gelişmeleri kapsayacak şekilde tamamen güncellenmiştir. Bu yöntemleri Python kod ve proje örneklerinden nasıl uygulamaya koyacağınızı öğreneceksiniz. Teknolojinin gelişiyle, hayatta kalmamızın onunla uyum sağlama ve gelişme yeteneğimize bağlı olduğu bir noktaya ulaştık. İnsanlar olarak, modern bilgiyi yaratmanın teknolojik sürecinin algılanması için kişisel bir paradigma geliştirmenin önemini ve bunun hayatta kalmamızı ve savaşan bir durumda insanların birleşmesini nasıl etkilediğini kabul etmeliyiz. Bu bağlamda, Python ile derin öğrenmeyi öğrenmek, bu hedefe ulaşmak için çok önemli bir adım olarak düşünülebilir. Derin öğrenme, büyük miktarda veriyi analiz edebilen ve içindeki kalıpları tanıyabilen algoritmalar geliştirmeye odaklanan bir yapay zeka alanıdır. Bu yetenek, görüntü ve konuşma tanıma, doğal dil işleme ve tahmini modelleme gibi çeşitli uygulamalarda kullanılabilir. Python, basitliği ve esnekliği nedeniyle derin öğrenmede kullanılan popüler bir programlama dilidir. Çeşitli derin öğrenme modellerini uygulama ve deneme sürecini basitleştiren TensorFlow, Keras, PyTorch ve scikit-learn dahil olmak üzere çok çeşitli kütüphaneler ve çerçeveler sunar.
مع هذا الدليل الشامل. الوصف الطويل: يحتوي الإصدار الثاني من Deep arning with Python على مقدمة مفصلة لأحدث التطورات في تقنيات التعلم العميق وتطبيقاتها باستخدام Python. يغطي هذا الكتاب الطيف الكامل للتعلم العميق، من أسس الشبكات العصبية إلى الموضوعات الأكثر تقدمًا مثل التجزئة وفهم المشهد والنماذج التوليدية. إنه مكتوب بأسلوب يمكن الوصول إليه مع العديد من الرسوم التوضيحية وأمثلة التعليمات البرمجية لمساعدتك على فهم الأفكار بسرعة وتنفيذها بشكل فعال. تم تحديث الكتاب بالكامل لتغطية أحدث التطورات في التعلم العميق، بما في ذلك استخدام المحولات، والشبكات العصبية التلافيفية (CNNs)، والشبكات العصبية المتكررة (RNNs)، وشبكات الذاكرة قصيرة المدى (LSTM)، وأجهزة التشفير الذاتي، وشبكات الخصومة التوليدية (GANs)، و أكثر. ستتعلم كيفية وضع هذه الأساليب موضع التنفيذ من أمثلة على كود ومشاريع بايثون. مع ظهور التكنولوجيا، وصلنا إلى نقطة يعتمد فيها بقاؤنا على قدرتنا على التكيف والتطور معها. بصفتنا بشرًا، يجب أن ندرك أهمية تطوير نموذج شخصي لتصور العملية التكنولوجية لخلق المعرفة الحديثة وكيف تؤثر على بقاءنا وتوحيد الناس في دولة متحاربة. في هذا السياق، يمكن اعتبار تعلم التعلم العميق مع Python خطوة حاسمة نحو تحقيق هذا الهدف. التعلم العميق هو مجال من مجالات الذكاء الاصطناعي يركز على تطوير خوارزميات قادرة على تحليل كميات كبيرة من البيانات والتعرف على الأنماط داخلها. يمكن استخدام هذه القدرة في مجموعة متنوعة من التطبيقات مثل التعرف على الصورة والكلام ومعالجة اللغة الطبيعية والنمذجة التنبؤية. Python هي لغة برمجة شائعة تستخدم في التعلم العميق نظرًا لبساطتها ومرونتها. يوفر مجموعة واسعة من المكتبات والأطر، بما في ذلك TensorFlow و Keras و PyTorch و scikit-learn، التي تبسط عملية تنفيذ وتجربة العديد من نماذج التعلم العميق.
이 포괄적 인 가이드와 함께. 긴 설명: Python을 사용한 Deep arning의 두 번째 버전에는 가장 최근의 딥 러닝 기술 및 Python을 사용한 응용 프로그램에 대한 자세한 소개가 포함되어 있습니다. 이 책은 신경망의 기초에서 세분화, 장면 이해 및 생성 모델과 같은 가장 진보 된 주제에 이르기까지 모든 딥 러닝을 다룹니다. 아이디어를 빠르게 이해하고 효과적으로 구현할 수 있도록 수많은 삽화와 코드 예제가 포함 된 액세스 가능한 스타일로 작성되었 이 책은 변압기, 컨볼 루션 신경망 (CNN), 재귀 신경망 (RNN), 장기 단기 메모리 (LSTM) 네트워크, 자동 인코더, 생성 적대적 네트워크 (GANs) 등 파이썬 코드 및 프로젝트의 예에서 이러한 방법을 실행하는 방법을 배웁니다. 기술의 출현으로 우리는 생존이 적응하고 발전시키는 능력에 달려있는 시점에 도달했습니다. 우리는 현대 지식을 창출하는 기술 과정에 대한 인식과 그것이 우리의 생존과 전쟁 상태에있는 사람들의 통일에 어떤 영향을 미치는지에 대한 개인적인 패러다임을 개발하는 것의 중요성을 인식해야합니다. 이러한 맥락에서 Python으로 딥 러닝을 배우는 것이이 목표를 달성하기위한 중요한 단계로 간주 될 수 있습니 딥 러닝은 많은 양의 데이터를 분석하고 그 안에 패턴을 인식 할 수있는 알고리즘 개발에 중점을 둔 인공 지능 영역입니다. 이 기능은 이미지 및 음성 인식, 자연어 처리 및 예측 모델링과 같은 다양한 응용 분야에서 사용할 수 있습니다. Python은 단순성과 유연성으로 인해 딥 러닝에 사용되는 인기있는 프로그래밍 언어입니다. TensorFlow, Keras, PyTorch 및 scikit-learn을 포함한 광범위한 라이브러리 및 프레임 워크를 제공하여 다양한 딥 러닝 모델을 구현하고 실험하는 프로세스를 단순화합니다.
