
BOOKS - PROGRAMMING - Deep Learning from first principles Second Edition In vectorize...

Deep Learning from first principles Second Edition In vectorized Python, R and Octave
Author: Tinniam V. Ganesh
Year: 2018
Pages: 770
Format: EPUB | PDF CONV
File size: 10.4 MB
Language: ENG
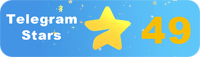
Year: 2018
Pages: 770
Format: EPUB | PDF CONV
File size: 10.4 MB
Language: ENG
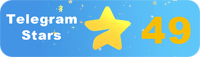
''
