
BOOKS - Data-Centric Artificial Intelligence for Multidisciplinary Applications

Data-Centric Artificial Intelligence for Multidisciplinary Applications
Author: Parikshit N. Mahalle, Namrata N. Wasatkar, Gitanjali R. Shinde
Year: 2024
Pages: 309
Format: PDF
File size: 19.4 MB
Language: ENG
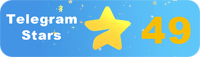
Year: 2024
Pages: 309
Format: PDF
File size: 19.4 MB
Language: ENG
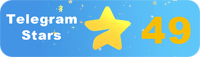
DataCentric Artificial Intelligence for Multidisciplinary Applications: A Paradigm Shift in AI Development In today's rapidly evolving technological landscape, the field of Artificial Intelligence (AI) is undergoing a significant transformation. The traditional model-centric approach, where AI models are built based on predefined algorithms and rules, is being challenged by a new paradigm - the DataCentric approach. This shift in perspective emphasizes the importance of high-quality data and its role in developing and implementing AI systems. The book "DataCentric Artificial Intelligence for Multidisciplinary Applications" explores the need for a DataCentric AI approach and its application in various domains, highlighting the benefits and potential of this paradigm shift. The Need for a DataCentric Approach The traditional model-centric approach to AI has been successful in solving specific problems within well-defined domains. However, as AI applications expand into more complex and multidisciplinary areas, the limitations of this approach become increasingly evident. The DataCentric approach offers a more comprehensive and flexible way of building AI systems that can adapt to changing data and environments. By focusing on data quality and analysis, DataCentric AI can provide more accurate and reliable outcomes, making it an essential tool for addressing the challenges of the future.
DataCentric Artificial Intelligence for Multidisciplinary Applications: A Paradigm Shift in AI Development В современном быстро развивающемся технологическом ландшафте сфера искусственного интеллекта (ИИ) переживает значительную трансформацию. Традиционный модельно-ориентированный подход, где модели ИИ строятся на основе заранее заданных алгоритмов и правил, оспаривается новой парадигмой - подходом DataCentric. Этот сдвиг в перспективе подчеркивает важность высококачественных данных и их роль в разработке и внедрении систем ИИ. Книга «DataCentric Artificial Intelligence for Multidisciplinary Applications» исследует необходимость подхода DataCentric AI и его применения в различных областях, подчеркивая преимущества и потенциал этой смены парадигмы. Потребность в подходе, ориентированном на данные Традиционный подход к ИИ, ориентированный на модели, успешно решает конкретные проблемы в четко определенных областях. Однако по мере того, как приложения ИИ расширяются в более сложные и междисциплинарные области, ограничения этого подхода становятся все более очевидными. Подход DataCentric предлагает более полный и гибкий способ создания систем искусственного интеллекта, которые могут адаптироваться к изменяющимся данным и средам. Сосредоточив внимание на качестве и анализе данных, DataCentric AI может обеспечить более точные и надежные результаты, что делает его важным инструментом для решения проблем будущего.
DataCentric Artificial Intelligence for Multidisciplinary Applications : A Paradigm Shift in AI Development Dans le paysage technologique en évolution rapide d'aujourd'hui, le domaine de l'intelligence artificielle (IA) connaît une transformation importante. L'approche modélisée traditionnelle, où les modèles d'IA sont construits sur la base d'algorithmes et de règles prédéfinis, est contestée par un nouveau paradigme, DataCentric. Ce changement de perspective souligne l'importance des données de haute qualité et leur rôle dans la conception et la mise en œuvre des systèmes d'IA. livre « DataCentric Artificial Intelligence for Multidisciplinary Applications » explore la nécessité de l'approche DataCentric AI et de son application dans différents domaines, en soulignant les avantages et le potentiel de ce changement de paradigme. Nécessité d'une approche axée sur les données L'approche traditionnelle de l'IA axée sur les modèles répond avec succès à des problèmes spécifiques dans des domaines bien définis. Cependant, à mesure que les applications de l'IA se développent dans des domaines plus complexes et interdisciplinaires, les limites de cette approche deviennent de plus en plus évidentes. L'approche DataCentric offre un moyen plus complet et plus flexible de créer des systèmes d'intelligence artificielle qui peuvent s'adapter à l'évolution des données et des environnements. En se concentrant sur la qualité et l'analyse des données, DataCentric AI peut fournir des résultats plus précis et plus fiables, ce qui en fait un outil essentiel pour relever les défis de l'avenir.
DataCentric Inteligencia Artificial para Aplicaciones Multidisciplinarias: A Paradigm Shift in AI Development En el panorama tecnológico actual y en rápida evolución, el campo de la inteligencia artificial (IA) está experimentando una transformación significativa. enfoque tradicional basado en modelos, donde los modelos de IA se construyen sobre la base de algoritmos y reglas preestablecidos, está siendo desafiado por un nuevo paradigma: el enfoque DataCentric. Este cambio de perspectiva pone de relieve la importancia de los datos de alta calidad y su papel en el desarrollo e implementación de sistemas de IA. libro «DataCentric Artificial Intelligence for Multidisciplinary Applications» explora la necesidad de un enfoque de DataCentric AI y sus aplicaciones en diferentes campos, destacando los beneficios y el potencial de este cambio de paradigma. La necesidad de un enfoque centrado en los datos enfoque tradicional de la IA centrado en los modelos aborda con éxito problemas específicos en áreas claramente definidas. n embargo, a medida que las aplicaciones de IA se expanden a campos más complejos e interdisciplinarios, las limitaciones de este enfoque son cada vez más evidentes. enfoque DataCentric ofrece una forma más completa y flexible de crear sistemas de inteligencia artificial que puedan adaptarse a datos y entornos cambiantes. Al centrarse en la calidad y el análisis de datos, DataCentric AI puede proporcionar resultados más precisos y confiables, lo que lo convierte en una herramienta importante para enfrentar los desafíos del futuro.
DataCentric Künstliche Intelligenz für multidisciplinäre Anwendungen: Paradigmenwechsel in der KI-Entwicklung In der heutigen schnelllebigen Technologielandschaft befindet sich der Bereich der Künstlichen Intelligenz (KI) im Umbruch. Der traditionelle modellorientierte Ansatz, bei dem KI-Modelle auf vordefinierten Algorithmen und Regeln basieren, wird durch ein neues Paradigma herausgefordert - den DataCentric-Ansatz. Dieser Perspektivwechsel unterstreicht die Bedeutung hochwertiger Daten und deren Rolle bei der Entwicklung und Implementierung von KI-Systemen. Das Buch „DataCentric Artificial Intelligence for Multidisciplinary Applications“ untersucht die Notwendigkeit des DataCentric AI-Ansatzes und seiner Anwendung in verschiedenen Bereichen und hebt die Vorteile und das Potenzial dieses Paradigmenwechsels hervor. Die Notwendigkeit eines datengetriebenen Ansatzes Der traditionelle modellgetriebene Ansatz der KI löst erfolgreich spezifische Probleme in klar definierten Bereichen. Da KI-Anwendungen jedoch in komplexere und interdisziplinärere Bereiche expandieren, werden die Grenzen dieses Ansatzes immer deutlicher. Der DataCentric-Ansatz bietet eine umfassendere und flexiblere Möglichkeit, KI-Systeme zu erstellen, die sich an sich ändernde Daten und Umgebungen anpassen können. Durch die Fokussierung auf Datenqualität und -analyse kann DataCentric AI genauere und zuverlässigere Ergebnisse liefern und ist damit ein wichtiges Werkzeug, um die Herausforderungen der Zukunft zu meistern.
''
DataCentric Çok Disiplinli Uygulamalar için Yapay Zeka: Yapay Zeka Gelişiminde Bir Paradigma Değişimi Günümüzün hızla gelişen teknolojik ortamında, yapay zeka (AI) alanı önemli bir dönüşüm geçiriyor. AI modellerinin önceden belirlenmiş algoritmalar ve kurallar temelinde inşa edildiği geleneksel model odaklı yaklaşım, yeni bir paradigma olan DataCentric yaklaşımı ile tartışılmaktadır. Perspektifteki bu değişim, yüksek kaliteli verilerin önemini ve AI sistemlerinin tasarımı ve uygulanmasındaki rolünü vurgulamaktadır. "DataCentric Artificial Intelligence for Multidisipliner Applications" (Çok Disiplinli Uygulamalar için DataCentric Yapay Zeka) kitabı, DataCentric AI yaklaşımına ve bunun birden fazla alanda uygulanmasına duyulan ihtiyacı araştırıyor ve bu paradigma değişiminin faydalarını ve potansiyelini vurguluyor. Veri merkezli bir yaklaşım ihtiyacı Yapay zekaya yönelik geleneksel model merkezli yaklaşım, iyi tanımlanmış alanlardaki belirli zorlukları başarıyla ele almaktadır. Bununla birlikte, AI uygulamaları daha karmaşık ve disiplinlerarası alanlara genişledikçe, bu yaklaşımın sınırlamaları daha belirgin hale gelmektedir. DataCentric yaklaşımı, değişen verilere ve ortamlara uyum sağlayabilen yapay zeka sistemleri oluşturmak için daha kapsamlı ve esnek bir yol sunar. DataCentric AI, veri kalitesi ve analizine odaklanarak daha doğru ve güvenilir sonuçlar sağlayabilir ve bu da onu geleceğin zorluklarını ele almak için önemli bir araç haline getirir.
الذكاء الاصطناعي المتمركز حول البيانات للتطبيقات متعددة التخصصات: تحول نموذجي في تطوير الذكاء الاصطناعي في المشهد التكنولوجي سريع التطور اليوم، يمر مجال الذكاء الاصطناعي (AI) بتحول كبير. النهج التقليدي الموجه نحو النموذج، حيث يتم بناء نماذج الذكاء الاصطناعي على أساس خوارزميات وقواعد محددة مسبقًا، يتنازع عليه نموذج جديد - نهج DataCentric. يسلط هذا التحول في المنظور الضوء على أهمية البيانات عالية الجودة ودورها في تصميم وتنفيذ أنظمة الذكاء الاصطناعي. يستكشف كتاب "DataCentric Artificial Intelligence for Multidisciples Applications'الحاجة إلى نهج DataCentric AI وتطبيقه عبر مجالات متعددة، مما يسلط الضوء على فوائد وإمكانات هذا التحول النموذجي. 27- يتصدى النهج التقليدي المتمحور حول النموذج إزاء الذكاء الاصطناعي بنجاح لتحديات محددة في مجالات محددة جيداً. ومع ذلك، مع توسع تطبيقات الذكاء الاصطناعي إلى مجالات أكثر تعقيدًا ومتعددة التخصصات، أصبحت قيود هذا النهج أكثر وضوحًا. يوفر نهج DataCentric طريقة أكثر شمولاً ومرونة لبناء أنظمة ذكاء اصطناعي يمكنها التكيف مع البيانات والبيئات المتغيرة. من خلال التركيز على جودة البيانات وتحليلها، يمكن أن يوفر الذكاء الاصطناعي DataCentric نتائج أكثر دقة وموثوقية، مما يجعله أداة مهمة لمواجهة تحديات المستقبل.
