
BOOKS - PROGRAMMING - Robust Machine Learning Distributed Methods for Safe AI

Robust Machine Learning Distributed Methods for Safe AI
Author: Rachid Guerraoui, Nirupam Gupta, Rafael Pinot
Year: 2024
Pages: 180
Format: PDF | EPUB
File size: 10.1 MB
Language: ENG
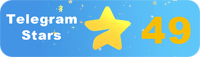
Year: 2024
Pages: 180
Format: PDF | EPUB
File size: 10.1 MB
Language: ENG
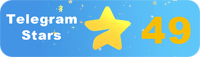
Book Description: Robust Machine Learning Distributed Methods for Safe AI Rachid Guerraoui, Nirupam Gupta, Rafael Pinot 2024 180 Summary: In today's world, machine learning algorithms are increasingly being used in various industries, from healthcare to finance, and their popularity continues to grow. However, as these algorithms become more widespread, the need for robustness and security becomes even more pressing. Robust Machine Learning Distributed Methods for Safe AI addresses this issue by providing a comprehensive guide to developing secure and reliable machine learning algorithms that can withstand threats such as hardware and software bugs, data poisoning, and malicious players. The book begins by introducing the concept of robustness in modern machine learning and its importance in ensuring the survival of humanity in the face of technological advancements. It then delves into the challenges of distributed machine learning, including the potential risks associated with distributing algorithms across multiple machines. The author explains how these risks can compromise the learning process and lead to undesirable outcomes, making it essential to understand the process of technology evolution and its impact on society.
Надежные распределенные методы машинного обучения для безопасного ИИ Рашид Геррауи, Нирупам Гупта, Рафаэль Пино 2024 180 Резюме: В современном мире алгоритмы машинного обучения все чаще используются в различных отраслях, от здравоохранения до финансов, и их популярность продолжает расти. Однако по мере распространения этих алгоритмов потребность в надежности и безопасности становится еще более насущной. Robust Machine arning Distributed Methods for Safe AI решает эту проблему, предоставляя исчерпывающее руководство по разработке безопасных и надежных алгоритмов машинного обучения, способных противостоять таким угрозам, как аппаратные и программные ошибки, отравление данных и вредоносные проигрыватели. Книга начинается с введения концепции устойчивости в современном машинном обучении и её важности в обеспечении выживания человечества перед лицом технологических достижений. Затем он углубляется в проблемы распределенного машинного обучения, включая потенциальные риски, связанные с распределением алгоритмов по нескольким машинам. Автор объясняет, как эти риски могут поставить под угрозу процесс обучения и привести к нежелательным результатам, что делает необходимым понимание процесса эволюции технологий и его влияния на общество.
Robustos métodos distribuidos de aprendizaje automático para una IA segura Rashid Gerraoui, Nirupam Gupta, Rafael Pino 2024 180 Resumen: En el mundo actual, los algoritmos de aprendizaje automático se utilizan cada vez más en diversas industrias, desde la salud hasta las finanzas, y su popularidad sigue creciendo. n embargo, a medida que estos algoritmos se extienden, la necesidad de confiabilidad y seguridad se vuelve aún más urgente. Robust Machine arning Distributed Methods for Safe AI resuelve este problema proporcionando una guía exhaustiva para desarrollar algoritmos de aprendizaje automático seguros y confiables capaces de enfrentar amenazas como errores de hardware y software, envenenamiento de datos y reproductores maliciosos. libro comienza introduciendo el concepto de sostenibilidad en el aprendizaje automático moderno y su importancia para garantizar la supervivencia de la humanidad frente a los avances tecnológicos. Luego se profundiza en los problemas del aprendizaje automático distribuido, incluyendo los riesgos potenciales asociados con la distribución de algoritmos a través de varias máquinas. autor explica cómo estos riesgos pueden comprometer el proceso de aprendizaje y conducir a resultados no deseados, lo que hace necesario comprender el proceso de evolución de la tecnología y su impacto en la sociedad.
''
Secure AI Rashid Gerraoui、 Nirupam Gupta、 Rafael Pino 2024 180の堅牢な分散型機械学習方法の概要:今日の世界では、機械学習アルゴリズムは医療から金融まで幅広い分野で使用されており、その人気は高まっています。しかし、これらのアルゴリズムが増大するにつれて、信頼性とセキュリティの必要性はさらに急務になります。安全なAIのための強力な機械学習分散法は、ハードウェアやソフトウェアのバグ、データ中毒、悪意のあるプレーヤーなどの脅威に耐える安全で信頼性の高い機械学習アルゴリズムを開発するための包括的なガイドを提供することによって、この問題を解決します。この本は、現代の機械学習における持続可能性の概念の導入と、技術の進歩に直面して人類の生存を確保する上での重要性から始まります。次に、複数のマシンにアルゴリズムを配布することに関連する潜在的なリスクを含む、分散型機械学習の問題を掘り下げます。これらのリスクがどのように学習プロセスを危険にさらし、望ましくない結果につながるのかを説明しています。
