
BOOKS - PROGRAMMING - Practicing Trustworthy Machine Learning

Practicing Trustworthy Machine Learning
Author: Yada Pruksachatkun, Matthew McAteer and Subhabrata Majumdar
Year: 2022-04-12 First Release
Format: EPUB
File size: 10 MB
Language: ENG
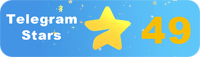
Year: 2022-04-12 First Release
Format: EPUB
File size: 10 MB
Language: ENG
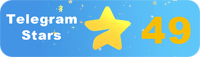
''
