
BOOKS - Interpretability in Deep Learning

Interpretability in Deep Learning
Author: Ayush Somani
Year: April 30, 2023
Format: PDF
File size: PDF 13 MB
Language: English
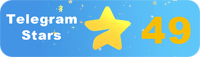
Year: April 30, 2023
Format: PDF
File size: PDF 13 MB
Language: English
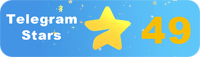
Book Interpretability in Deep Learning Introduction: In today's fast-paced world of technological advancements, it is crucial to understand the intricacies of deep learning models and their interpretability. With the rapid evolution of technology, it is essential to study and comprehend the process of developing modern knowledge to ensure the survival of humanity and unity among people. This book provides a comprehensive overview of recent research tools for interpreting deep learning models, focusing on neural network architectures. It also features case studies from various application-oriented articles in the fields of computer vision, optics, and machine learning. As a monograph on interpretability in deep learning, this book covers the latest developments and future prospects in the field, making it an indispensable resource for scientists, researchers, and graduate students alike. Chapter 1: The Importance of Interpretability in Deep Learning The need for interpretability in deep learning arises from the complexity of these models, which can be challenging to understand and debug. As the models become more sophisticated, it becomes increasingly important to comprehend their inner workings to avoid errors and improve performance. This chapter discusses the significance of interpretability in deep learning and its impact on the development of modern knowledge.
Интерпретируемость книги в глубоком обучении Введение: В современном быстро развивающемся мире технологических достижений крайне важно понимать тонкости моделей глубокого обучения и их интерпретируемость. С быстрым развитием технологий необходимо изучать и осмысливать процесс развития современных знаний для обеспечения выживания человечества и единства среди людей. В этой книге представлен всесторонний обзор инструментов последних исследований для интерпретации моделей глубокого обучения с акцентом на архитектуры нейронных сетей. В нем также представлены тематические исследования из различных прикладных статей в области компьютерного зрения, оптики и машинного обучения. Как монография о интерпретируемости в глубоком обучении, эта книга охватывает последние разработки и будущие перспективы в этой области, что делает ее незаменимым ресурсом как для ученых, исследователей, так и для аспирантов. Глава 1: Важность интерпретируемости в глубоком обучении Необходимость интерпретируемости в глубоком обучении возникает из-за сложности этих моделей, которые могут быть сложными для понимания и отладки. По мере того, как модели становятся все более сложными, становится все более важным понимать их внутреннюю работу, чтобы избежать ошибок и улучшить производительность. В этой главе обсуждается значение интерпретируемости в глубоком обучении и ее влияние на развитие современных знаний.
Interprétation d'un livre en apprentissage profond Introduction : Dans le monde actuel en évolution rapide des progrès technologiques, il est essentiel de comprendre les subtilités des modèles d'apprentissage profond et leur interprétabilité. Avec le développement rapide de la technologie, il est nécessaire d'étudier et de réfléchir au processus de développement des connaissances modernes pour assurer la survie de l'humanité et l'unité entre les hommes. Ce livre présente un aperçu complet des outils de recherche les plus récents pour interpréter les modèles d'apprentissage profond en mettant l'accent sur les architectures de réseaux neuronaux. Il présente également des études de cas provenant de divers articles appliqués dans les domaines de la vision par ordinateur, de l'optique et de l'apprentissage automatique. En tant que monographie sur l'interprétabilité en apprentissage profond, ce livre couvre les développements récents et les perspectives d'avenir dans ce domaine, ce qui en fait une ressource indispensable pour les scientifiques, les chercheurs et les étudiants des cycles supérieures. Chapitre 1 : L'importance de l'interprétabilité dans l'apprentissage profond La nécessité de l'interprétabilité dans l'apprentissage profond découle de la complexité de ces modèles, qui peuvent être difficiles à comprendre et à déboguer. À mesure que les modèles deviennent de plus en plus complexes, il devient de plus en plus important de comprendre leur fonctionnement interne pour éviter les erreurs et améliorer les performances. Ce chapitre traite de l'importance de l'interprétabilité dans l'apprentissage profond et de son impact sur le développement des connaissances modernes.
La interpretabilidad del libro en el aprendizaje profundo Introducción: En el mundo actual, en rápida evolución, de los avances tecnológicos es fundamental comprender las sutilezas de los modelos de aprendizaje profundo y su interpretabilidad. Con el rápido desarrollo de la tecnología, es necesario estudiar y reflexionar sobre el proceso de desarrollo del conocimiento moderno para garantizar la supervivencia de la humanidad y la unidad entre los seres humanos. Este libro ofrece una visión general completa de las herramientas de la investigación más reciente para interpretar modelos de aprendizaje profundo con énfasis en la arquitectura de redes neuronales. También presenta estudios de casos de diversos artículos aplicados en el campo de la visión por ordenador, la óptica y el aprendizaje automático. Como monografía sobre la interpretabilidad en el aprendizaje profundo, este libro abarca los últimos desarrollos y perspectivas de futuro en este campo, convirtiéndolo en un recurso indispensable tanto para científicos, investigadores y estudiantes de posgrado. Capítulo 1: La importancia de la interpretabilidad en el aprendizaje profundo La necesidad de la interpretabilidad en el aprendizaje profundo surge de la complejidad de estos modelos, que pueden ser complejos de entender y depurar. A medida que los modelos se vuelven cada vez más complejos, es cada vez más importante comprender su funcionamiento interno para evitar errores y mejorar el rendimiento. Este capítulo discute la importancia de la interpretabilidad en el aprendizaje profundo y su impacto en el desarrollo del conocimiento moderno.
Interpretabilidade do livro no aprendizado profundo Introdução: Em um mundo em desenvolvimento moderno, os avanços tecnológicos são essenciais para compreender as sutilezas dos modelos de aprendizado profundo e sua interpretação. Com o rápido desenvolvimento da tecnologia, é preciso estudar e refletir o processo de desenvolvimento do conhecimento moderno para garantir a sobrevivência da humanidade e a unidade entre os seres humanos. Este livro apresenta uma revisão completa das ferramentas de pesquisa mais recentes para interpretar modelos de aprendizagem profunda com foco na arquitetura de redes neurais. Ele também apresenta estudos de caso de diversos artigos de aplicação sobre visão de computador, ótica e aprendizado de máquinas. Como uma monografia sobre interpretação na aprendizagem profunda, este livro abrange os últimos desenvolvimentos e perspectivas futuras nesta área, tornando-o um recurso indispensável para cientistas, pesquisadores e pós-graduados. Capítulo 1: A importância da interpretação no aprendizado profundo A necessidade de interpretação no aprendizado profundo decorre da complexidade destes modelos, que podem ser complexos para a compreensão e depuração. À medida que os modelos se tornam cada vez mais complexos, é cada vez mais importante compreender o seu funcionamento interno para evitar erros e melhorar a produtividade. Este capítulo discute o significado da interpretação no aprendizado profundo e sua influência no desenvolvimento do conhecimento moderno.
Interpretabilità del libro nell'apprendimento approfondito Introduzione: In un mondo in continua evoluzione, il progresso tecnologico è fondamentale per comprendere le finezze dei modelli di apprendimento profondo e la loro interpretabilità. Con il rapido sviluppo della tecnologia, è necessario studiare e comprendere il processo di sviluppo delle conoscenze moderne per garantire la sopravvivenza dell'umanità e l'unità tra gli uomini. Questo libro fornisce una panoramica completa degli strumenti di ricerca più recenti per interpretare modelli di apprendimento approfondito con un focus sull'architettura delle reti neurali. Contiene anche studi di caso provenienti da diversi articoli applicativi in materia di visione informatica, ottica e apprendimento automatico. Come monografia sull'interpretabilità nell'apprendimento profondo, questo libro copre gli ultimi sviluppi e le prospettive future in questo campo, rendendolo una risorsa indispensabile sia per scienziati, ricercatori che per studenti di laurea. Capitolo 1: L'importanza dell'interpretazione nell'apprendimento approfondito La necessità di interpretazione nell'apprendimento approfondito deriva dalla complessità di questi modelli, che possono essere complessi da comprendere e debugger. Mentre i modelli diventano sempre più complessi, diventa sempre più importante comprendere il loro funzionamento interno per evitare errori e migliorare le prestazioni. In questo capitolo si discute il significato dell'interpretazione nell'apprendimento profondo e il suo impatto sullo sviluppo delle conoscenze moderne.
Interpretierbarkeit des Buches im Deep arning Einleitung: In der heutigen schnelllebigen Welt des technologischen Fortschritts ist es entscheidend, die Feinheiten von Deep-arning-Modellen und ihre Interpretierbarkeit zu verstehen. Mit der rasanten Entwicklung der Technologie ist es notwendig, den Prozess der Entwicklung des modernen Wissens zu studieren und zu verstehen, um das Überleben der Menschheit und die Einheit unter den Menschen zu gewährleisten. Dieses Buch bietet einen umfassenden Überblick über die Werkzeuge der neuesten Forschung zur Interpretation von Deep-arning-Modellen mit Schwerpunkt auf neuronalen Netzwerkarchitekturen. Es präsentiert auch Fallstudien aus verschiedenen angewandten Artikeln in den Bereichen Computer Vision, Optik und maschinelles rnen. Als Monographie über die Interpretierbarkeit im Deep arning behandelt dieses Buch die neuesten Entwicklungen und Zukunftsperspektiven in diesem Bereich und ist damit eine unverzichtbare Ressource für Wissenschaftler, Forscher und Doktoranden gleichermaßen. Kapitel 1: Die Bedeutung der Interpretierbarkeit im Deep arning Die Notwendigkeit der Interpretierbarkeit im Deep arning ergibt sich aus der Komplexität dieser Modelle, die schwer zu verstehen und zu debuggen sein können. Da Modelle immer komplexer werden, wird es immer wichtiger, ihr Innenleben zu verstehen, um Fehler zu vermeiden und die istung zu verbessern. Dieses Kapitel diskutiert die Bedeutung der Interpretierbarkeit im Deep arning und ihre Auswirkungen auf die Entwicklung des modernen Wissens.
Book Interprettability in Deep arning Introduction: בעולם המתפתח במהירות של התקדמות טכנולוגית, חיוני להבין את המורכבות של מודלים של למידה עמוקה ואת הפרשנות שלהם. עם ההתפתחות המהירה של הטכנולוגיה, יש צורך ללמוד ולהבין את התפתחות הידע המודרני כדי להבטיח את הישרדות האנושות והאחדות בקרב בני האדם. ספר זה מספק סקירה מקיפה של כלי מחקר עדכניים לפירוש מודלים ללמידה עמוקה תוך התמקדות בארכיטקטורות רשת עצבית. הוא כולל גם מחקרי מקרה ממגוון עבודות שימושיות בראייה ממוחשבת, אופטיקה ולימוד מכונה. כמונוגרפיה על יכולת פרשנות בלמידה מעמיקה, הספר סוקר את ההתפתחויות האחרונות ואת סיכויי העתיד בתחום, מה שהופך אותו למשאב הכרחי עבור מדענים, חוקרים ותלמידי תואר שני כאחד. פרק 1: חשיבותה של יכולת הפרשנות בלמידה עמוקה הצורך בפרשנות בלמידה עמוקה נובע ממורכבותם של מודלים אלה, אשר יכולים להיות קשים להבנה ולהדיפה. ככל שמודלים נעשים מורכבים יותר, נעשה חשוב יותר להבין את פעולתם הפנימית כדי להימנע מטעויות ולשפר את הביצועים. פרק זה דן במשמעות הפרשנות של הלמידה העמוקה ובהשפעתה על התפתחות הידע המודרני.''
Derin Öğrenmede Kitap Yorumlanabilirliği Giriş: Günümüzün hızla gelişen teknolojik gelişmeler dünyasında, derin öğrenme modellerinin inceliklerini ve yorumlanabilirliklerini anlamak zorunludur. Teknolojinin hızla gelişmesiyle birlikte, insanlığın hayatta kalmasını ve insanlar arasında birliği sağlamak için modern bilginin gelişimini incelemek ve kavramak gerekir. Bu kitap, sinir ağı mimarilerine odaklanarak derin öğrenme modellerini yorumlamak için son araştırma araçlarına kapsamlı bir genel bakış sunmaktadır. Ayrıca, bilgisayar görüşü, optik ve makine öğrenimi alanlarında çeşitli uygulamalı makalelerden vaka çalışmaları içermektedir. Derin öğrenmede yorumlanabilirlik üzerine bir monografi olarak, bu kitap alandaki son gelişmeleri ve gelecekteki beklentileri kapsamakta ve onu bilim adamları, araştırmacılar ve lisansüstü öğrenciler için vazgeçilmez bir kaynak haline getirmektedir. Bölüm 1: Derin Öğrenmede Yorumlanabilirliğin Önemi Derin öğrenmede yorumlanabilirlik ihtiyacı, anlaşılması ve hata ayıklanması zor olabilen bu modellerin karmaşıklığından kaynaklanmaktadır. Modeller daha karmaşık hale geldikçe, hataları önlemek ve performansı artırmak için iç çalışmalarını anlamak daha önemli hale gelir. Bu bölümde derin öğrenmede yorumlanabilirliğin önemi ve modern bilginin gelişimi üzerindeki etkisi tartışılmaktadır.
تفسير الكتاب في مقدمة التعلم العميق: في عالم اليوم سريع التطور للتقدم التكنولوجي، من الضروري فهم تعقيدات نماذج التعلم العميق وإمكانية تفسيرها. مع التطور السريع للتكنولوجيا، من الضروري دراسة وفهم تطور المعرفة الحديثة لضمان بقاء البشرية والوحدة بين الناس. يقدم هذا الكتاب لمحة عامة شاملة عن أدوات البحث الحديثة لتفسير نماذج التعلم العميق مع التركيز على بنى الشبكات العصبية. كما يعرض دراسات حالة من أوراق تطبيقية مختلفة في الرؤية الحاسوبية والبصريات والتعلم الآلي. كدراسة حول قابلية التفسير في التعلم العميق، يغطي هذا الكتاب التطورات الأخيرة والآفاق المستقبلية في هذا المجال، مما يجعله مصدرًا لا غنى عنه للعلماء والباحثين وطلاب الدراسات العليا على حد سواء. الفصل 1: أهمية التفسير في التعلم العميق تنشأ الحاجة إلى إمكانية التفسير في التعلم العميق من تعقيد هذه النماذج، التي قد يصعب فهمها وتصحيحها. عندما تصبح النماذج أكثر تعقيدًا، يصبح من المهم فهم أساليب عملها الداخلية لتجنب الأخطاء وتحسين الأداء. يناقش هذا الفصل أهمية القابلية للتفسير في التعلم العميق وأثرها على تطور المعرفة الحديثة.
딥 러닝 소개에서 책 해석: 오늘날 빠르게 진화하는 기술 발전 세계에서 딥 러닝 모델의 복잡성과 해석 성을 이해하는 것이 필수적입니다. 기술의 빠른 발전으로 사람들 간의 인류와 연합의 생존을 보장하기 위해 현대 지식의 발전을 연구하고 이해해야합니다. 이 책은 신경망 아키텍처에 중점을 둔 딥 러닝 모델을 해석하기위한 최신 연구 도구에 대한 포괄적 인 개요를 제공합니다. 또한 컴퓨터 비전, 광학 및 기계 학습에 관한 다양한 응용 논문의 사례 연구도 제공합니다. 딥 러닝의 해석 가능성에 관한 논문으로서이 책은 최근 해당 분야의 발전과 미래 전망을 다루며 과학자, 연구원 및 대학원생 모두에게 없어서는 안될 자료입니다. 1 장: 딥 러닝의 통역 가능성의 중요성 딥 러닝의 해석 가능성은 이러한 모델의 복잡성에서 비롯되며 이해하기 어려울 수 있습니다. 모델이 더욱 복잡 해짐에 따라 실수를 피하고 성능을 향상시키기 위해 내부 작업을 이해하는 것이 더 중요해집니다. 이 장에서는 딥 러닝에서의 해석 가능성의 중요성과 현대 지식의 발전에 미치는 영향에 대해 설명합니다.
深入學習書的可解釋性介紹:在當今快速發展的技術進步世界中,了解深度學習模型的復雜性及其可解釋性至關重要。隨著技術的迅速發展,必須研究和思考現代知識的發展,以確保人類的生存和人類的團結。本書全面概述了最新研究工具,以解釋深度學習模型,重點是神經網絡架構。它還提供了來自計算機視覺,光學和機器學習領域各種應用文章的案例研究。作為一本關於深度學習中的解釋性的專著,本書涵蓋了該領域的最新發展和未來前景,使其成為學者,研究人員和研究生不可或缺的資源。第1章:在深度學習中解釋的重要性深度學習中解釋的必要性源於這些模型的復雜性,這些模型可能難以理解和調試。隨著模型變得越來越復雜,了解其內部操作以避免錯誤並提高性能變得越來越重要。本章討論了深度學習中解釋性的意義及其對現代知識發展的影響。
