
BOOKS - Ultimate Salesforce Data Cloud for Customer Experience: Explore, Implement, a...

Ultimate Salesforce Data Cloud for Customer Experience: Explore, Implement, and Elevate B2C Experiences Through Customer Data Innovations Using Salesforce Data Cloud (English Edition)
Author: Gourab Mukherjee
Year: January 16, 2024
Format: PDF
File size: PDF 11 MB
Language: English
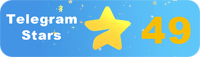
Year: January 16, 2024
Format: PDF
File size: PDF 11 MB
Language: English
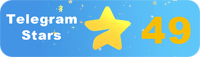
As we continue to explore the world of data science, it's important to understand the different types of data that exist and how they can be used to gain insights into various phenomena. In this article, we will delve into the concept of time series data and learn about its characteristics, applications, and challenges. Time series data refers to the sequence of data points observed at regular intervals over a period of time. This type of data is commonly found in fields such as finance, economics, weather forecasting, and healthcare. Time series data can be used to analyze trends, patterns, and anomalies in the data, which can provide valuable insights into the behavior of a system or process over time. Characteristics of Time Series Data: 1. Temporal Dependence: Time series data exhibits temporal dependence, meaning that the value of a data point at one time is influenced by the values of previous data points. 2. Autocorrelation: Time series data often exhibit autocorrelation, which means that the value of a data point at one time is correlated with the values of previous data points. 3.
По мере того, как мы продолжаем исследовать мир науки о данных, важно понимать различные типы существующих данных и то, как их можно использовать для понимания различных явлений. В этой статье мы углубимся в понятие данных временных рядов и узнаем о его характеристиках, приложениях и проблемах. Данные временных рядов относятся к последовательности точек данных, наблюдаемых с регулярными интервалами в течение определенного периода времени. Этот тип данных обычно встречается в таких областях, как финансы, экономика, прогнозирование погоды и здравоохранение. Данные временных рядов могут использоваться для анализа тенденций, закономерностей и аномалий в данных, что может дать ценную информацию о поведении системы или процесса во времени. Характеристики данных временных рядов: 1. Временная зависимость: Данные временных рядов демонстрируют временную зависимость, что означает, что на значение точки данных в один момент времени влияют значения предыдущих точек данных. 2. Автокорреляция: данные временных рядов часто демонстрируют автокорреляцию, что означает, что значение точки данных за один раз коррелирует со значениями предыдущих точек данных. 3.
Alors que nous continuons à explorer le monde de la science des données, il est important de comprendre les différents types de données existantes et comment elles peuvent être utilisées pour comprendre différents phénomènes. Dans cet article, nous allons approfondir la notion de données de séries chronologiques et en apprendre davantage sur ses caractéristiques, ses applications et ses problèmes. s données de séries chronologiques se rapportent à une succession de points de données observés à intervalles réguliers sur une période de temps déterminée. Ce type de données se trouve généralement dans des domaines tels que la finance, l'économie, les prévisions météorologiques et les soins de santé. s données des séries chronologiques peuvent être utilisées pour analyser les tendances, les schémas et les anomalies des données, ce qui peut fournir des informations précieuses sur le comportement du système ou du processus dans le temps. Caractéristiques des séries chronologiques : 1. Dépendance temporelle : s données des séries chronologiques montrent une dépendance temporelle, ce qui signifie que la valeur d'un point de données est influencée à un moment donné par les valeurs des points de données précédents. 2. Autocorrélation : les données de séries chronologiques démontrent souvent l'autocorrélation, ce qui signifie que la valeur d'un point de données est corrélée à la fois avec les valeurs des points de données précédents. 3.
A medida que continuamos explorando el mundo de la ciencia de los datos, es importante comprender los diferentes tipos de datos existentes y cómo pueden usarse para entender los diferentes fenómenos. En este artículo profundizaremos en la noción de serie temporal de datos y aprenderemos sobre sus características, aplicaciones y problemas. datos de series de tiempo se refieren a una secuencia de puntos de datos observados a intervalos regulares durante un período de tiempo determinado. Este tipo de datos se encuentran generalmente en áreas como finanzas, economía, predicción meteorológica y salud. datos de series temporales se pueden utilizar para analizar tendencias, patrones y anomalías en los datos, lo que puede proporcionar información valiosa sobre el comportamiento de un sistema o proceso en el tiempo. Características de los datos de series temporales: 1. Dependencia de tiempo: datos de series de tiempo muestran una dependencia de tiempo, lo que significa que el valor del punto de datos en un punto de tiempo se ve afectado por los valores de los puntos de datos anteriores. 2. Autocorrelación: los datos de series temporales a menudo muestran autocorrelación, lo que significa que el valor del punto de datos se correlaciona a la vez con los valores de los puntos de datos anteriores. 3.
À medida que continuamos a explorar o mundo da ciência dos dados, é importante compreender os diferentes tipos de dados existentes e como eles podem ser usados para compreender diferentes fenômenos. Neste artigo, vamos nos aprofundar no conceito de dados das séries de tempo e aprender sobre suas características, aplicações e problemas. Os dados das séries temporais referem-se a uma seqüência de pontos de dados observados em intervalos regulares por um determinado período de tempo. Este tipo de dados é geralmente encontrado em áreas como finanças, economia, previsões meteorológicas e saúde. Estes dados podem ser usados para analisar tendências, padrões e anomalias nos dados, o que pode fornecer informações valiosas sobre o comportamento do sistema ou do processo no tempo. As características das séries de tempo são 1. Dependência do tempo: os dados das séries de tempo mostram dependência temporária, o que significa que o valor do ponto de dados é influenciado por pontos de dados anteriores em um momento. 2. Correção automática: os dados das séries de tempo costumam mostrar a correção automática, o que significa que o valor do ponto de dados é correlacionado de uma vez para outra com os valores dos pontos de dados anteriores. 3.
Mentre continuiamo a esplorare il mondo della scienza dei dati, è importante comprendere i diversi tipi di dati esistenti e come possono essere utilizzati per comprendere i vari fenomeni. In questo articolo approfondiremo il concetto dei dati delle serie temporali e scopriremo le sue caratteristiche, le sue applicazioni e i suoi problemi. I dati delle serie temporali si riferiscono a una sequenza di punti dati osservati a intervalli regolari per un determinato periodo di tempo. Questo tipo di dati si trova solitamente in settori quali finanza, economia, previsioni meteo e sanità. I dati delle serie temporali possono essere utilizzati per analizzare tendenze, schemi e anomalie dei dati, fornendo informazioni preziose sul comportamento del sistema o del processo nel tempo. Caratteristiche dei dati delle serie temporali: 1. Dipendenza temporanea: i dati delle serie temporali mostrano una dipendenza temporale, il che significa che il valore del punto dati è influenzato dai valori dei punti dati precedenti in un unico momento. 2. Correzione automatica: i dati delle serie temporali spesso mostrano una correttura automatica, il che significa che il valore del punto dati è correlato alla volta ai valori dei punti dati precedenti. 3.
Während wir die Welt der Datenwissenschaft weiter erforschen, ist es wichtig, die verschiedenen Arten vorhandener Daten zu verstehen und wie sie zum Verständnis verschiedener Phänomene verwendet werden können. In diesem Artikel werden wir tiefer in das Konzept von Zeitreihendaten eintauchen und uns über seine Eigenschaften, Anwendungen und Probleme informieren. Zeitreihendaten beziehen sich auf eine Abfolge von Datenpunkten, die in regelmäßigen Abständen über einen bestimmten Zeitraum beobachtet werden. Diese Art von Daten wird häufig in Bereichen wie Finanzen, Wirtschaft, Wettervorhersage und Gesundheitswesen gefunden. Zeitreihendaten können verwendet werden, um Trends, Muster und Anomalien in den Daten zu analysieren, die wertvolle Informationen über das Verhalten eines Systems oder Prozesses im Laufe der Zeit liefern können. Merkmale der Zeitreihendaten: 1. Zeitabhängigkeit: Zeitreihendaten zeigen eine Zeitabhängigkeit, was bedeutet, dass der Wert eines Datenpunkts zu einem Zeitpunkt durch die Werte früherer Datenpunkte beeinflusst wird. 2. Autokorrelation: Zeitreihendaten weisen häufig eine Autokorrelation auf, was bedeutet, dass der Wert eines Datenpunkts gleichzeitig mit den Werten früherer Datenpunkte korreliert. 3.
Ponieważ nadal badamy świat danych naukowych, ważne jest, aby zrozumieć różne rodzaje istniejących danych i jak można je wykorzystać do zrozumienia różnych zjawisk. W tym artykule zagłębiamy się w koncepcję danych z serii czasowych i poznajemy jej cechy, aplikacje i problemy. Dane z szeregów czasowych odnoszą się do sekwencji punktów danych obserwowanych w regularnych odstępach czasu. Tego typu dane są powszechnie spotykane w takich dziedzinach jak finanse, ekonomia, prognozy pogody i opieka zdrowotna. Dane z serii czasowych mogą być wykorzystywane do analizy trendów, wzorców i anomalii w danych, które mogą dostarczyć cennych informacji o zachowaniu systemu lub procesu w czasie. Charakterystyka tych serii czasowych: 1. Zależność czasu: Dane szeregów czasowych pokazują zależność czasu, co oznacza, że wartość punktu danych w jednym czasie jest uzależniona od wartości poprzednich punktów danych. 2. Autokorrelacja: Dane z serii czasowych często pokazują autokorrelację, co oznacza, że wartość punktu danych w jednym czasie koreluje z wartościami poprzednich punktów danych. 3.
בעודנו ממשיכים לחקור את עולם מדעי הנתונים, חשוב להבין את סוגי הנתונים הקיימים השונים במאמר זה, אנו מתעמקים במושג של נתונים מסדרות זמן ולומדים על המאפיינים, היישומים והבעיות שלו. נתוני סדרת זמן מתייחסים לרצף של נקודות נתונים שנצפו במרווחים קבועים לאורך פרק זמן. נתונים מסוג זה נפוצים בתחומים כגון פיננסים, כלכלה, תחזית מזג אוויר ושירותי בריאות. ניתן להשתמש במידע מסדרת הזמן כדי לנתח מגמות, תבניות וחריגות במידע, אשר יכולות לספק מידע בעל ערך על התנהגות של מערכת או תהליך לאורך זמן. מאפיינים של סדרות זמן אלה: 1. תלות בזמן: נתוני סדרת זמן מציגים תלות בזמן, מה שאומר שהערך של נקודת מידע פעם אחת מושפע מהערכים של נקודות נתונים קודמות. 2. Autocorlation: מידע מסדרת טיים מראה לעתים קרובות שינוי אוטוקורלציה, מה שאומר שהערך של נקודת מידע בזמן מסוים תואם לערכים של נקודות נתונים קודמות. 3.''
Veri bilimi dünyasını keşfetmeye devam ederken, farklı veri türlerini ve farklı fenomenleri anlamak için nasıl kullanılabileceğini anlamak önemlidir. Bu yazıda, zaman serisi verileri kavramını inceliyoruz ve özellikleri, uygulamaları ve sorunları hakkında bilgi ediniyoruz. Zaman serisi verileri, belirli bir süre boyunca düzenli aralıklarla gözlemlenen bir dizi veri noktasını ifade eder. Bu tür veriler genellikle finans, ekonomi, hava tahmini ve sağlık hizmetleri gibi alanlarda bulunur. Zaman serisi verileri, bir sistemin veya sürecin zaman içindeki davranışı hakkında değerli bilgiler sağlayabilen verilerdeki eğilimleri, kalıpları ve anormallikleri analiz etmek için kullanılabilir. Bu zaman serilerinin özellikleri: 1. Zaman bağımlılığı: Zaman serisi verileri zaman bağımlılığını gösterir; bu, bir kerede bir veri noktasının değerinin önceki veri noktalarının değerlerinden etkilendiği anlamına gelir. 2. Otokorelasyon: Zaman serisi verileri genellikle otokorelasyonu gösterir, bu da bir veri noktasının bir kerede değerinin önceki veri noktalarının değerleriyle ilişkili olduğu anlamına gelir. 3.
بينما نواصل استكشاف عالم علم البيانات، من المهم فهم الأنواع المختلفة للبيانات الموجودة وكيف يمكن استخدامها لفهم الظواهر المختلفة. في هذه المقالة، نتعمق في مفهوم بيانات السلاسل الزمنية ونتعرف على خصائصها وتطبيقاتها ومشاكلها. تشير بيانات السلاسل الزمنية إلى سلسلة من نقاط البيانات التي لوحظت على فترات منتظمة على مدى فترة من الزمن. يوجد هذا النوع من البيانات بشكل شائع في مجالات مثل التمويل والاقتصاد والتنبؤ بالطقس والرعاية الصحية. يمكن استخدام بيانات السلاسل الزمنية لتحليل الاتجاهات والأنماط والشذوذ في البيانات، والتي يمكن أن توفر معلومات قيمة حول سلوك النظام أو العملية بمرور الوقت. خصائص هذه السلاسل الزمنية: 1. الاعتماد على الوقت: تظهر بيانات السلسلة الزمنية الاعتماد على الوقت، مما يعني أن قيمة نقطة البيانات في وقت واحد تتأثر بقيم نقاط البيانات السابقة. 2. الارتباط التلقائي: غالبًا ما تُظهر بيانات السلسلة الزمنية الارتباط التلقائي، مما يعني أن قيمة نقطة البيانات في وقت واحد ترتبط بقيم نقاط البيانات السابقة. 3.
데이터 과학의 세계를 계속 탐색함에 따라 다양한 유형의 기존 데이터와 다른 현상을 이해하는 데 사용할 수있는 방법을 이해하는 것이 중요합니다. 이 기사에서 우리는 시계열 데이터의 개념을 탐구하고 그 특성, 응용 프로그램 및 문제에 대해 배웁니다. 시계열 데이터는 일정 기간 동안 일정한 간격으로 관찰되는 일련의 데이터 포인트를 나타냅니다. 이러한 유형의 데이터는 일반적으로 금융, 경제, 일기 예보 및 건강 관리와 같은 분야에서 발견됩니다. 시계열 데이터는 데이터의 추세, 패턴 및 이상을 분석하는 데 사용될 수 있으며 시스템의 동작 또는 시간이 지남에 따라 프로세스에 대한 유용한 정보를 제공 할 수 있습니다. 이 시계열의 특징: 1. 시간 종속성: 시계열 데이터는 시간 종속성을 보여줍니다. 즉, 한 번에 데이터 포인트의 값은 이전 데이터 포인트의 값에 영향을받습니다. 2. 자기 상관: 시계열 데이터는 종종 자기 상관을 보여줍니다. 이는 한 번에 데이터 포인트의 값이 이전 데이터 포인트의 값과 관련이 있음을 의미합니다. 3.
私たちはデータサイエンスの世界を探求し続けているので、既存のデータの種類と、それらを使用してさまざまな現象を理解する方法を理解することが重要です。この記事では、時系列データの概念を掘り下げ、その特性、用途、問題について学びます。時系列データとは、一定期間にわたって一定の間隔で観測される一連のデータポイントを指します。このタイプのデータは、金融、経済、天気予報、医療などの分野で一般的に見られます。時系列データを使用して、データの傾向、パターン、および異常を分析することができます。これらの時系列の特徴:1。時間依存性:時系列データは時間依存性を示します。つまり、データポイントの値は以前のデータポイントの値の影響を受けます。2.自己相関:時系列データはしばしば自己相関を示します。つまり、データポイントの値は以前のデータポイントの値と一度に相関します。3.
當我們繼續探索數據科學的世界時,了解不同類型的現有數據以及如何利用它們來理解不同的現象很重要。本文將深入研究時間序列數據的概念,了解其特征、應用和問題。時間序列數據是指在特定時間段內定期觀察到的數據點序列。這種類型的數據通常出現在金融,經濟,天氣預報和醫療保健等領域。時間序列數據可用於分析數據中的趨勢,模式和異常,這可以提供有關系統或過程隨時間變化的行為的有價值的信息。時間序列數據特征:1。時間依賴性:時間序列數據顯示時間依賴性,這意味著某個時間點的數據點值受先前數據點的值影響。2.自相關:時間序列數據通常顯示自相關,這意味著數據點值一次與先前數據點的值相關。3.
