
BOOKS - Machine Learning for Physics and Astronomy

Machine Learning for Physics and Astronomy
Author: Viviana Acquaviva
Year: 2023
Pages: 281
Format: PDF
File size: 61.0 MB
Language: ENG
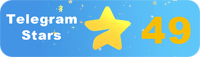
Year: 2023
Pages: 281
Format: PDF
File size: 61.0 MB
Language: ENG
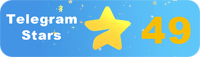
The book "Machine Learning for Physics and Astronomy" is a comprehensive guide to understanding the role of machine learning in the field of physics and astronomy. The author, a renowned expert in the field, provides a detailed overview of the current state of machine learning research and its applications in these fields, highlighting the challenges and opportunities that come with this rapidly evolving technology. The book covers topics such as supervised and unsupervised learning, deep learning, neural networks, and reinforcement learning, providing readers with a solid foundation in the principles and practices of machine learning. It also explores the various applications of machine learning in physics and astronomy, including image processing, data analysis, and modeling complex systems. The author emphasizes the need to study and understand the process of technological evolution, particularly in the context of machine learning, as it has the potential to revolutionize our understanding of the universe and our place within it. They argue that developing a personal paradigm for perceiving the technological process of developing modern knowledge is essential for survival in a warring world. By embracing this perspective, we can better appreciate the significance of machine learning in shaping our future and the importance of staying informed about its development.
Книга «Машинное обучение для физики и астрономии» является всеобъемлющим руководством по пониманию роли машинного обучения в области физики и астрономии. Автор, известный эксперт в этой области, дает подробный обзор текущего состояния исследований в области машинного обучения и их применения в этих областях, подчеркивая проблемы и возможности, которые возникают в связи с этой быстро развивающейся технологией. Книга охватывает такие темы, как контролируемое и неконтролируемое обучение, глубокое обучение, нейронные сети и обучение с подкреплением, предоставляя читателям прочную основу в принципах и практиках машинного обучения. Он также исследует различные приложения машинного обучения в физике и астрономии, включая обработку изображений, анализ данных и моделирование сложных систем. Автор подчеркивает необходимость изучения и понимания процесса технологической эволюции, особенно в контексте машинного обучения, поскольку он может революционизировать наше понимание Вселенной и нашего места в ней. Они утверждают, что разработка личной парадигмы восприятия технологического процесса развития современных знаний необходима для выживания в воюющем мире. Принимая эту точку зрения, мы можем лучше оценить значение машинного обучения в формировании нашего будущего и важность того, чтобы оставаться в курсе его развития.
''
