
BOOKS - PROGRAMMING - Machine Learning-Based Modelling in Atomic Layer Deposition Pro...

Machine Learning-Based Modelling in Atomic Layer Deposition Processes
Author: Oluwatobi Adeleke, Tien-Chien Jen, Sina Karimzadeh
Year: 2024
Pages: 377
Format: PDF
File size: 40.2 MB
Language: ENG
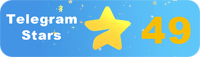
Year: 2024
Pages: 377
Format: PDF
File size: 40.2 MB
Language: ENG
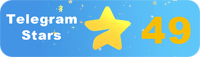
''
