
BOOKS - PROGRAMMING - Deep Learning Applications In Computer Vision, Signals And Netw...

Deep Learning Applications In Computer Vision, Signals And Networks
Author: Qi Xuan, Yun Xiang, Dongwei Xu
Year: 2023
Pages: 309
Format: PDF
File size: 40.2 MB
Language: ENG
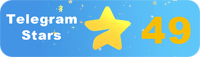
Year: 2023
Pages: 309
Format: PDF
File size: 40.2 MB
Language: ENG
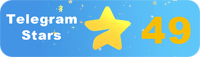
''
