
BOOKS - Data Engineering for Machine Learning Pipelines From Python Libraries to ML P...

Data Engineering for Machine Learning Pipelines From Python Libraries to ML Pipelines and Cloud Platforms
Author: Pavan Kumar Narayanan
Year: 2024
Pages: 631
Format: PDF
File size: 33.0 MB
Language: ENG
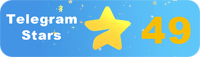
Year: 2024
Pages: 631
Format: PDF
File size: 33.0 MB
Language: ENG
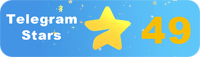
Book Description: The book "Data Engineering for Machine Learning Pipelines From Python Libraries to ML Pipelines and Cloud Platforms" provides a comprehensive overview of the field of data engineering, from the basics of Python libraries to the latest advancements in cloud platforms. The book covers the entire spectrum of data engineering, from data ingestion and storage to data processing and analysis, and finally to machine learning pipelines. It offers practical guidance on how to build scalable and reliable data pipelines using Python libraries such as NumPy, pandas, and scikit-learn, as well as popular cloud platforms like AWS, GCP, and Azure. The book also explores the challenges of working with large datasets and the importance of data quality, data governance, and data security. The book begins by introducing the concept of data engineering and its role in the broader field of artificial intelligence (AI) and machine learning (ML). It explains how data engineering has evolved over time, from simple data storage solutions to complex data pipelines that power modern AI/ML applications. The authors highlight the need for a personal paradigm for perceiving the technological process of developing modern knowledge, emphasizing the importance of understanding the evolution of technology and its impact on society. They argue that this understanding is crucial for survival in today's rapidly changing world. The book then delves into the details of data ingestion, explaining how data can be extracted from various sources, such as databases, APIs, and files.
В книге «Data Engineering for Machine arning Pipelines From Python Libraries to ML Pipelines and Cloud Platforms» представлен всесторонний обзор области инженерии данных, от основ библиотек Python до последних достижений в области облачных платформ. Книга охватывает весь спектр инженерии данных, от приема и хранения данных до обработки и анализа данных и, наконец, до конвейеров машинного обучения. Он предлагает практическое руководство по созданию масштабируемых и надежных конвейеров данных с использованием библиотек Python, таких как NumPy, pandas и scikit-learn, а также популярных облачных платформ, таких как AWS, GCP и Azure. В книге также рассматриваются проблемы работы с большими наборами данных и важность качества данных, управления данными и безопасности данных. Книга начинается с введения концепции инженерии данных и её роли в более широкой области искусственного интеллекта (ИИ) и машинного обучения (ML). Он объясняет, как инженерия данных развивалась с течением времени, от простых решений для хранения данных до сложных конвейеров данных, которые обеспечивают работу современных приложений AI/ML. Авторы подчеркивают необходимость личностной парадигмы восприятия технологического процесса развития современных знаний, подчеркивая важность понимания эволюции технологий и ее влияния на общество. Они утверждают, что это понимание имеет решающее значение для выживания в современном быстро меняющемся мире. Затем книга углубляется в детали приема данных, объясняя, как данные могут быть извлечены из различных источников, таких как базы данных, API и файлы.
Il libro Data Engineering for Machine arning Pipelines From Python Libraries to ML Pipelines and Cloud Platforms fornisce una panoramica completa dell'ingegneria dei dati, dai fondamentali delle librerie Python agli ultimi progressi nelle piattaforme cloud. Il libro comprende tutta la gamma dell'ingegneria dei dati, dall'acquisizione e conservazione ai dati, fino all'elaborazione e all'analisi dei dati, fino alla catena di montaggio dell'apprendimento automatico. Offre un manuale pratico per la creazione di sistemi di spedizione scalabili e affidabili con librerie Python, come NumPy, pandas e scikit-learn, e piattaforme cloud popolari come AWS, GCP e Azure. Il libro affronta anche le problematiche relative ai dataset di grandi dimensioni e l'importanza della qualità dei dati, della gestione dei dati e della sicurezza dei dati. Il libro inizia introducendo il concetto di ingegneria dei dati e il suo ruolo nel campo più ampio dell'intelligenza artificiale (IA) e dell'apprendimento automatico (ML). Spiega come l'ingegneria dei dati si sia evoluta nel corso del tempo, dalle semplici soluzioni di storage alle complesse reti di montaggio dei dati che garantiscono il funzionamento delle attuali applicazioni AI/ML. Gli autori sottolineano la necessità di un paradigma personale della percezione del processo tecnologico dello sviluppo della conoscenza moderna, sottolineando l'importanza di comprendere l'evoluzione della tecnologia e il suo impatto sulla società. Sostengono che questa comprensione sia fondamentale per la sopravvivenza in un mondo in rapido cambiamento. Il libro viene quindi approfondito nelle parti di ricezione dei dati, spiegando come i dati possono essere recuperati da diverse origini, quali database, API e file.
''
