
BOOKS - Deep Learning with PyTorch, Second Edition (MEAP v5)

Deep Learning with PyTorch, Second Edition (MEAP v5)
Author: Luca Antiga, Eli Stevens, Howard Huang
Year: 2024
Pages: 326
Format: PDF | EPUB
File size: 27.0 MB
Language: ENG
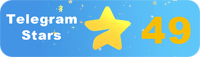
Year: 2024
Pages: 326
Format: PDF | EPUB
File size: 27.0 MB
Language: ENG
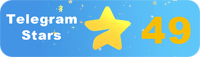
The book provides a step-by-step approach to building and training deep learning models, from the basics of tensor operations to advanced techniques such as transfer learning and domain adaptation. The book also covers the latest advancements in deep learning research, including attention mechanisms, transformers, and generative models. With this book, readers will gain a solid understanding of the principles of deep learning and the skills to implement them in real-world applications. The book is divided into four parts: Part I: Fundamentals of Deep Learning, Part II: Building and Training Deep Learning Models, Part III: Applications of Deep Learning, and Part IV: Advanced Topics in Deep Learning. Each part builds upon the previous one, providing a comprehensive overview of the field of deep learning and its applications. The book is written in an accessible and easy-to-understand style, making it suitable for both beginners and experienced practitioners who want to learn about deep learning and its applications.
В книге представлен пошаговый подход к построению и обучению моделей глубокого обучения, от основ тензорных операций до передовых техник, таких как трансферное обучение и адаптация доменов. Книга также охватывает последние достижения в области исследований глубокого обучения, включая механизмы внимания, трансформаторы и генеративные модели. С помощью этой книги читатели получат твердое понимание принципов глубокого обучения и навыки их реализации в реальных приложениях. Книга разделена на четыре части: Часть I: Основы глубокого обучения, Часть II: Построение и обучение моделей глубокого обучения, Часть III: Применение глубокого обучения и Часть IV: Продвинутые темы в глубоком обучении. Каждая часть основывается на предыдущей, предоставляя всесторонний обзор области глубокого обучения и его приложений. Книга написана в доступном и простом для понимания стиле, что делает ее подходящей как для начинающих, так и для опытных практиков, которые хотят узнать о глубоком обучении и его приложениях.
''
