
BOOKS - PROGRAMMING - Operationalizing Machine Learning Pipelines

Operationalizing Machine Learning Pipelines
Author: Vishwajyoti Pandey, Shaleen Bengani
Year: 2022
Pages: 203
Format: EPUB
File size: 10 MB
Language: ENG
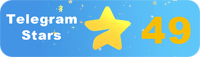
Year: 2022
Pages: 203
Format: EPUB
File size: 10 MB
Language: ENG
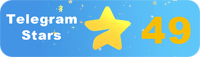
sets. The goal of this book is to show readers how to create a productionalized ML workflow that is scalable, reliable, secure, and easy to maintain. The book provides guidance on how to implement MLOps at scale, build robust data pipelines, manage model training and deployment, and monitor and optimize model performance. It also covers security, compliance, and governance aspects of MLOps. Each chapter provides practical examples and exercises to help readers apply their knowledge and skills to real-world scenarios. The book begins by exploring the need for operationalizing machine learning (ML) pipelines in large organizations and the challenges associated with scaling up ML models in production environments. It then delves into the foundational concepts of MLOps, including version control, continuous integration and delivery, containerization, and cloud computing. The next section discusses the tools and techniques used in MLOps, such as TensorFlow, PyTorch, AWS SageMaker, Azure Machine Learning, and GitHub Actions. The book concludes with a discussion on the future of MLOps, including emerging trends and technologies like explainable AI, transfer learning, and federated learning. The book's focus is on providing practical guidance for data scientists, engineers, and IT professionals who want to operationalize ML workflows in their organizations.
sets. Цель этой книги - показать читателям, как создать производственный рабочий процесс ML, который является масштабируемым, надежным, безопасным и простым в обслуживании. Книга содержит руководство о том, как реализовать MLOps в масштабе, построить надежные конвейеры данных, управлять обучением и развертыванием моделей, а также контролировать и оптимизировать производительность моделей. Он также охватывает аспекты безопасности, соответствия и управления MLOps.В каждой главе приводятся практические примеры и упражнения, которые помогут читателям применить свои знания и навыки к реальным сценариям. Книга начинается с изучения необходимости операционализации конвейеров машинного обучения (ML) в крупных организациях и проблем, связанных с масштабированием ML-моделей в производственных средах. Затем он углубляется в основополагающие концепции MLOps, включая контроль версий, непрерывную интеграцию и доставку, контейнеризацию и облачные вычисления. В следующем разделе обсуждаются инструменты и методы, используемые в MLOps, такие как TensorFlow, PyTorch, AWS SageMaker, Azure Machine arning и GitHub Actions.Книга завершается обсуждением будущего MLOps, включая новые тенденции и технологии, такие как объяснимый ИИ, трансфертное обучение и федеративное обучение. Основное внимание в книге уделяется практическому руководству для специалистов по данным, инженеров и ИТ-специалистов, которые хотят внедрить рабочие процессы ML в своих организациях.
sets. but de ce livre est de montrer aux lecteurs comment créer un flux de production ML qui est évolutif, fiable, sûr et facile à entretenir. livre fournit un guide sur la façon de mettre en œuvre MLOps à l'échelle, de construire des pipelines de données fiables, de gérer la formation et le déploiement des modèles, et de surveiller et d'optimiser les performances des modèles. Chaque chapitre présente des exemples pratiques et des exercices qui aideront les lecteurs à appliquer leurs connaissances et leurs compétences à des scénarios réels. livre commence par étudier la nécessité d'exploiter les convoyeurs d'apprentissage automatique (ML) dans les grandes organisations et les problèmes liés à l'échelle des modèles ML dans les environnements de production. Il se penche ensuite sur les concepts fondamentaux de MLOps, y compris le contrôle de version, l'intégration et la livraison continues, la conteneurisation et le cloud computing. La section suivante traite des outils et des méthodes utilisés dans les MLOps, tels que TensorFlow, PyTorch, AWS SageMaker, Azure Machine arning et GitHub Actions. livre se termine par une discussion sur l'avenir des MLOps, y compris les nouvelles tendances et technologies telles que l'IA explicable, la formation de transfert et la formation fédérale. livre se concentre sur un guide pratique pour les professionnels des données, les ingénieurs et les professionnels de l'informatique qui veulent mettre en œuvre les flux de travail ML dans leurs organisations.
sets. objetivo de este libro es mostrar a los lectores cómo crear un flujo de trabajo de producción de ML que sea escalable, confiable, seguro y fácil de mantener. libro proporciona una guía sobre cómo implementar MLOps a escala, construir transportadores de datos confiables, administrar el aprendizaje y la implementación de modelos, y monitorear y optimizar el rendimiento de los modelos. También cubre aspectos de seguridad, cumplimiento y gestión de MLOps.Cada capítulo proporciona ejemplos prácticos y ejercicios que ayudarán a los lectores a aplicar sus conocimientos y habilidades a escenarios reales. libro comienza explorando la necesidad de operacionalizar los transportadores de aprendizaje automático (ML) en grandes organizaciones y los problemas relacionados con la escala de modelos ML en entornos de producción. A continuación, profundiza en los conceptos fundamentales de MLOps, incluyendo el control de versiones, la integración y entrega continuas, la containerización y la computación en la nube. En la siguiente sección se analizan las herramientas y métodos utilizados en MLOps, como TensorFlow, PyTorch, AWS SageMaker, Azure Machine arning y GitHub Actions Ops, incluyendo nuevas tendencias y tecnologías como la IA explicable, el aprendizaje de transferencia y el aprendizaje federado. libro se centra en una guía práctica para profesionales de datos, ingenieros y profesionales de TI que desean implementar flujos de trabajo de ML en sus organizaciones.
sets. O objetivo deste livro é mostrar aos leitores como criar o fluxo de trabalho de produção ML, que é escalável, confiável, seguro e fácil de manter. O livro contém um guia sobre como implementar MLOs em escala, construir linhas de montagem confiáveis, gerenciar treinamento e implantação de modelos e monitorar e otimizar o desempenho dos modelos. Ele também abrange os aspectos de segurança, conformidade e gerenciamento do MLOps.Cada capítulo fornece exemplos práticos e exercícios que ajudam os leitores a aplicar seus conhecimentos e habilidades aos cenários reais. O livro começa por estudar a necessidade de operacionalizar as linhas de montagem de aprendizagem de máquinas (ML) em grandes organizações e os desafios de escalar modelos ML em ambientes de produção. Depois, aprofundou-se nos conceitos fundamentais do MLOps, incluindo controle de versões, integração e entrega contínuas, contêineres e computação em nuvem. A próxima seção aborda ferramentas e métodos usados no MLOps, tais como TensorFlow, PyTorch, AWS SageMaker, Azure Machine arning e GitHub Actions.O livro termina com discussões sobre o futuro do MLOps, incluindo novas tendências e tecnologias, tais como IA explicável, treinamento de transferência e formação federal. O livro é focado em orientações práticas para especialistas em dados, engenheiros e profissionais de TI que desejam implementar os processos de trabalho da ML em suas organizações.
sets. Lo scopo di questo libro è quello di mostrare ai lettori come creare un flusso di lavoro di produzione ML scalabile, affidabile, sicuro e di facile manutenzione. Il libro fornisce una guida su come implementare MLOs in scala, costruire reti di montaggio affidabili, gestire la formazione e l'implementazione dei modelli e monitorare e ottimizzare le prestazioni dei modelli. Include anche gli aspetti relativi alla sicurezza, alla conformità e alla gestione di MLOps.In ogni capitolo vengono forniti esempi pratici ed esercizi che aiutano i lettori ad applicare le proprie conoscenze e competenze agli scenari reali. Il libro inizia con l'analisi della necessità di operare le reti di montaggio per l'apprendimento automatico (ML) in aziende di grandi dimensioni e dei problemi legati alla scalabilità dei modelli ML negli ambienti di produzione. Viene quindi approfondito nei concetti fondamentali di MLOs, inclusi il controllo delle versioni, l'integrazione continua e la spedizione, il contenitore e il cloud computing. Nella sezione seguente vengono descritti gli strumenti e i metodi utilizzati in MLOs, come TensorFlow, PyTorch, AWS SageMaker, Azure Machine arning e GitHub Actions.Il libro si conclude con il dibattito sul futuro di MLOs, incluse nuove tendenze e tecnologie, come l'intelligenza artificiale spiegabile, l'apprendimento dei trasferimenti e l'apprendimento federale. Il libro si concentra sulla guida pratica per esperti di dati, ingegneri e professionisti IT che desiderano implementare i flussi di lavoro ML nelle loro organizzazioni.
sets. Das Ziel dieses Buches ist es, den sern zu zeigen, wie sie einen ML-Produktionsworkflow erstellen können, der skalierbar, zuverlässig, sicher und einfach zu warten ist. Das Buch enthält Anleitungen zur Implementierung von MLOps in großem Maßstab, zum Aufbau robuster Datenpipelines, zur Verwaltung von Modellschulungen und -bereitstellungen sowie zur Überwachung und Optimierung der Modellleistung. Es deckt auch die cherheits-, Compliance- und Managementaspekte von MLOs ab.Jedes Kapitel bietet praktische Beispiele und Übungen, die den sern helfen, ihr Wissen und ihre Fähigkeiten auf reale Szenarien anzuwenden. Das Buch beginnt mit der Untersuchung der Notwendigkeit der Operationalisierung von Machine arning (ML) -Pipelines in großen Organisationen und der Herausforderungen bei der Skalierung von ML-Modellen in Produktionsumgebungen. Anschließend werden die grundlegenden Konzepte von MLOps vertieft, darunter Versionskontrolle, kontinuierliche Integration und Lieferung, Containerisierung und Cloud Computing. Im folgenden Abschnitt werden die in MLOps verwendeten Tools und Methoden wie TensorFlow, PyTorch, AWS SageMaker, Azure Machine arning und GitHub Actions diskutiert. Das Buch schließt mit einer Diskussion über die Zukunft von MLOps, einschließlich neuer Trends und Technologien wie erklärbare KI, Transferlernen und föderales rnen. Der Schwerpunkt des Buches liegt auf einem praktischen itfaden für Datenspezialisten, Ingenieure und IT-Experten, die ML-Workflows in ihren Organisationen implementieren möchten.
zestawy |. Celem tej książki jest pokazanie czytelnikom, jak stworzyć przepływ pracy ML, który jest skalowalny, niezawodny, bezpieczny i łatwy w utrzymaniu. Książka zawiera wskazówki, jak wdrożyć MLOP na skalę, zbudować solidne rurociągi danych, zarządzać uczeniem się i wdrażaniem modeli oraz monitorować i optymalizować wydajność modelu. Obejmuje również aspekty bezpieczeństwa, zgodności i zarządzania MLOP. Każdy rozdział zawiera praktyczne przykłady i ćwiczenia, aby pomóc czytelnikom zastosować ich wiedzę i umiejętności do scenariuszy rzeczywistych. Książka rozpoczyna się od zbadania potrzeby operacjonalizacji rurociągów uczenia maszynowego (ML) w dużych organizacjach oraz wyzwań związanych ze skalowaniem modeli ML w środowiskach produkcyjnych. Następnie przenika do podstawowych koncepcji MLOp, w tym kontroli wersji, ciągłej integracji i dostawy, konteneryzacji i chmury obliczeniowej. W poniższej sekcji omówiono narzędzia i metody stosowane w MLOp, takie jak TensorFlow, PyTorch, AWS SageMaker, Azure Machine arning i GitHub Actions.Książka kończy się dyskusją na temat przyszłości MLOp, w tym pojawiających się trendów i technologii, takich jak: zrozumiała sztuczna inteligencja, uczenie się transferu i uczenie się sfederowane. Książka skupia się na praktycznych wskazówkach dla naukowców zajmujących się danymi, inżynierów i specjalistów z branży IT, którzy chcą wdrożyć przepływy pracy ML w swoich organizacjach.
סטים. מטרת הספר היא להראות לקוראים כיצד ליצור זרם עבודה של ייצור ML שניתן לאמוד, אמין, בטוח וקל לתחזוקה. הספר מספק הדרכה כיצד ליישם MLops בקנה מידה, לבנות צינורות נתונים חזקים, לנהל למידת מודל ופריסה, ולפקח ולייעל ביצועי מודל. הוא מכסה גם את היבטי האבטחה, הציות והניהול של MLOps. כל פרק מספק דוגמאות ותרגולים מעשיים שיעזרו לקוראים ליישם את הידע והמיומנויות שלהם בתרחישים של העולם האמיתי. הספר מתחיל בכך שהוא בוחן את הצורך בלמידת מכונות (ML) בארגונים גדולים ואת האתגרים הנלווים לסימון מודלי ML בסביבות הייצור. לאחר מכן הוא מתעמק במושגים הבסיסיים של MLOps, כולל בקרת גרסה, אינטגרציה רציפה והעברה, בלימה ומחשוב ענן. הסעיף הבא דן בכלים ובשיטות המשמשים ב-MLOps, כגון TensorFlow, PyTorch, AWS SageMaker, Azure Machine arning ו-GitHub Actions ולמידה פדרלית. הספר מתמקד בהדרכה מעשית למדעני נתונים, למהנדסים ולמומחי IT שרוצים ליישם את זרם העבודה של ML בארגוניהם.''
setleri. Bu kitabın amacı, okuyuculara ölçeklenebilir, güvenilir, güvenli ve bakımı kolay bir ML üretim iş akışının nasıl oluşturulacağını göstermektir. Kitap, MLOps'nin ölçekte nasıl uygulanacağı, sağlam veri boru hatları oluşturma, model öğrenme ve dağıtımını yönetme ve model performansını izleme ve optimize etme konusunda rehberlik sağlar. Ayrıca MLOps'ın güvenlik, uyumluluk ve yönetim yönlerini de kapsar. Her bölüm, okuyucuların bilgi ve becerilerini gerçek dünya senaryolarına uygulamalarına yardımcı olacak pratik örnekler ve alıştırmalar sunar. Kitap, büyük organizasyonlarda makine öğrenimi (ML) boru hatlarının operasyonel hale getirilmesi ihtiyacını ve üretim ortamlarında ML modellerinin ölçeklendirilmesiyle ilgili zorlukları inceleyerek başlıyor. Daha sonra, sürüm kontrolü, sürekli entegrasyon ve teslimat, konteynerleştirme ve bulut bilişim dahil olmak üzere MLOps'ın temel kavramlarını inceler. Aşağıdaki bölümde, TensorFlow, PyTorch, AWS SageMaker, Azure Machine arning ve GitHub Actions gibi MLOps'ta kullanılan araçlar ve yöntemler tartışılmaktadır. Kitap, açıklanabilir AI, aktarım gibi ortaya çıkan trendler ve teknolojiler de dahil olmak üzere MLOps'ın geleceği hakkında bir tartışma ile sona ermektedir Öğrenme ve federe öğrenme. Kitap, kuruluşlarında ML iş akışlarını uygulamak isteyen veri bilimcileri, mühendisler ve BT uzmanları için pratik rehberliğe odaklanmaktadır.
مجموعة |. الغرض من هذا الكتاب هو إظهار للقراء كيفية إنشاء سير عمل لإنتاج ML قابل للتطوير وموثوق به وآمن وسهل الصيانة. يقدم الكتاب إرشادات حول كيفية تنفيذ MLOps على نطاق واسع، وبناء خطوط أنابيب بيانات قوية، وإدارة تعلم النماذج ونشرها، ومراقبة أداء النموذج وتحسينه. كما يغطي جوانب الأمن والامتثال والإدارة في MLOps. يقدم كل فصل أمثلة عملية وتمارين لمساعدة القراء على تطبيق معارفهم ومهاراتهم على سيناريوهات العالم الحقيقي. يبدأ الكتاب بفحص الحاجة إلى تشغيل خطوط أنابيب التعلم الآلي (ML) في المؤسسات الكبيرة والتحديات المرتبطة بتوسيع نطاق نماذج ML في بيئات الإنتاج. ثم يتعمق في المفاهيم الأساسية لـ MLOps، بما في ذلك التحكم في الإصدار، والتكامل المستمر والتسليم، والحاويات، والحوسبة السحابية. يناقش القسم التالي الأدوات والطرق المستخدمة في MLOps، مثل TensorFlow و PyTorch و AWS SageMaker و Azure Machine arning و GitHub Actions مثل الذكاء الاصطناعي القابل للتفسير، ونقل التعلم، والتعلم الاتحادي. يركز الكتاب على الإرشادات العملية لعلماء البيانات والمهندسين ومتخصصي تكنولوجيا المعلومات الذين يرغبون في تنفيذ سير عمل ML في مؤسساتهم.
세트. 이 책의 목적은 독자들에게 확장 가능하고 안정적이며 안전하며 유지 관리하기 쉬운 ML 생산 워크 플로우를 만드는 방법을 보여주는 것입니다. 이 책은 대규모 MLop을 구현하고 강력한 데이터 파이프 라인을 구축하며 모델 학습 및 배포를 관리하며 모델 성능을 모니터링하고 최적화하는 방법에 대한 지침을 제공합니다. 또한 MLops의 보안, 규정 준수 및 관리 측면도 다룹니다. 각 장은 독자가 지식과 기술을 실제 시나리오에 적용 할 수 있도록 실용적인 예와 연습을 제공합니다. 이 책은 대규모 조직에서 기계 학습 (ML) 파이프 라인을 운영해야 할 필요성과 생산 환경에서 ML 모델 확장과 관련된 문제를 조사하는 것으로 시작됩니다. 그런 다음 버전 제어, 지속적인 통합 및 전달, 컨테이너화 및 클라우드 컴퓨팅을 포함한 MLops의 기본 개념을 탐구합니다. 다음 섹션에서는 TensorFlow, PyTorch, AWS SageMaker, Azure Machine arning 및 GitHub Action과 같은 MLops에 사용되는 도구 및 방법에 대해 설명합니다. AI, 전학 학습 및 연합 학습. 이 책은 조직에서 ML 워크 플로우를 구현하려는 데이터 과학자, 엔지니어 및 IT 전문가를위한 실제 지침에 중점을 둡니다.
セット。この本の目的は、スケーラブルで信頼性が高く、安全でメンテナンスが容易なML制作ワークフローを作成する方法を読者に示すことです。この本では、MLOpsを大規模に実装し、堅牢なデータパイプラインを構築し、モデル学習と展開を管理し、モデルパフォーマンスを監視および最適化する方法についてのガイダンスを提供します。また、MLOpsのセキュリティ、コンプライアンス、管理の側面もカバーしています。各章では、実際のシナリオに自分の知識とスキルを適用するための実践的な例と演習を提供します。本書は、大規模な組織における機械学習(ML)パイプラインの運用の必要性と、生産環境におけるMLモデルのスケーリングに関連する課題を検討することから始まります。その後、バージョン管理、継続的な統合と配信、コンテナ化、クラウドコンピューティングなど、MLOpsの基本的な概念を掘り下げます。次のセクションでは、TensorFlow、 PyTorch、 AWS SageMaker、 Azure Machine arning、 GitHub Actionsなど、MLOpsで使用されるツールとメソッドについて説明しますそのような説明可能なAI、転送学習、および連合学習などの新興トレンドと技術。本書では、組織にMLワークフローを実装したいデータサイエンティスト、エンジニア、ITプロフェッショナルのための実践的なガイダンスに焦点を当てています。
sets.本書的目的是向讀者展示如何創建可擴展、可靠、安全且易於維護的ML生產工作流。該書提供了有關如何大規模實施MLOps,構建可靠的數據流水線,管理模型的培訓和部署以及控制和優化模型性能的指南。它還涵蓋了MLOps.的安全性,合規性和管理方面。每章提供了實用的示例和練習,以幫助讀者將其知識和技能應用於現實世界中的場景。本書首先探討了大型組織中機器學習(ML)流水線的操作需求以及在生產環境中擴展ML模型的問題。然後,他深入研究了MLOps的基本概念,包括版本控制,持續集成和交付,集裝箱化和雲計算。下一節討論了MLOps中使用的工具和技術,例如TensorFlow,PyTorch,AWS SageMaker,Azure Machine arning和GitHub Actions。該書以討論MLO的未來而告終,包括新的趨勢。以及可解釋的AI,轉移學習和聯邦學習等技術。本書的重點是為希望在其組織中實施ML工作流的數據專業人員,工程師和IT專業人員提供實用指南。
