
BOOKS - Split Federated Learning for Secure IoT Applications Concepts, frameworks, ap...

Split Federated Learning for Secure IoT Applications Concepts, frameworks, applications and case studies
Author: Gururaj Harinahalli Lokesh, Geetabai S. Hukkeri, N.Z. Jhanjhi, Hong Lin
Year: 2024
Pages: 285
Format: PDF
File size: 10.1 MB
Language: ENG
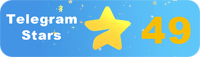
Year: 2024
Pages: 285
Format: PDF
File size: 10.1 MB
Language: ENG
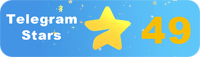
The book "Split Federated Learning for Secure IoT Applications: Concepts, Frameworks, and Case Studies" provides a comprehensive overview of the current state of federated learning research and its applications in secure IoT applications. The book covers the fundamental concepts of federated learning, including the challenges and limitations of traditional federated learning approaches, and introduces the concept of split federated learning as a potential solution to these challenges. It also discusses various frameworks and case studies that demonstrate the effectiveness of split federated learning in securing IoT applications. The book begins by exploring the need for secure IoT applications and the role that federated learning can play in achieving this goal. It highlights the importance of protecting sensitive data and ensuring the privacy and security of users in IoT applications, and how federated learning can help achieve this goal. The book then delves into the details of traditional federated learning approaches, including their limitations and challenges, and how split federated learning can address these issues. The book next presents the concept of split federated learning and its benefits, including improved data privacy and security, better communication efficiency, and enhanced fault tolerance. It explains how split federated learning works and how it can be applied to various IoT applications, such as smart homes, industrial control systems, and intelligent transportation systems. The book also discusses the challenges associated with implementing split federated learning in real-world scenarios and provides guidance on overcoming these challenges.
В книге «Split Federated arning for Secure IoT Applications: Concepts, Frameworks, and Case Studies» (Разделение федеративного обучения для безопасных приложений Интернета вещей: концепции, инфраструктуры и практические примеры) представлен всесторонний обзор текущего состояния исследований федеративного обучения и его приложений в безопасных приложениях Интернета вещей. Книга охватывает фундаментальные концепции федеративного обучения, включая проблемы и ограничения традиционных подходов к федеративному обучению, и представляет концепцию раздельного федеративного обучения как потенциальное решение этих проблем. Здесь также обсуждаются различные структуры и тематические исследования, демонстрирующие эффективность раздельного объединенного обучения в обеспечении безопасности приложений Интернета вещей. Книга начинается с изучения потребности в безопасных приложениях для Интернета вещей и роли, которую федеративное обучение может сыграть в достижении этой цели. В нем подчеркивается важность защиты конфиденциальных данных и обеспечения конфиденциальности и безопасности пользователей в приложениях Интернета вещей, а также то, как объединенное обучение может помочь в достижении этой цели. Затем в книге подробно рассматриваются традиционные подходы к федеративному обучению, включая их ограничения и проблемы, а также то, как разделенное федеративное обучение может решить эти проблемы. Далее в книге представлена концепция раздельного федеративного обучения и его преимущества, включая повышение конфиденциальности и безопасности данных, повышение эффективности связи и повышение отказоустойчивости. В нем объясняется, как работает разделенное федеративное обучение и как его можно применить к различным приложениям Интернета вещей, таким как умные дома, промышленные системы управления и интеллектуальные транспортные системы. В книге также обсуждаются проблемы, связанные с внедрением раздельного федеративного обучения в реальных сценариях, и приводятся рекомендации по преодолению этих проблем.
''
