
BOOKS - The Computational Content Analyst Using Machine Learning to Classify Media Me...

The Computational Content Analyst Using Machine Learning to Classify Media Messages
Author: Chris J. Vargo
Year: 2025
Pages: 144
Format: PDF | EPUB | MOBI
File size: 10.1 MB
Language: ENG
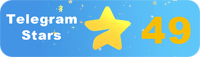
Year: 2025
Pages: 144
Format: PDF | EPUB | MOBI
File size: 10.1 MB
Language: ENG
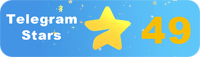
The Computational Content Analyst Using Machine Learning to Classify Media Messages In today's digital age, the amount of data available to us has grown exponentially, making it increasingly difficult to process and analyze manually. The advent of immense datasets requires a more productive approach to labeling media beyond a team of researchers. This book offers practical guidance and Python code to traverse the vast expanses of data, significantly enhancing productivity without compromising scholarly integrity. Understanding the Need for Technological Evolution The rapid evolution of technology has led to an explosion of digital content, with thousands of news articles and millions of social media posts being generated every day. This deluge of information has made it imperative to develop a personal paradigm for perceiving the technological process of developing modern knowledge. By studying and understanding the process of technological evolution, we can better appreciate the need for computational content analysis and its potential to classify media messages accurately and precisely. Computational Content Analysis: A Necessity in Today's World Computational content analysis is a powerful tool that enables researchers to analyze large datasets quickly and accurately. It involves using machine learning algorithms to classify media messages based on their content, allowing for a more efficient and effective approach to labeling media. This methodology is particularly useful for academic researchers looking to classify media data and advanced scholars in mass communications research, media studies, digital communication, political communication, and journalism. Selecting the Best Methodology The book provides a comprehensive overview of computer-based classification approaches, focusing on easy-to-understand methodological explanations and best practices to ensure accurate and precise labeling of media messages. Researchers will learn how to select the best computational content analysis methodology for their specific needs and problems.
The Computational Content Analyst Using Machine arning to Classification Media Messages В современную цифровую эпоху количество доступных нам данных росло в геометрической прогрессии, усложняя обработку и анализ вручную. Появление огромных наборов данных требует более продуктивного подхода к маркировке СМИ за пределами команды исследователей. Эта книга предлагает практическое руководство и код на Python для обхода огромных пространств данных, значительно повышая производительность без ущерба для научной целостности. Понимание необходимости технологической эволюции Стремительное развитие технологий привело к взрыву цифрового контента: ежедневно генерируются тысячи новостных статей и миллионы постов в социальных сетях. Этот поток информации сделал необходимым разработку личной парадигмы восприятия технологического процесса развития современных знаний. Изучая и понимая процесс технологической эволюции, мы можем лучше оценить потребность в вычислительном анализе контента и его потенциал для точной и точной классификации медиасообщений. Анализ вычислительного контента: необходимость в современном мире Анализ вычислительного контента - это мощный инструмент, который позволяет исследователям быстро и точно анализировать большие наборы данных. Он предполагает использование алгоритмов машинного обучения для классификации медиасообщений на основе их содержания, что позволяет более эффективно и действенно подходить к маркировке медиа. Эта методология особенно полезна для академических исследователей, желающих классифицировать данные СМИ, и передовых ученых в области исследований в области массовых коммуникаций, медиа-исследований, цифровых коммуникаций, политических коммуникаций и журналистики. Выбор наилучшей методологии В книге представлен всесторонний обзор компьютерных подходов к классификации с акцентом на простые для понимания методологические объяснения и лучшие практики для обеспечения точной и точной маркировки сообщений СМИ. Исследователи узнают, как выбрать лучшую методологию анализа вычислительного контента для своих конкретных потребностей и проблем.
The Computational Content Analyst Using Machine arning to Classification Media Messages À l'ère numérique moderne, le nombre de données disponibles a augmenté de façon exponentielle, compliquant le traitement et l'analyse manuelle. L'émergence de vastes ensembles de données nécessite une approche plus productive de l'étiquetage des médias au-delà de l'équipe de chercheurs. Ce livre offre un guide pratique et du code sur Python pour contourner les vastes espaces de données, augmentant considérablement la productivité sans compromettre l'intégrité scientifique. Comprendre la nécessité de l'évolution technologique développement rapide de la technologie a entraîné une explosion du contenu numérique : des milliers d'articles d'actualité et des millions de posts sur les réseaux sociaux sont générés chaque jour. Ce flux d'informations a rendu nécessaire l'élaboration d'un paradigme personnel de perception du processus technologique du développement des connaissances modernes. En étudiant et en comprenant le processus d'évolution technologique, nous pouvons mieux évaluer le besoin d'analyse informatique du contenu et son potentiel pour une classification précise et précise des messages médiatiques. Analyse de contenu informatique : une nécessité dans le monde d'aujourd'hui L'analyse de contenu informatique est un outil puissant qui permet aux chercheurs d'analyser rapidement et avec précision de grands ensembles de données. Il s'agit d'utiliser des algorithmes d'apprentissage automatique pour classer les messages multimédias en fonction de leur contenu, ce qui permet une approche plus efficace et efficiente du marquage des médias. Cette méthodologie est particulièrement utile pour les chercheurs universitaires désireux de classer les données des médias et les scientifiques de pointe dans les domaines de la recherche sur les communications de masse, la recherche sur les médias, la communication numérique, la communication politique et le journalisme. Sélection de la meilleure méthodologie livre présente un aperçu complet des approches informatiques de la classification, en mettant l'accent sur des explications méthodologiques faciles à comprendre et sur les meilleures pratiques pour assurer un étiquetage précis et précis des messages des médias. s chercheurs apprendront à choisir la meilleure méthodologie d'analyse de contenu informatique pour leurs besoins et défis spécifiques.
The Computacional Content Analyst Using Machine arning to Classification Media Messages En la era digital actual, la cantidad de datos disponibles para nosotros ha crecido exponencialmente, complicando el procesamiento y el análisis manuales. La aparición de enormes conjuntos de datos requiere un enfoque más productivo para etiquetar los medios fuera del equipo de investigadores. Este libro ofrece una guía práctica y código en Python para sortear enormes espacios de datos, mejorando significativamente el rendimiento sin comprometer la integridad científica. Entender la necesidad de la evolución tecnológica rápido desarrollo de la tecnología ha llevado a la explosión del contenido digital: miles de artículos de noticias y millones de publicaciones en redes sociales se generan diariamente. Este flujo de información ha hecho necesario el desarrollo del paradigma personal de la percepción del proceso tecnológico del desarrollo del conocimiento moderno. Mediante el estudio y la comprensión del proceso de evolución tecnológica, podemos evaluar mejor la necesidad de análisis computacional de los contenidos y su potencial para una clasificación precisa y precisa de los mensajes mediáticos. Análisis de contenido computacional: una necesidad en el mundo actual análisis de contenido computacional es una herramienta poderosa que permite a los investigadores analizar grandes conjuntos de datos de manera rápida y precisa. Implica el uso de algoritmos de aprendizaje automático para clasificar los mensajes de medios basados en su contenido, lo que permite un enfoque más eficiente y eficiente en el etiquetado de medios. Esta metodología es especialmente útil para los investigadores académicos que desean clasificar los datos de los medios de comunicación y para los académicos avanzados en investigación en comunicación de masas, investigación de medios, comunicación digital, comunicación política y periodismo. Selección de la mejor metodología libro ofrece una amplia visión general de los enfoques informáticos de la clasificación, con énfasis en explicaciones metodológicas fáciles de entender y mejores prácticas para garantizar el etiquetado preciso y preciso de los mensajes de los medios de comunicación. investigadores aprenderán a elegir la mejor metodología de análisis de contenido computacional para sus necesidades y problemas específicos.
The Computational Content Analyst Using Machine arning to Classificazione Media Messagges.Nell'era digitale attuale, i dati disponibili sono cresciuti in modo esponenziale, rendendo più difficile l'elaborazione e l'analisi manuale. La nascita di enormi set di dati richiede un approccio più produttivo all'etichettatura dei media al di fuori del team di ricercatori. Questo libro offre una guida pratica e un codice su Python per aggirare enormi spazi di dati, migliorando notevolmente le prestazioni senza compromettere l'integrità scientifica. La comprensione della necessità di un'evoluzione tecnologica Il rapido sviluppo della tecnologia ha fatto esplodere i contenuti digitali, generando ogni giorno migliaia di notizie e milioni di post sui social media. Questo flusso di informazioni ha reso necessario sviluppare un paradigma personale della percezione del processo tecnologico di sviluppo delle conoscenze moderne. Studiando e comprendendo l'evoluzione tecnologica, possiamo valutare meglio la necessità di un'analisi computazionale dei contenuti e il loro potenziale per una classificazione accurata e precisa dei messaggi multimediali. Analisi dei contenuti di elaborazione: necessità nel mondo moderno L'analisi dei contenuti di elaborazione è uno strumento potente che consente ai ricercatori di analizzare in modo rapido e preciso grandi set di dati. Prevede l'utilizzo di algoritmi di apprendimento automatico per classificare i messaggi multimediali basati sul loro contenuto, consentendo un approccio più efficiente e efficiente all'etichettatura dei media. Questa metodologia è particolarmente utile per i ricercatori accademici che desiderano classificare i dati dei media e per gli studiosi all'avanguardia nel campo delle comunicazioni di massa, della ricerca sui media, delle comunicazioni digitali, della comunicazione politica e del giornalismo. La scelta della metodologia migliore Il libro fornisce una panoramica completa degli approcci informatici per la classificazione, con un focus sulle spiegazioni metodologiche semplici da comprendere e le migliori pratiche per garantire un'etichettatura accurata e precisa dei messaggi dei media. I ricercatori scopriranno come scegliere la migliore metodologia di analisi dei contenuti computazionali per le proprie esigenze e problemi specifici.
The Computational Content Analyst Using Machine arning to Classification Media Messages Im heutigen digitalen Zeitalter ist die Menge der uns zur Verfügung stehenden Daten exponentiell gewachsen, was die manuelle Verarbeitung und Analyse erschwert. Die Entstehung riesiger Datensätze erfordert einen produktiveren Ansatz zur Kennzeichnung von Medien außerhalb des Forscherteams. Dieses Buch bietet einen praktischen itfaden und Python-Code, um riesige Datenräume zu umgehen und die istung erheblich zu verbessern, ohne die wissenschaftliche Integrität zu beeinträchtigen. Die Notwendigkeit der technologischen Entwicklung verstehen Die rasante Entwicklung der Technologie hat zu einer Explosion digitaler Inhalte geführt: Täglich werden Tausende von Nachrichtenartikeln und Millionen von Beiträgen in sozialen Medien generiert. Dieser Informationsfluss machte es notwendig, ein persönliches Paradigma für die Wahrnehmung des technologischen Prozesses der Entwicklung des modernen Wissens zu entwickeln. Durch das Studium und Verständnis des technologischen Evolutionsprozesses können wir den Bedarf an computergestützter Inhaltsanalyse und ihr Potenzial für eine genaue und genaue Klassifizierung von Medienmitteilungen besser einschätzen. Analyse von Computerinhalten: eine Notwendigkeit in der heutigen Welt Die Analyse von Computerinhalten ist ein leistungsfähiges Werkzeug, das es Forschern ermöglicht, große Datensätze schnell und genau zu analysieren. Es beinhaltet den Einsatz von maschinellen rnalgorithmen, um Medienmitteilungen basierend auf ihrem Inhalt zu klassifizieren, was einen effizienteren und effizienteren Ansatz für die Medienbeschriftung ermöglicht. Diese Methodik ist besonders nützlich für akademische Forscher, die Mediendaten klassifizieren möchten, und Spitzenwissenschaftler in den Bereichen Massenkommunikationsforschung, Medienforschung, digitale Kommunikation, politische Kommunikation und Journalismus. Auswahl der besten Methodik Das Buch bietet einen umfassenden Überblick über computergestützte Klassifizierungsansätze mit Schwerpunkt auf leicht verständlichen methodischen Erklärungen und Best Practices, um eine genaue und genaue Kennzeichnung von Medienberichten zu gewährleisten. Die Forscher lernen, wie sie die beste Methodik zur Analyse von Computerinhalten für ihre spezifischen Bedürfnisse und Herausforderungen auswählen.
Obliczeniowy analityk treści przy użyciu maszyny arning do klasyfikacji wiadomości medialnych W nowoczesnej erze cyfrowej ilość dostępnych nam danych wzrosła wykładniczo, komplikując ręczne przetwarzanie i analizę. Pojawienie się ogromnych zbiorów danych wymaga bardziej wydajnego podejścia do etykietowania mediów poza zespołem badawczym. Ta książka oferuje praktyczny przewodnik i kod Pythona do przemierzania rozległych przestrzeni danych, dramatycznie poprawiając wydajność bez naruszania integralności naukowej. Zrozumienie potrzeby ewolucji technologicznej Szybki rozwój technologii doprowadził do eksplozji treści cyfrowych: codziennie generowane są tysiące artykułów informacyjnych i miliony postów w mediach społecznościowych. Ten przepływ informacji spowodował konieczność opracowania osobistego paradygmatu postrzegania technologicznego procesu rozwoju nowoczesnej wiedzy. Badając i rozumiejąc proces ewolucji technologicznej, możemy lepiej ocenić potrzebę analizy treści obliczeniowych i jej potencjał do dokładnej i precyzyjnej klasyfikacji wiadomości medialnych. Analiza treści obliczeniowych: Potrzeba dzisiejszej światowej analizy treści obliczeniowych jest potężnym narzędziem umożliwiającym badaczom szybką i dokładną analizę dużych zbiorów danych. Polega ona na wykorzystaniu algorytmów uczenia maszynowego do klasyfikacji wiadomości medialnych w oparciu o ich zawartość, co pozwala na bardziej wydajne i skuteczne podejście do etykietowania mediów. Metodologia ta jest szczególnie przydatna dla naukowców akademickich, którzy chcą klasyfikować dane mediów i zaawansowanych naukowców w dziedzinie badań nad masową komunikacją, badań mediów, komunikacji cyfrowej, komunikacji politycznej i dziennikarstwa. Wybór najlepszej metodologii Książka zapewnia kompleksowy przegląd metod obliczeniowych do klasyfikacji, ze szczególnym uwzględnieniem łatwych do zrozumienia wyjaśnień metodologicznych i najlepszych praktyk w celu zapewnienia dokładnego i precyzyjnego etykietowania raportów medialnych. Naukowcy dowiedzą się, jak wybrać najlepszą metodologię analizy treści obliczeniowych dla swoich specyficznych potrzeb i wyzwań.
אנליסט התוכן החישובי תוך שימוש במכונה העוסקת בסיווג הודעות מדיה בעידן הדיגיטלי המודרני, כמות הנתונים הזמינים לנו גדלה באופן אקספוננציאלי, מסבכת עיבוד וניתוח ידני. הופעתן של מערכות נתונים ענקיות דורשת גישה פורה יותר לתוויות מדיה מחוץ לצוות המחקר. הספר הזה מציע מדריך ידני וקוד פייתון לחצות מרחבי נתונים עצומים, לשפר ביצועים באופן דרמטי מבלי להתפשר על שלמות מדעית. הבנת הצורך באבולוציה טכנולוגית ההתפתחות המהירה של הטכנולוגיה הובילה להתפוצצות של תוכן דיגיטלי: אלפי מאמרים חדשותיים ומיליוני פרסומים ברשתות החברתיות מופקים מדי יום. זרימה זו של מידע גרמה לכך שהיה צורך לפתח פרדיגמה אישית לתפיסה של התהליך הטכנולוגי של התפתחות הידע המודרני. על ידי לימוד והבנת תהליך האבולוציה הטכנולוגית, אנו יכולים להעריך טוב יותר את הצורך בניתוח תוכן חישובי ואת הפוטנציאל שלו לסיווג מדויק ומדויק של הודעות מדיה. ניתוח תוכן חישובי: הצורך בניתוח תוכן חישובי עולמי של ימינו הוא כלי רב עוצמה המאפשר לחוקרים לנתח מערכות מידע גדולות במהירות ובדייקנות. היא כרוכה בשימוש באלגוריתמים ללימוד מכונה כדי לסווג הודעות מדיה המבוססות על התוכן שלהם, המאפשר גישה יעילה ויעילה יותר לתוויות מדיה. מתודולוגיה זו שימושית במיוחד עבור חוקרים אקדמיים המבקשים לסווג נתוני מדיה וחוקרים מתקדמים בתחומי חקר תקשורת ההמונים, לימודי מדיה, תקשורת דיגיטלית, תקשורת פוליטית ועיתונאות. בחירת המתודולוגיה הטובה ביותר הספר מספק סקירה מקיפה של גישות חישוביות לסיווג, עם התמקדות בסברים מתודולוגיים קלים להבנה ושיטות טובות ביותר כדי להבטיח תוויות מדויקות ומדויקות של דיווחים בתקשורת. חוקרים ילמדו כיצד לבחור את מתודולוגיית ניתוח התוכן החישובי הטובה ביותר לצרכים והאתגרים הספציפיים שלהם.''
Hesaplamalı İçerik Analisti Sınıflandırma Medya Mesajlarına Makine Kullanımı Modern dijital çağda, elimizdeki veri miktarı katlanarak artmıştır, bu da manuel işleme ve analizi zorlaştırmaktadır. Büyük veri kümelerinin ortaya çıkması, araştırma ekibinin dışındaki medya etiketlemesine daha üretken bir yaklaşım gerektirir. Bu kitap, geniş veri alanlarını dolaşmak için uygulamalı bir rehber ve Python kodu sunar ve bilimsel bütünlükten ödün vermeden performansı önemli ölçüde artırır. Teknolojinin hızlı gelişimi dijital içeriğin patlamasına yol açtı: Her gün binlerce haber ve milyonlarca sosyal medya yayını üretiliyor. Bu bilgi akışı, modern bilginin gelişiminin teknolojik sürecinin algılanması için kişisel bir paradigma geliştirmeyi gerekli kılmıştır. Teknolojik evrim sürecini inceleyerek ve anlayarak, hesaplamalı içerik analizi ihtiyacını ve medya mesajlarının doğru ve kesin sınıflandırılması potansiyelini daha iyi değerlendirebiliriz. Hesaplamalı İçerik Analizi: Günümüz Dünyasının İhtiyacı Hesaplamalı içerik analizi, araştırmacıların büyük veri setlerini hızlı ve doğru bir şekilde analiz etmelerini sağlayan güçlü bir araçtır. Medya mesajlarını içeriklerine göre sınıflandırmak için makine öğrenme algoritmalarının kullanılmasını içerir, bu da medya etiketlemesine daha verimli ve etkili bir yaklaşım sağlar. Bu metodoloji, medya verilerini sınıflandırmak isteyen akademik araştırmacılar ve kitle iletişim araştırmaları, medya çalışmaları, dijital iletişim, politik iletişim ve gazetecilik alanlarında ileri düzey akademisyenler için özellikle yararlıdır. En iyi metodolojinin seçilmesi Kitap, medya raporlarının doğru ve kesin bir şekilde etiketlenmesini sağlamak için anlaşılması kolay metodolojik açıklamalara ve en iyi uygulamalara odaklanarak sınıflandırmaya yönelik hesaplamalı yaklaşımlara kapsamlı bir genel bakış sunar. Araştırmacılar, özel ihtiyaçları ve zorlukları için en iyi hesaplamalı içerik analizi metodolojisini nasıl seçeceklerini öğreneceklerdir.
محلل المحتوى الحاسوبي باستخدام التعلم الآلي لتصنيف رسائل الوسائط في العصر الرقمي الحديث، نمت كمية البيانات المتاحة لنا بشكل كبير، مما أدى إلى تعقيد المعالجة اليدوية والتحليل. يتطلب ظهور مجموعات بيانات ضخمة نهجًا أكثر إنتاجية لوضع العلامات على الوسائط خارج فريق البحث. يقدم هذا الكتاب دليلًا عمليًا وشفرة بايثون لاجتياز مساحات بيانات شاسعة، مما يحسن الأداء بشكل كبير دون المساس بالنزاهة العلمية. فهم الحاجة إلى التطور التكنولوجي أدى التطور السريع للتكنولوجيا إلى انفجار المحتوى الرقمي: يتم إنشاء آلاف المقالات الإخبارية وملايين المنشورات على وسائل التواصل الاجتماعي كل يوم. وهذا التدفق للمعلومات جعل من الضروري وضع نموذج شخصي لتصور العملية التكنولوجية لتطور المعرفة الحديثة. من خلال دراسة وفهم عملية التطور التكنولوجي، يمكننا تقييم الحاجة إلى تحليل المحتوى الحسابي وإمكاناته لتصنيف دقيق ودقيق لرسائل الوسائط. تحليل المحتوى الحاسوبي: تحليل المحتوى الحاسوبي العالمي اليوم هو أداة قوية تمكن الباحثين من تحليل مجموعات البيانات الكبيرة بسرعة ودقة. يتضمن استخدام خوارزميات التعلم الآلي لتصنيف رسائل الوسائط بناءً على محتواها، مما يسمح بنهج أكثر كفاءة وفعالية في تصنيف الوسائط. هذه المنهجية مفيدة بشكل خاص للباحثين الأكاديميين الراغبين في تصنيف بيانات وسائل الإعلام والباحثين المتقدمين في مجالات أبحاث الاتصال الجماهيري والدراسات الإعلامية والاتصالات الرقمية والاتصالات السياسية والصحافة. يقدم الكتاب لمحة عامة شاملة عن النهج الحسابية للتصنيف، مع التركيز على التفسيرات المنهجية السهلة الفهم وأفضل الممارسات لضمان توسيم التقارير الإعلامية بدقة ودقة. سيتعلم الباحثون كيفية اختيار أفضل منهجية لتحليل المحتوى الحسابي لاحتياجاتهم وتحدياتهم المحددة.
計算內容分析使用機器進行分類媒體信息在當今的數字時代,我們可用的數據數量呈指數增長,使手動處理和分析更加復雜。巨大的數據集的出現需要一種更加富有成效的方法來標記研究人員團隊之外的媒體。本書在Python上提供了實用指南和代碼,以繞過巨大的數據空間,從而在不損害科學完整性的情況下顯著提高了性能。了解技術發展的必要性技術的迅速發展導致了數字內容的爆發:每天有數千篇新聞文章和數百萬篇社交媒體文章。這種信息流動使得有必要發展個人範式,以感知現代知識發展的過程過程。通過研究和了解技術演化的過程,我們可以更好地評估對內容進行計算分析的需求及其對媒體消息進行準確和準確分類的潛力。計算內容分析:現代世界的需求計算內容分析是一個強大的工具,使研究人員能夠快速準確地分析大型數據集。它涉及使用機器學習算法根據其內容對媒體消息進行分類,從而可以更有效,更高效地進行媒體標記。這種方法對於希望對媒體數據進行分類的學術研究人員和大眾傳播,媒體研究,數字傳播,政治傳播和新聞學領域的高級學者特別有用。本書全面概述了計算機分類方法,著重於易於理解的方法論解釋和確保準確準確地標記媒體報道的最佳做法。研究人員將學習如何根據自己的特定需求和問題選擇更好的計算內容分析方法。
